Error Bounds for Kernel-Based Linear System Identification with Unknown Hyperparameters
arxiv(2023)
摘要
The kernel-based method has been successfully applied in linear system identification using stable kernel designs. From a Gaussian process perspective, it automatically provides probabilistic error bounds for the identified models from the posterior covariance, which are useful in robust and stochastic control. However, the error bounds require knowledge of the true hyperparameters in the kernel design and are demonstrated to be inaccurate with estimated hyperparameters for lightly damped systems or in the presence of high noise. In this work, we provide reliable quantification of the estimation error when the hyperparameters are unknown. The bounds are obtained by first constructing a high-probability set for the true hyperparameters from the marginal likelihood function and then finding the worst-case posterior covariance within the set. The proposed bound is proven to contain the true model with a high probability and its validity is verified in numerical simulation.
更多查看译文
关键词
linear system identification,hyperparameters,kernel-based
AI 理解论文
溯源树
样例
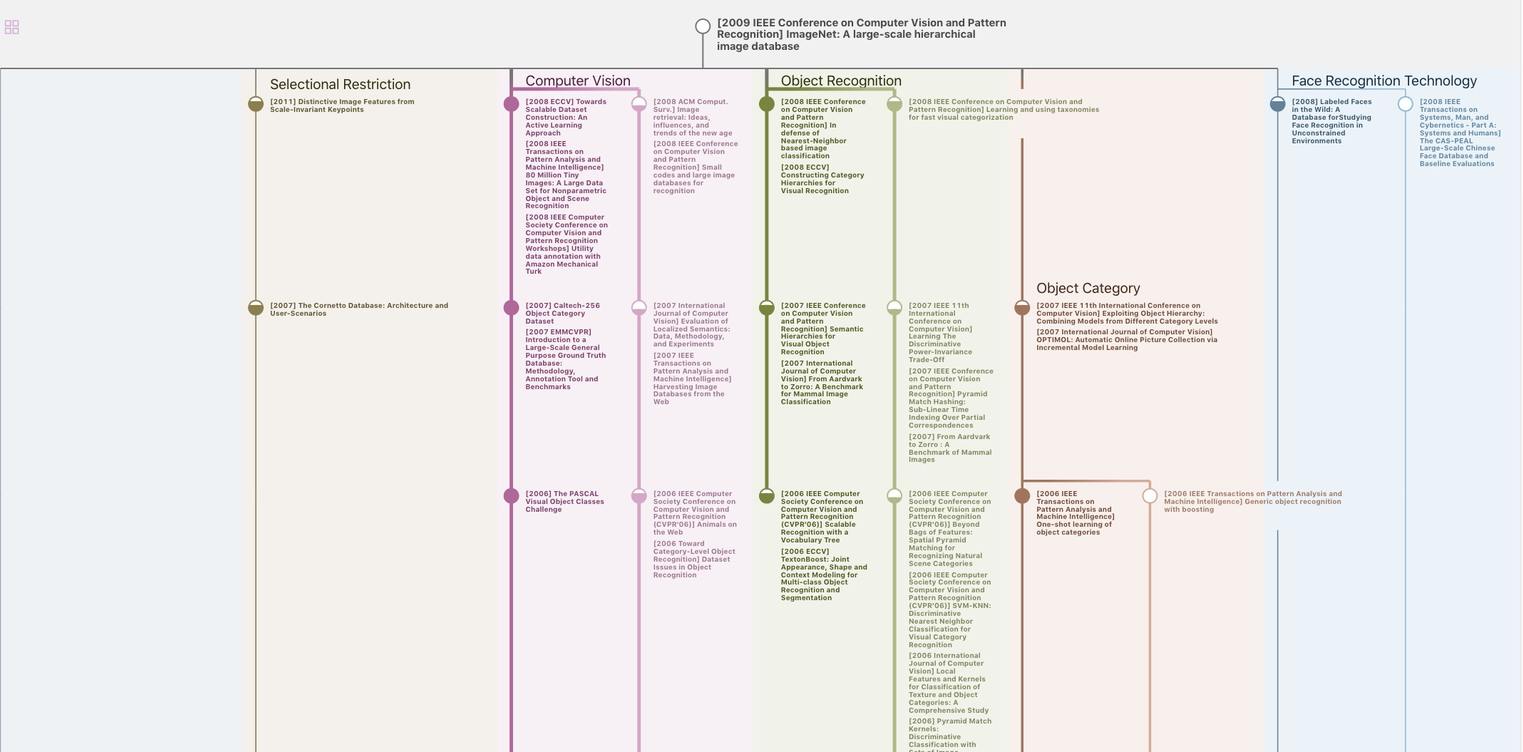
生成溯源树,研究论文发展脉络
Chat Paper
正在生成论文摘要