Characterization of Moving Sound Sources Direction-of-Arrival Estimation Using Different Deep Learning Architectures.
IEEE Trans. Instrum. Meas.(2023)
摘要
Sound source localization is an important task for several applications and the use of deep learning for this task has recently become a popular research topic. While a number of previous works have focused on static sound sources, in this article, we evaluate the performance of a deep learning classification system for localization of moving sound sources. In particular, we evaluate the effect of key parameters at the levels of feature extraction (e.g., short-time Fourier transform (STFT) parameters) and model training (e.g., neural network (NN) architectures). We evaluate the performance of different settings in terms of precision and F-score, in a multiclass multilabel classification framework. In our previous work for localization of moving sound sources, we investigated feedforward NNs (FNNs) under different acoustic conditions and STFT parameters and showed that the presence of some reverberation in the training dataset can help in achieving better detection for the direction of arrival of the sources. In this article, we extend the work to show that the window size does not affect the performance of static sources but highly affects the performance of moving sources, a sequence length has a significant effect on the performance of recurrent architectures, and a temporal convolutional NN can outperform both recurrent and feedforward networks for moving sound sources.
更多查看译文
关键词
Direction-of-arrival estimation,Acoustics,Estimation,Convolutional neural networks,Feature extraction,Task analysis,Deep learning,Direction-of-arrival (DOA) detection,machine learning,microphone arrays,moving acoustic sources,neural networks (NNs)
AI 理解论文
溯源树
样例
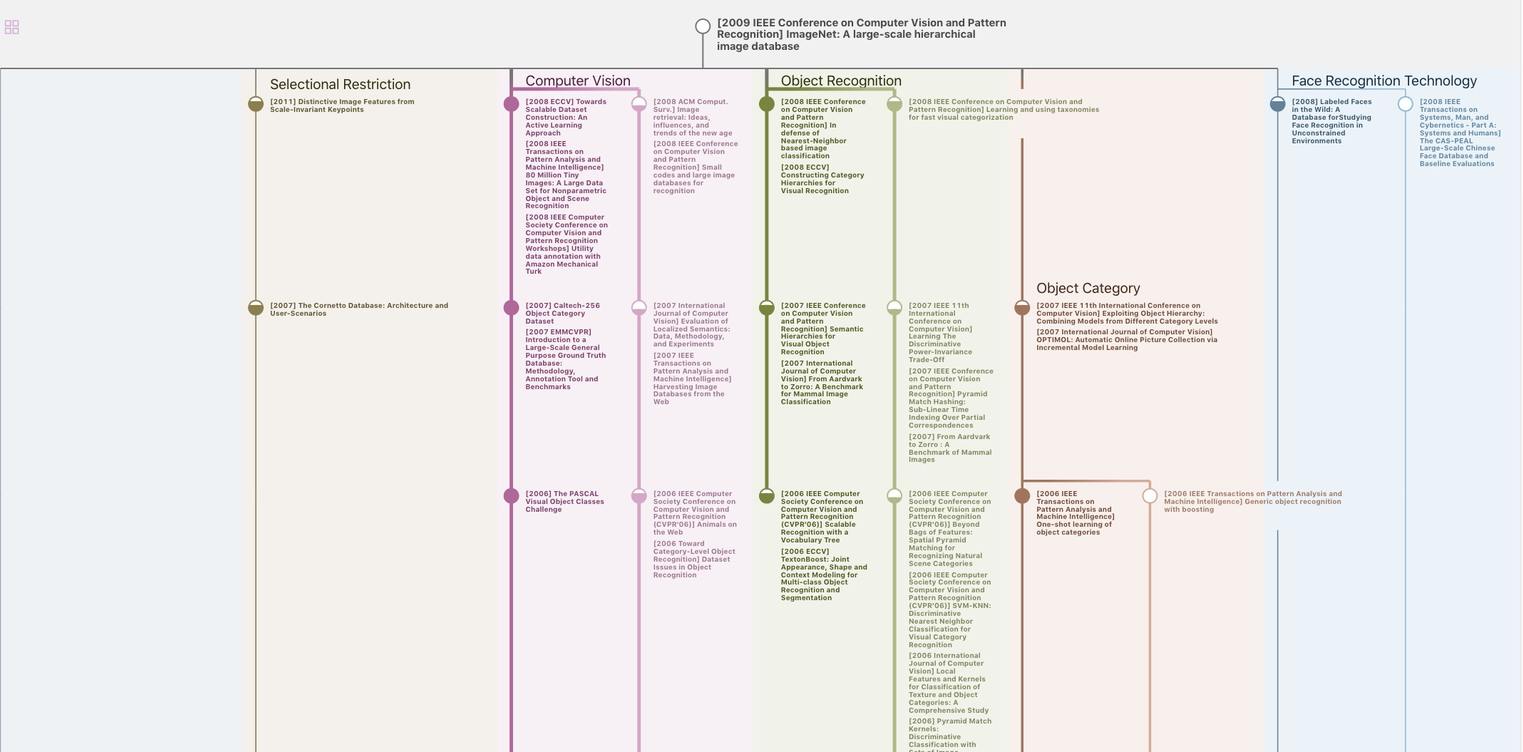
生成溯源树,研究论文发展脉络
Chat Paper
正在生成论文摘要