A Novel Machine Learning Based Bias Correction Method and Its Application to Sea Level in an Ensemble of Downscaled Climate Projections
Tellus: Series A, Dynamic Meteorology and Oceanography(2023)
摘要
A new machine learning based bias correction method is presented and applied to sea level in a regional climate model. The bias corrections derived using this method depend on the state of the model it corrects. This contrasts with conventional bias correction methods that operate on distributions of output variables. The dependence on model states allows for better performance on classical skill scores, but it also limits the applicability of the method to models that can perform hindcasts. A very large dataset of corrected hourly sea levels from many different emission scenarios is created. In total the dataset contains over 2600 model years and exists for seven different tide-gauge stations on the Swedish Baltic Sea coast. The prevalence of significant trends in yearly sea level maximum is found to be independent of emission scenario, suggesting that anthropogenic climate change is no significant driver of storm surge variability in the area. Lastly, the dataset is used to estimate return levels for very long return periods, and the block length used in the return level computation is found to affect the result at some stations. This suggests that the commonly used annual maximum approach is not always applicable for determining return levels for sea level.
更多查看译文
关键词
Bias corrections, Extreme sea levels, Neural network, Machine learning
AI 理解论文
溯源树
样例
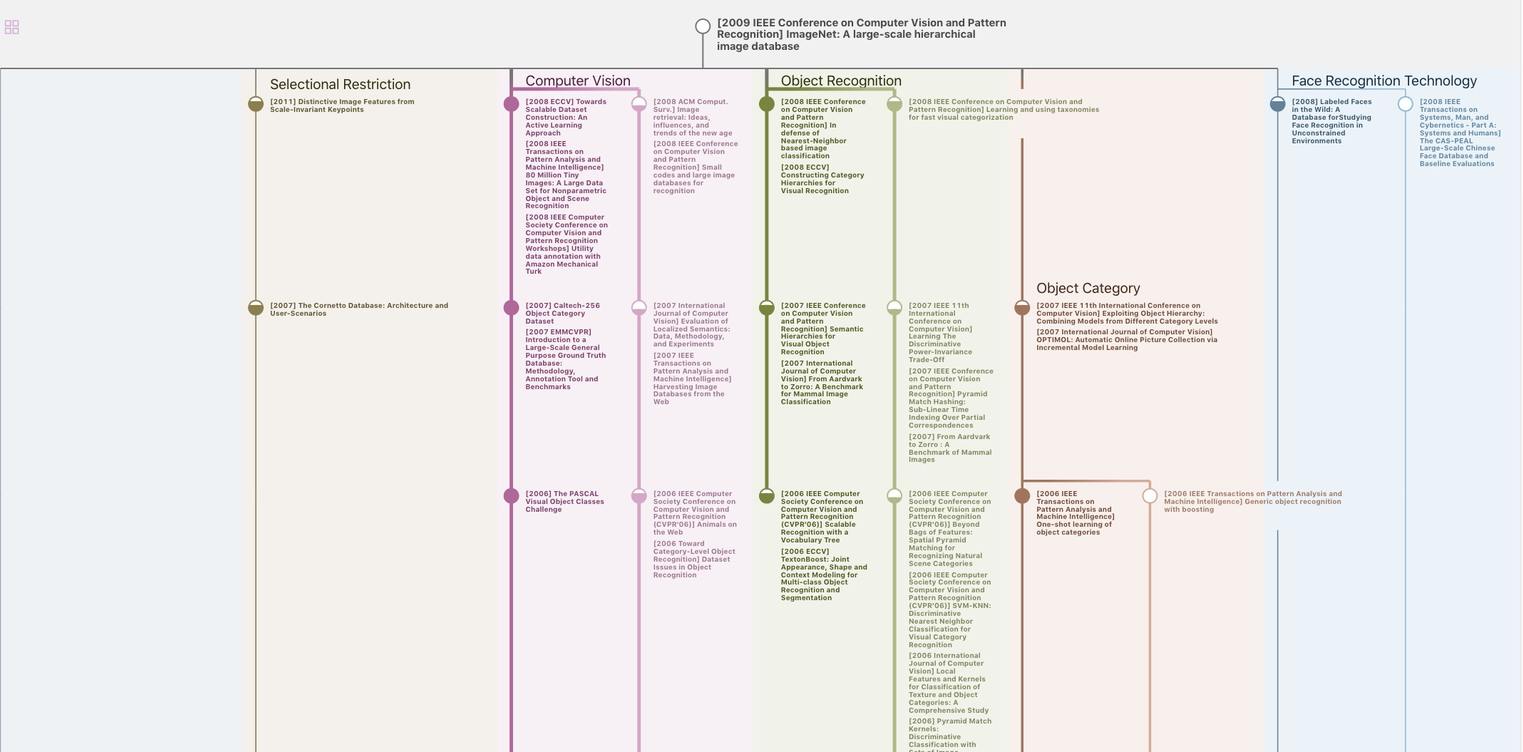
生成溯源树,研究论文发展脉络
Chat Paper
正在生成论文摘要