Learning Rationality in Potential Games
2023 62ND IEEE CONFERENCE ON DECISION AND CONTROL, CDC(2023)
Abstract
We propose a stochastic first-order algorithm to learn the rationality parameters of simultaneous and non-cooperative potential games, i.e., the parameters of the agents' optimization problems. Our technique combines an active-set step that enforces that the agents play at a Nash equilibrium and an implicit-differentiation step to update the estimates of the rationality parameters. We detail the convergence properties of our algorithm and perform numerical experiments on Cournot and congestion games. In practice, we show that our algorithm effectively finds high-quality solutions with minimal out-of-sample loss and scales to large datasets.
MoreTranslated text
Key words
Convex Optimization,Hyperparameter Optimization,Bandit Optimization,Regret Analysis,Prediction Accuracy
AI Read Science
Must-Reading Tree
Example
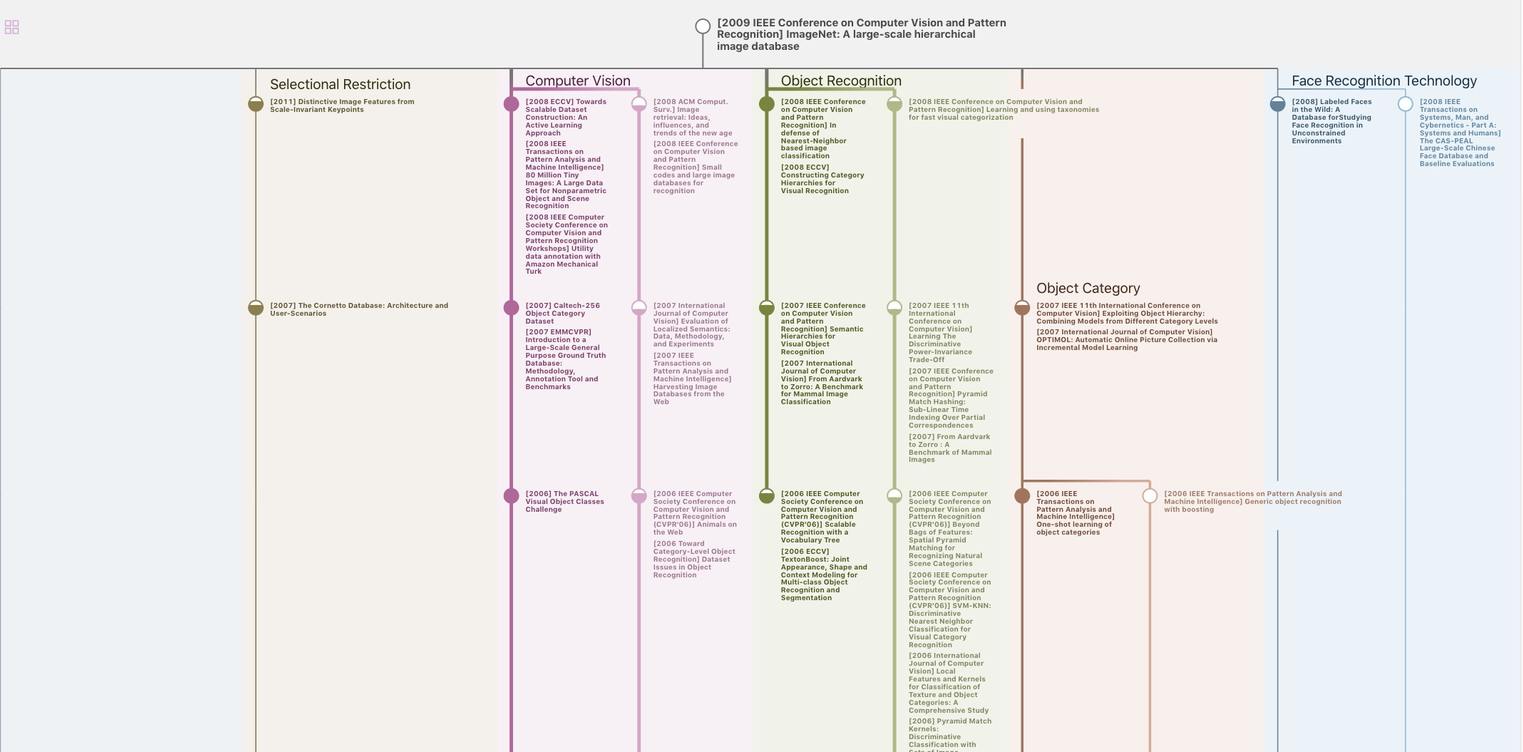
Generate MRT to find the research sequence of this paper
Chat Paper
Summary is being generated by the instructions you defined