A novel approach to discover frequent weighted subgraphs using the average measure
Applied Intelligence(2023)
摘要
Mining a weighted single large graph has recently attracted many researchers. The WeGraMi algorithm is considered the state-of-the-art among current approaches. It uses a MaxMin measure to calculate weights for all mined subgraphs. However, if all values in the domain have the same role and the user needs an average value of that domain, then we have to use another measure. In this paper, we introduce a novel algorithm called AWeGraMi (Average Weighted Graph Mining) to solve the above problem, and our method calculates the weight based on the average of all values in the domain. We also apply the MaxMin measure as an upper-bound to prune the search space. The new algorithm can mine all frequent weighted subgraphs effectively. Our experiments on the directed and undirected datasets have shown that AWeGraMi has better performance in comparison to post-processing GraMi for all three criteria: search space (the number of candidate subgraphs), running time, and memory consumption.
更多查看译文
关键词
Single large, Weighted graph, Weighted subgraph mining, Upper bound, Data mining
AI 理解论文
溯源树
样例
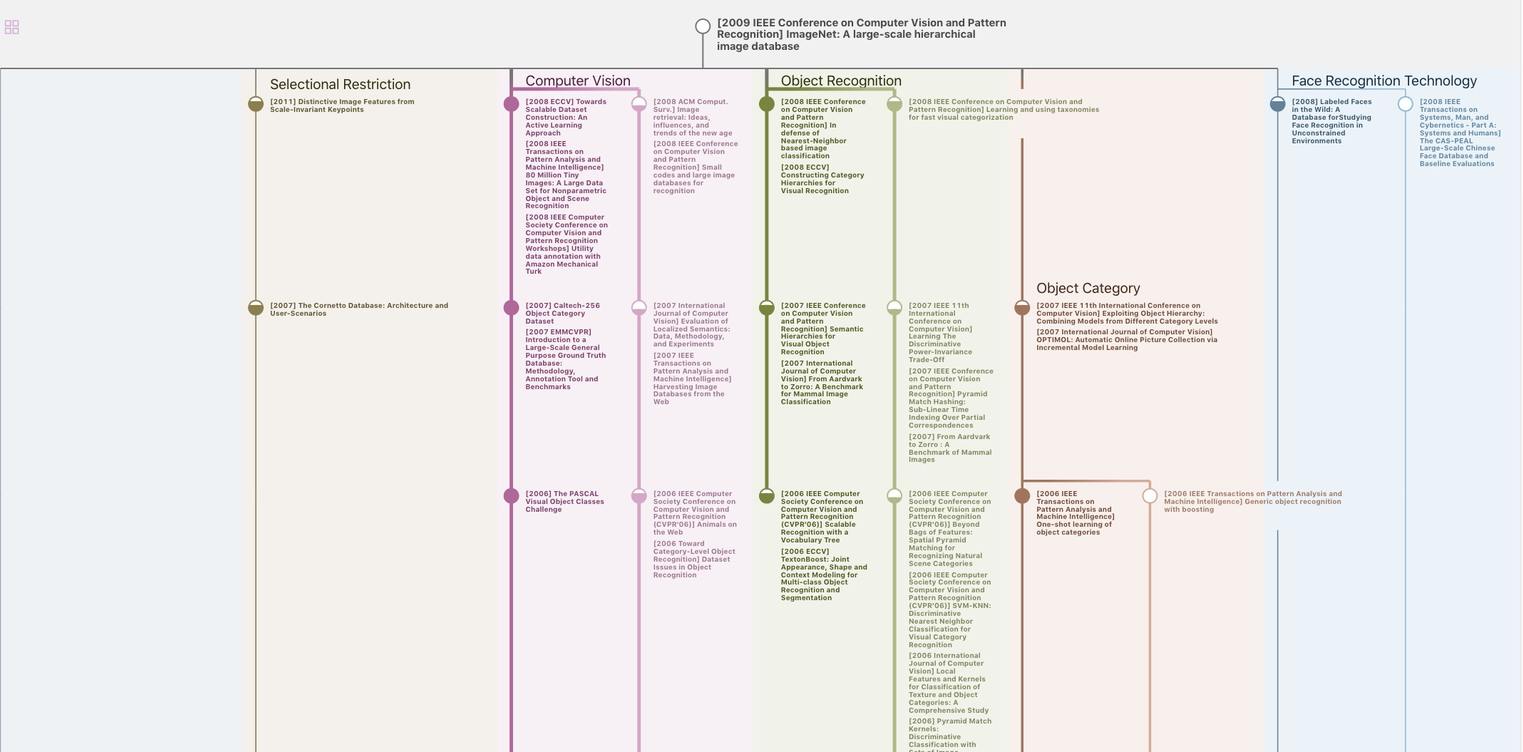
生成溯源树,研究论文发展脉络
Chat Paper
正在生成论文摘要