InCrowdFormer: On-Ground Pedestrian World Model From Egocentric Views
arxiv(2023)
摘要
We introduce an on-ground Pedestrian World Model, a computational model that can predict how pedestrians move around an observer in the crowd on the ground plane, but from just the egocentric-views of the observer. Our model, InCrowdFormer, fully leverages the Transformer architecture by modeling pedestrian interaction and egocentric to top-down view transformation with attention, and autoregressively predicts on-ground positions of a variable number of people with an encoder-decoder architecture. We encode the uncertainties arising from unknown pedestrian heights with latent codes to predict the posterior distributions of pedestrian positions. We validate the effectiveness of InCrowdFormer on a novel prediction benchmark of real movements. The results show that InCrowdFormer accurately predicts the future coordination of pedestrians. To the best of our knowledge, InCrowdFormer is the first-of-its-kind pedestrian world model which we believe will benefit a wide range of egocentric-view applications including crowd navigation, tracking, and synthesis.
更多查看译文
关键词
views,model,world,on-ground
AI 理解论文
溯源树
样例
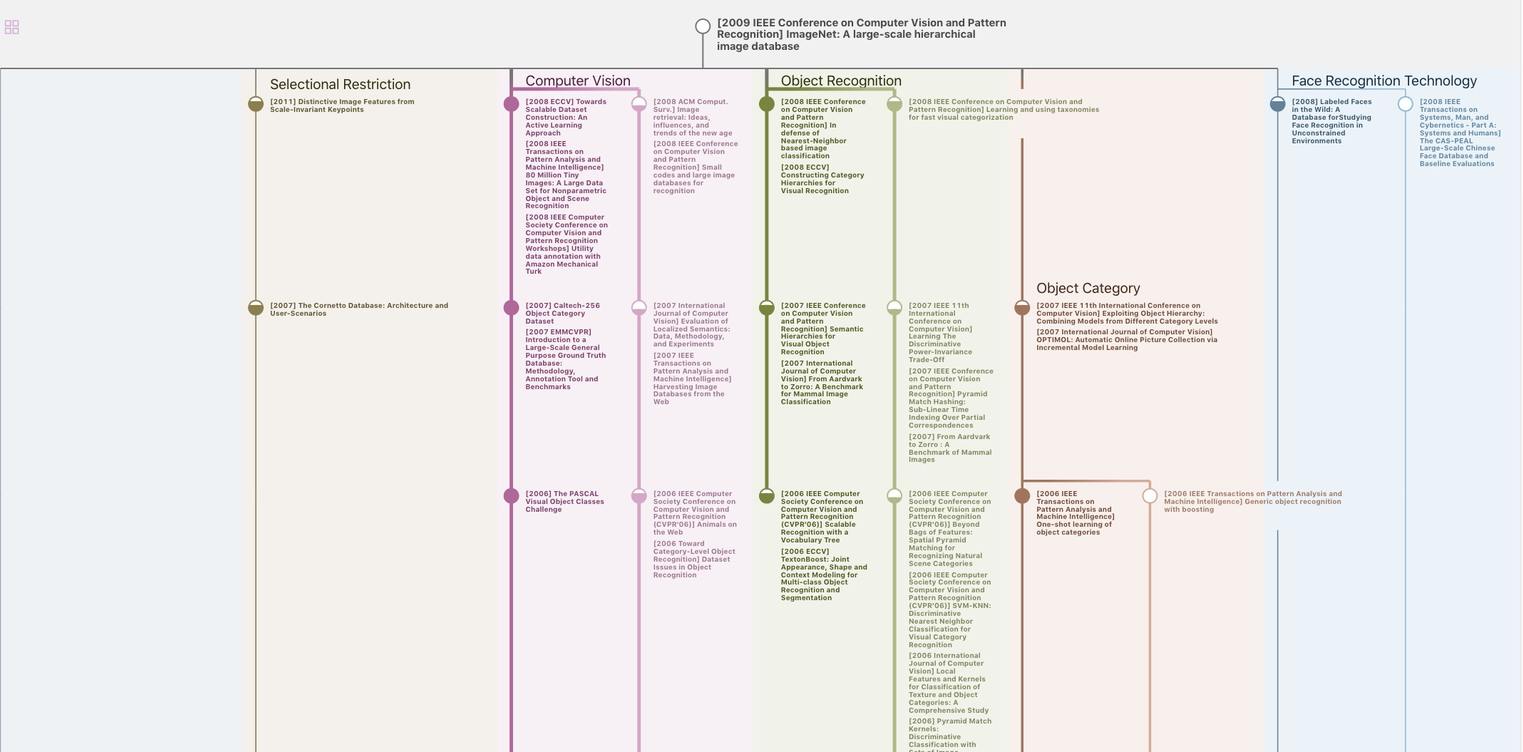
生成溯源树,研究论文发展脉络
Chat Paper
正在生成论文摘要