Active Learning-based Model Predictive Coverage Control
arxiv(2023)
摘要
The problem of coverage control, i.e., of coordinating multiple agents to optimally cover an area, arises in various applications. However, existing coverage algorithms face two major challenges: (1) dealing with nonlinear dynamics while respecting system and safety critical constraints, and (2) performing the task in an initially unknown environment. We solve the coverage problem by using a hierarchical framework, where references are calculated at a central server and passed to the agents' local model predictive control (MPC) tracking schemes. Furthermore, a probabilistic exploration-exploitation trade-off is deployed to ensure that the environment is actively explored by the agents. In addition, we derive a control framework that avoids the hierarchical structure by integrating the reference optimization in the MPC formulation. Active learning is then performed drawing inspiration from Upper Confidence Bound (UCB) approaches. We guarantee recursive feasibility and convergence to an optimal configuration for all developed control architectures. Furthermore, all methods are tested and compared on hardware using a miniature car platform.
更多查看译文
关键词
Coverage Control,NL Predictive Control,Cooperative Control,Machine Learning,Agents and Autonomous Systems
AI 理解论文
溯源树
样例
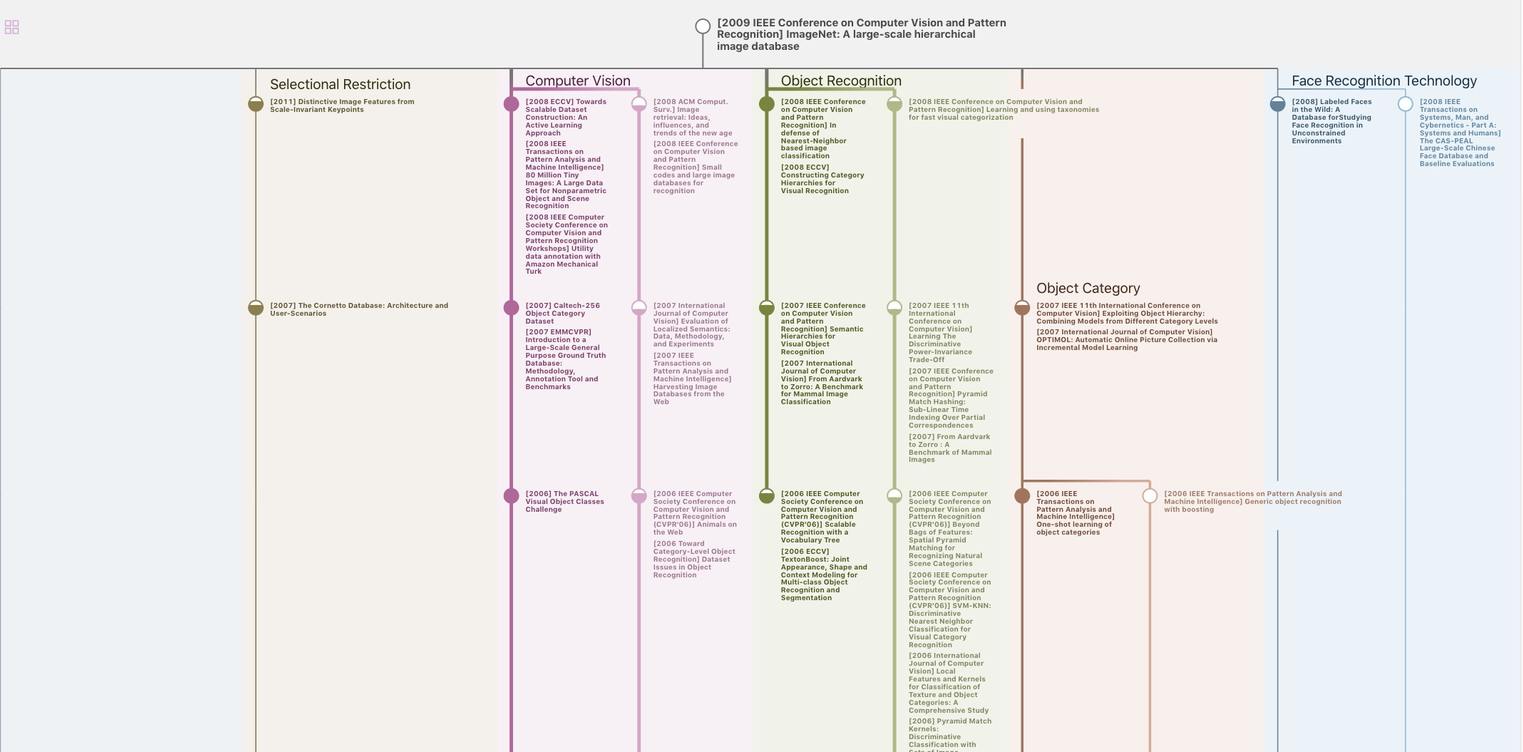
生成溯源树,研究论文发展脉络
Chat Paper
正在生成论文摘要