Aerothermodynamic Simulators for Rocket Design using Neural Fields
arxiv(2023)
摘要
The typical size of computational meshes needed for realistic geometries and high-speed flow conditions makes Computational Fluid Dynamics (CFD) impractical for full-mission performance prediction and control. Reduced-Order Models (ROMs) in low-speed aerodynamics have come a long way in terms of reconstructing coherent flow patterns, thus enabling aerodynamic performance prediction. While many examples of ROMs exist for low-speed aerodynamics, there is no such broad literature for high-speed flows. We propose to use physics-enhanced neural fields for prediction of the steady, supersonic flow around a rocket for resolving: the bow shock profile, the boundary layer gradients and the wake over a range of incidences. This approach can lead to the construction of a computationally inexpensive, continuous aerothermodynamic model of a rocket at realistic flight conditions with applications to aerodynamic design and mission analysis and control. The use of neural fields allows to quickly and efficiently sweep the Angle of Attack (AoA) in a continuous manner, as compared to traditional CFD which requires running simulations for each discrete incidence value.
更多查看译文
关键词
rocket design
AI 理解论文
溯源树
样例
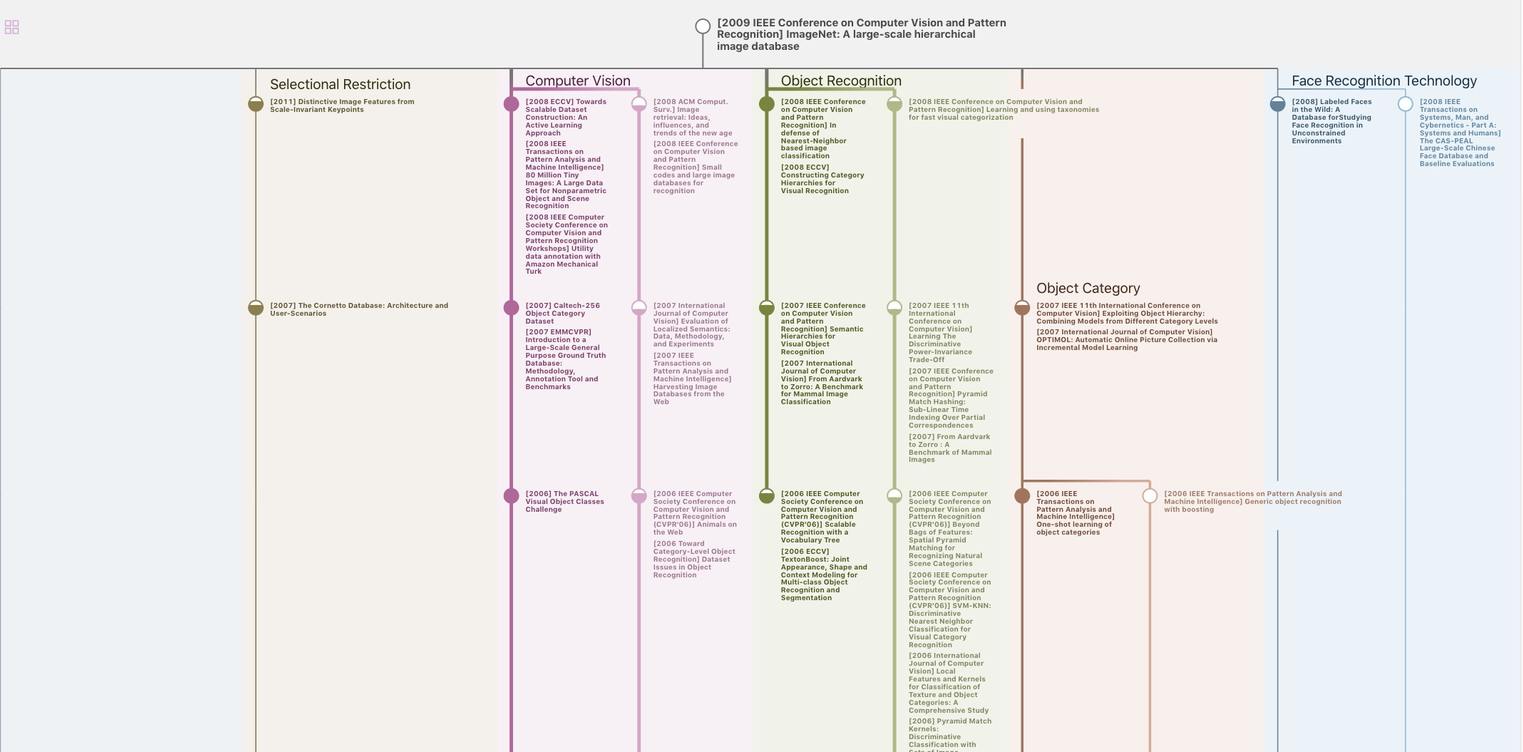
生成溯源树,研究论文发展脉络
Chat Paper
正在生成论文摘要