What Makes Data Suitable for a Locally Connected Neural Network? A Necessary and Sufficient Condition Based on Quantum Entanglement
NeurIPS(2023)
摘要
The question of what makes a data distribution suitable for deep learning is
a fundamental open problem. Focusing on locally connected neural networks (a
prevalent family of architectures that includes convolutional and recurrent
neural networks as well as local self-attention models), we address this
problem by adopting theoretical tools from quantum physics. Our main
theoretical result states that a certain locally connected neural network is
capable of accurate prediction over a data distribution if and only if the data
distribution admits low quantum entanglement under certain canonical partitions
of features. As a practical application of this result, we derive a
preprocessing method for enhancing the suitability of a data distribution to
locally connected neural networks. Experiments with widespread models over
various datasets demonstrate our findings. We hope that our use of quantum
entanglement will encourage further adoption of tools from physics for formally
reasoning about the relation between deep learning and real-world data.
更多查看译文
关键词
connected neural network,quantum,data suitable
AI 理解论文
溯源树
样例
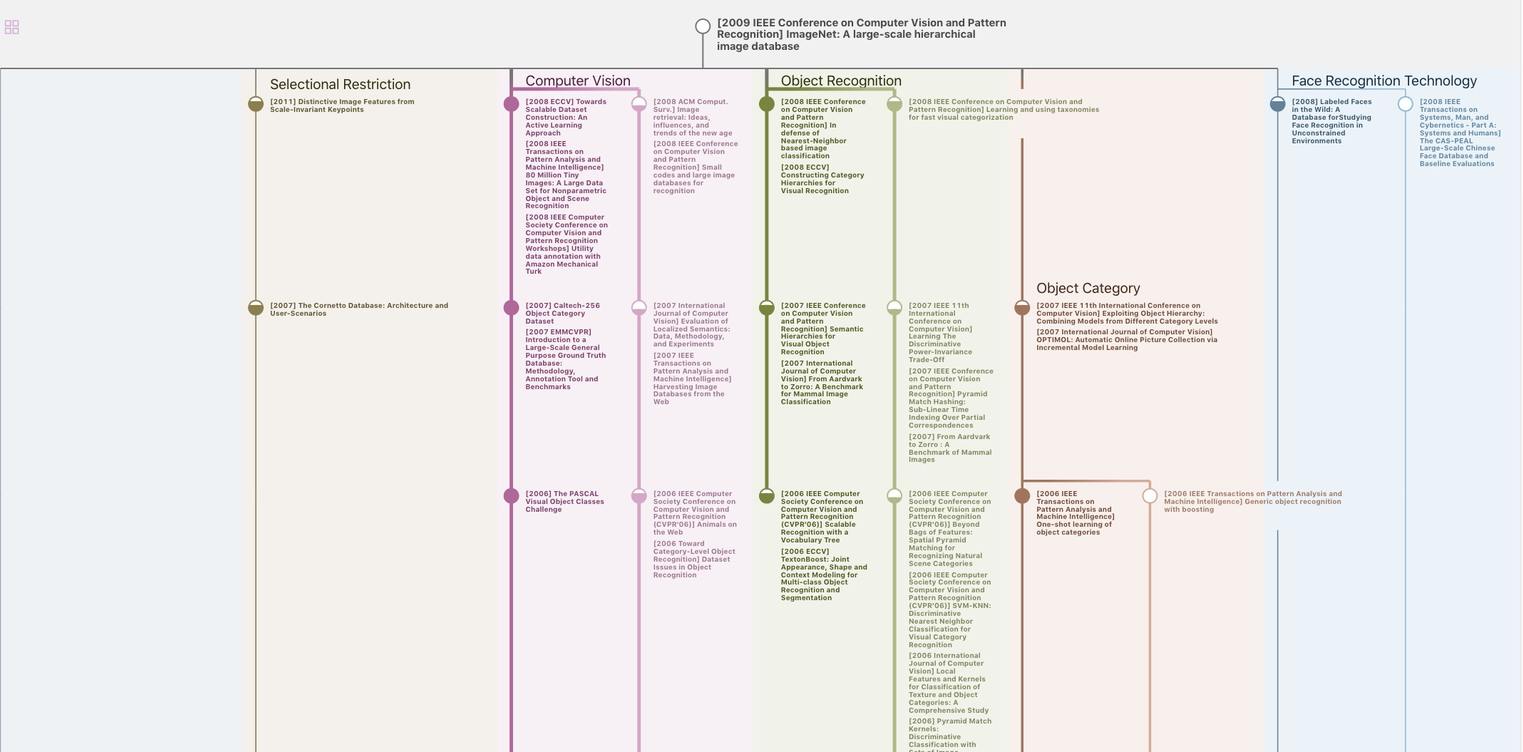
生成溯源树,研究论文发展脉络
Chat Paper
正在生成论文摘要