Statistics and Machine Learning in Mass Spectrometry-Based Metabolomics Analysis.
Methods in molecular biology (Clifton, N.J.)(2023)
摘要
In this chapter, we review the cutting-edge statistical and machine learning methods for missing value imputation, normalization, and downstream analyses in mass spectrometry metabolomics studies, with illustration by example datasets. The missing peak recovery includes simple imputation by zero or limit of detection, regression-based or distribution-based imputation, and prediction by random forest. The batch effect can be removed by data-driven methods, internal standard-based, and quality control sample-based normalization. We also summarize different types of statistical analysis for metabolomics and clinical outcomes, such as inference on metabolic biomarkers, clustering of metabolomic profiles, metabolite module building, and integrative analysis with transcriptome.
更多查看译文
关键词
Imputation,Integrative analysis,Mass spectrometry,Metabolomics,Normalization,Statistical and machine learning
AI 理解论文
溯源树
样例
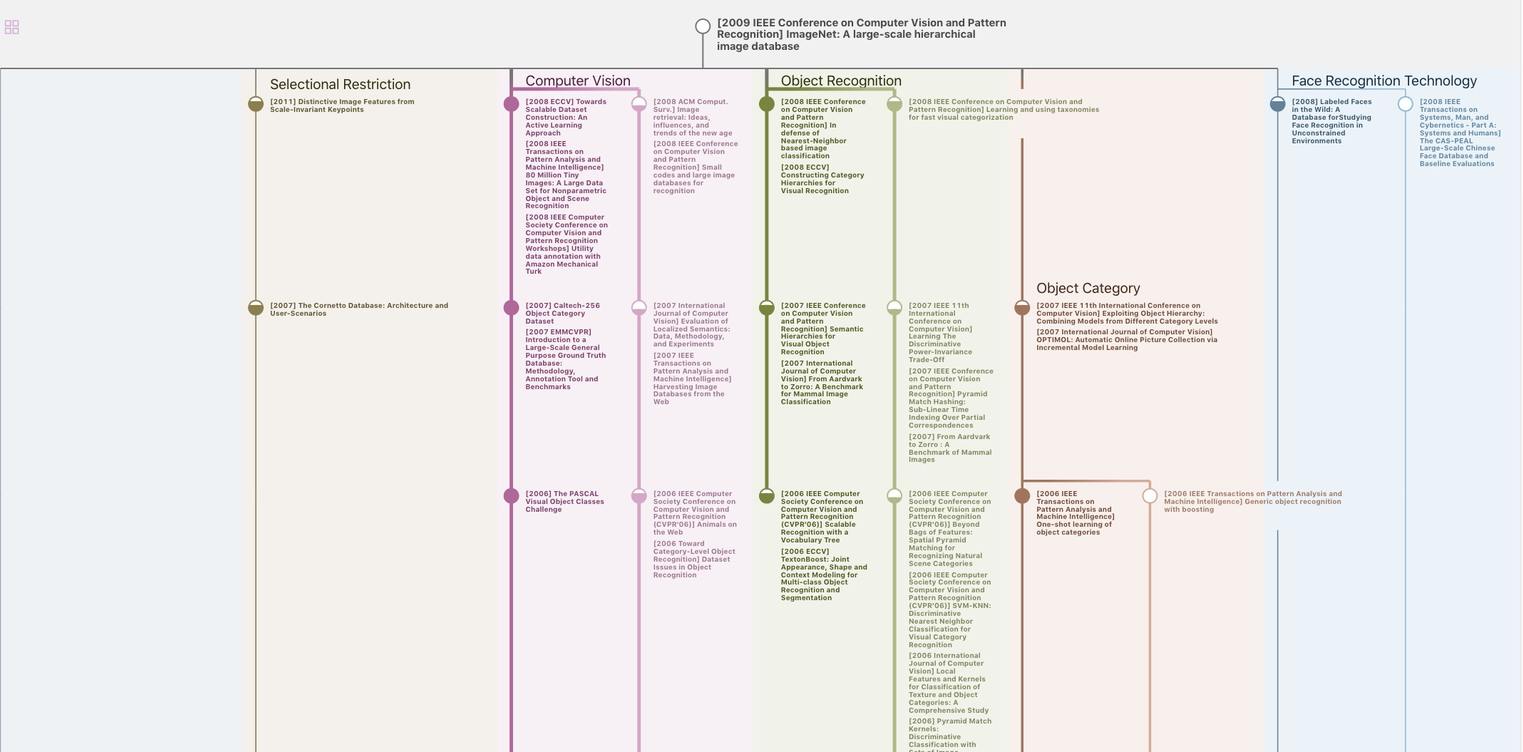
生成溯源树,研究论文发展脉络
Chat Paper
正在生成论文摘要