Audio-Text Models Do Not Yet Leverage Natural Language
ICASSP 2023 - 2023 IEEE International Conference on Acoustics, Speech and Signal Processing (ICASSP)(2023)
Abstract
Multi-modal contrastive learning techniques in the audio-text domain have quickly become a highly active area of research. Most works are evaluated with standard audio retrieval and classification benchmarks assuming that (i) these models are capable of leveraging the rich information contained in natural language, and (ii) current benchmarks are able to capture the nuances of such information. In this work, we show that state-of-the-art audio-text models do not yet really understand natural language, especially contextual concepts such as sequential or concurrent ordering of sound events. Our results suggest that existing benchmarks are not sufficient to assess these models' capabilities to match complex contexts from the audio and text modalities. We propose a Transformer-based architecture and show that, unlike prior work, it is capable of modeling the sequential relationship between sound events in the text and audio, given appropriate benchmark data. We advocate for the collection or generation of additional, diverse, data to allow future research to fully leverage natural language for audio-text modeling.
MoreTranslated text
AI Read Science
Must-Reading Tree
Example
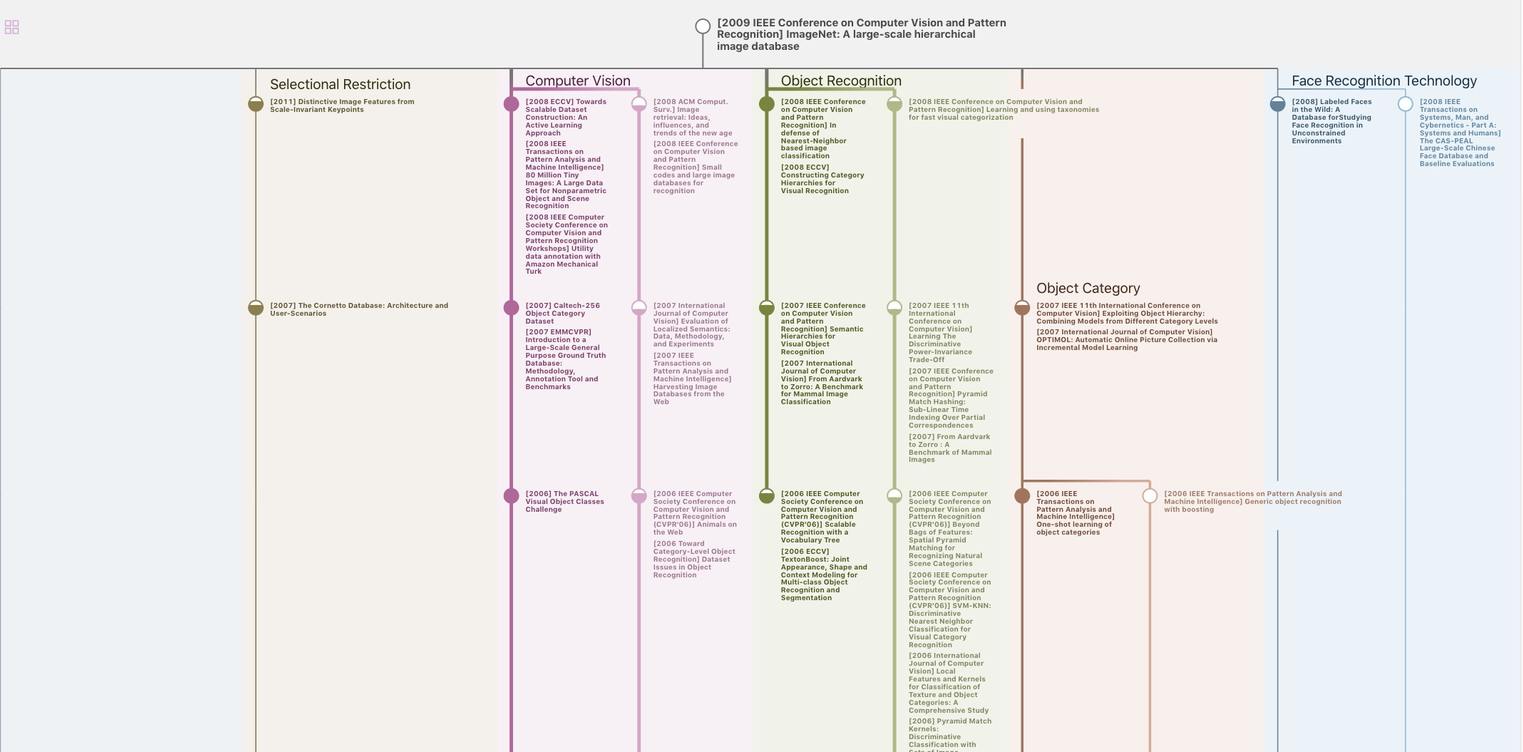
Generate MRT to find the research sequence of this paper
Chat Paper
Summary is being generated by the instructions you defined