Sample size determination via learning-type curves
arxiv(2023)
摘要
This paper is concerned with sample size determination methodology for prediction models. We propose combining the individual calculations via a learning-type curve. We suggest two distinct ways of doing so, a deterministic skeleton of a learning curve and a Gaussian process centred upon its deterministic counterpart. We employ several learning algorithms for modelling the primary endpoint and distinct measures for trial efficacy. We find that the performance may vary with the sample size, but borrowing information across sample size universally improves the performance of such calculations. The Gaussian process-based learning curve appears more robust and statistically efficient, while computational efficiency is comparable. We suggest that anchoring against historical evidence when extrapolating sample sizes should be adopted when such data are available. The methods are illustrated on binary and survival endpoints.
更多查看译文
关键词
sample size determination,sample size,curves,learning-type
AI 理解论文
溯源树
样例
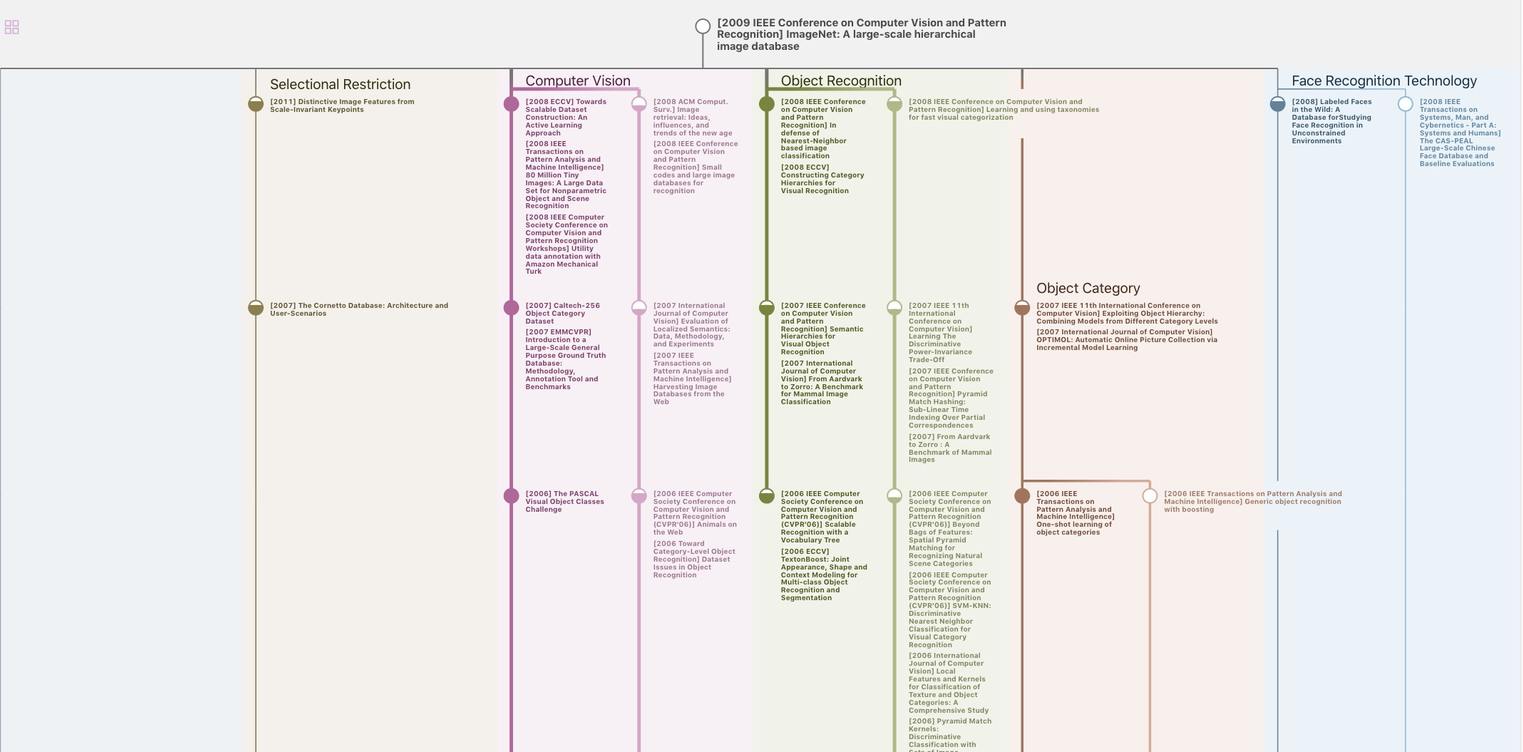
生成溯源树,研究论文发展脉络
Chat Paper
正在生成论文摘要