Part-and-whole: A novel framework for deformable medical image registration
Applied Intelligence(2022)
摘要
Medical image registration is a vital initial step in medical image analysis that aims to establish accurate correspondence between image pairs. Various methods have achieved impressive results on this task over the last few years. However, the existing methods fail to achieve desirable registration accuracy because they do not pay sufficient attention on the integration between global and local information in medical images and ignore the geometric constraints in anatomical regions. The registration results still need to be refined to become sufficiently accurate for clinical application considering the current situation. In this paper, we propose a novel part-and-whole registration framework for 3D medical images. The proposed framework consists of two encoder-decoder architectures which take image pairs and patch pairs as inputs, respectively, and estimate the deformation field as the result. In particular, we propose a multi-view feature slicing attention module and embed it into the part-and-whole architecture to enhance the context relation between the local and global information. A voxel-shape-aware loss function is developed to refine the registration performance. We validate the proposed framework on several 3D human brain magnetic resonance image datasets. Results show that our method consistently outperforms recent state-of-the-art baselines.
更多查看译文
关键词
Image registration, Convolutional neural network, Unsupervised learning, Brain MR images
AI 理解论文
溯源树
样例
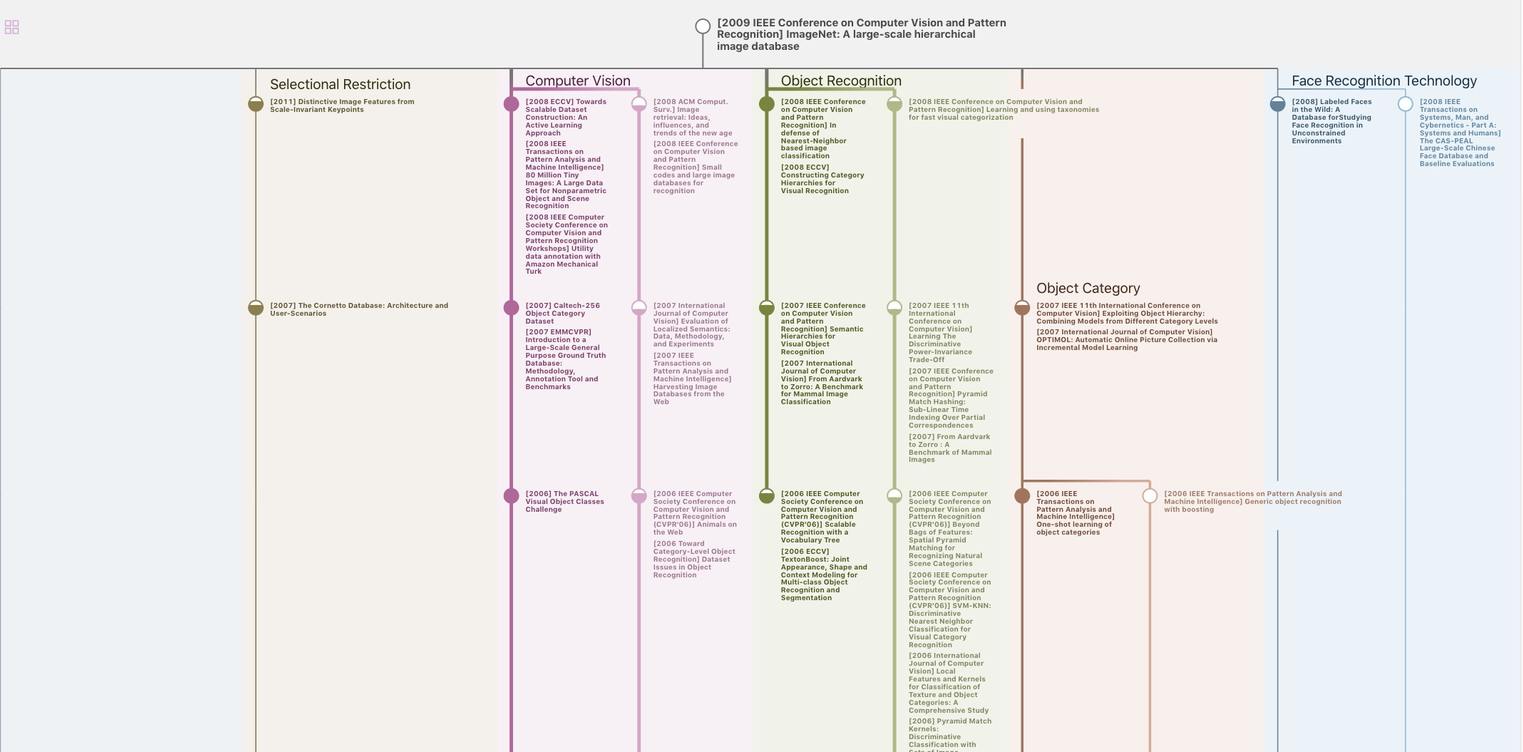
生成溯源树,研究论文发展脉络
Chat Paper
正在生成论文摘要