LION: Implicit Vision Prompt Tuning
AAAI 2024(2024)
摘要
Despite recent promising performances across a range of vision tasks, vision Transformers still have an issue of high computational costs.
Recently, vision prompt learning has provided an economical solution to this problem without fine-tuning the whole large-scale model.
However, the efficiency and effectiveness of existing models are still far from satisfactory due to the parameter cost of extensive prompt blocks and tricky prompt framework designs.
In this paper, we propose a light-weight prompt framework named impLicit vIsion prOmpt tuNing (LION), which is motivated by deep implicit models with stable low memory costs for various complex tasks.
In particular, we merely insect two equilibrium implicit layers in two ends of the pre-trained backbone with parameters frozen. Moreover, according to the lottery hypothesis, we further prune the parameters to relieve the computation burden in implicit layers. Various experiments have validated that our LION obtains promising performances on a wide range of datasets. Most importantly, LION reduces up to 11.5 % of training parameter numbers while obtaining higher performance than the state-of-the-art VPT, especially under challenging scenes. Furthermore, we find that our proposed LION has an excellent generalization performance, making it an easy way to boost transfer learning in the future.
更多查看译文
关键词
CV: Representation Learning for Vision
AI 理解论文
溯源树
样例
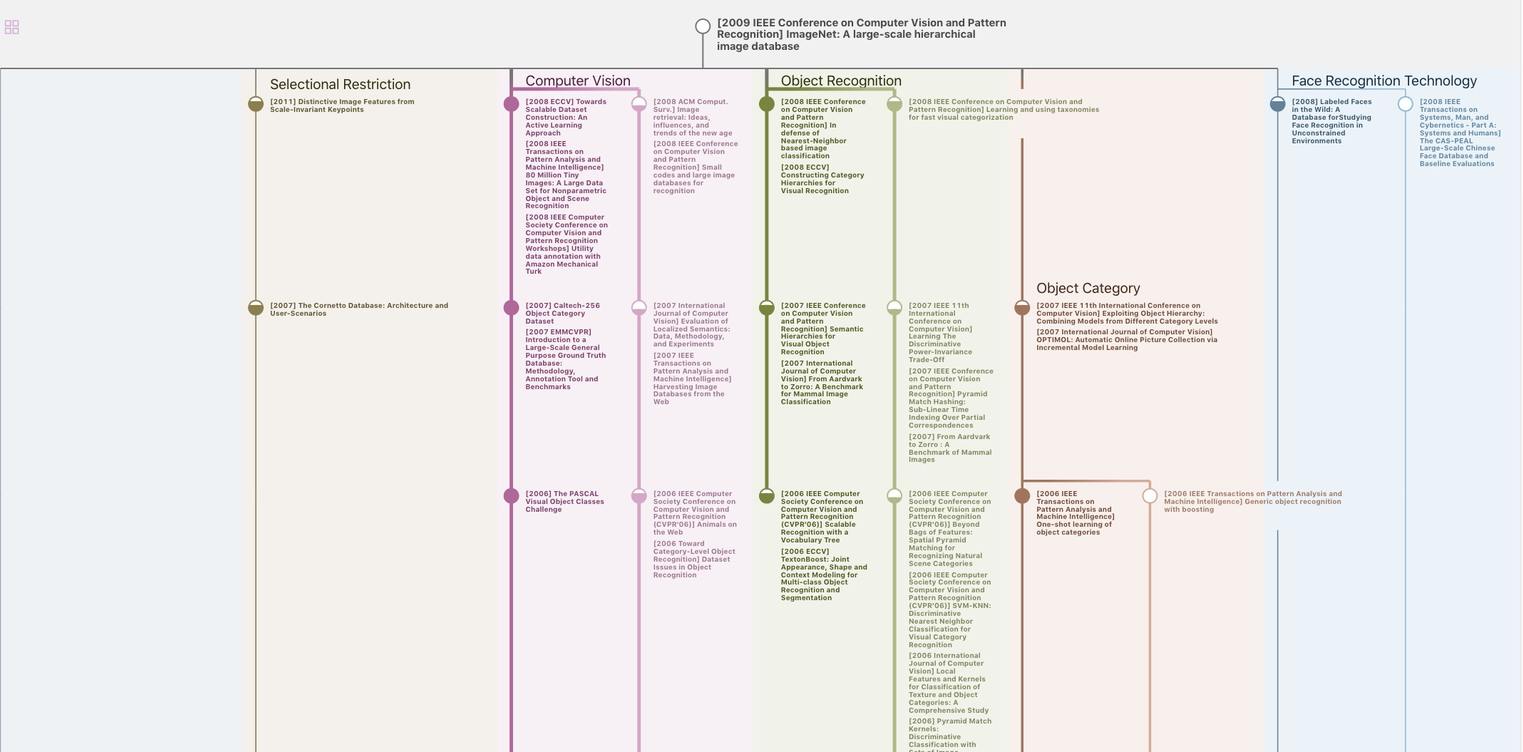
生成溯源树,研究论文发展脉络
Chat Paper
正在生成论文摘要