Reduction of rain-induced errors for wind speed estimation on SAR observations using convolutional neural networks
arxiv(2023)
摘要
Synthetic Aperture Radar is known to be able to provide high-resolution estimates of surface wind speed. These estimates usually rely on a Geophysical Model Function (GMF) that has difficulties accounting for non-wind processes such as rain events. Convolutional neural network, on the other hand, have the capacity to use contextual information and have demonstrated their ability to delimit rainfall areas. By carefully building a large dataset of SAR observations from the Copernicus Sentinel-1 mission, collocated with both GMF and atmospheric model wind speeds as well as rainfall estimates, we were able to train a wind speed estimator with reduced errors under rain. Collocations with in-situ wind speed measurements from buoys show a root mean square error that is reduced by 27% (resp. 45%) under rainfall estimated at more than 1 mm/h (resp. 3 mm/h). These results demonstrate the capacity of deep learning models to correct rain-related errors in SAR products.
更多查看译文
关键词
wind speed estimation,sar observations,neural networks,rain-induced
AI 理解论文
溯源树
样例
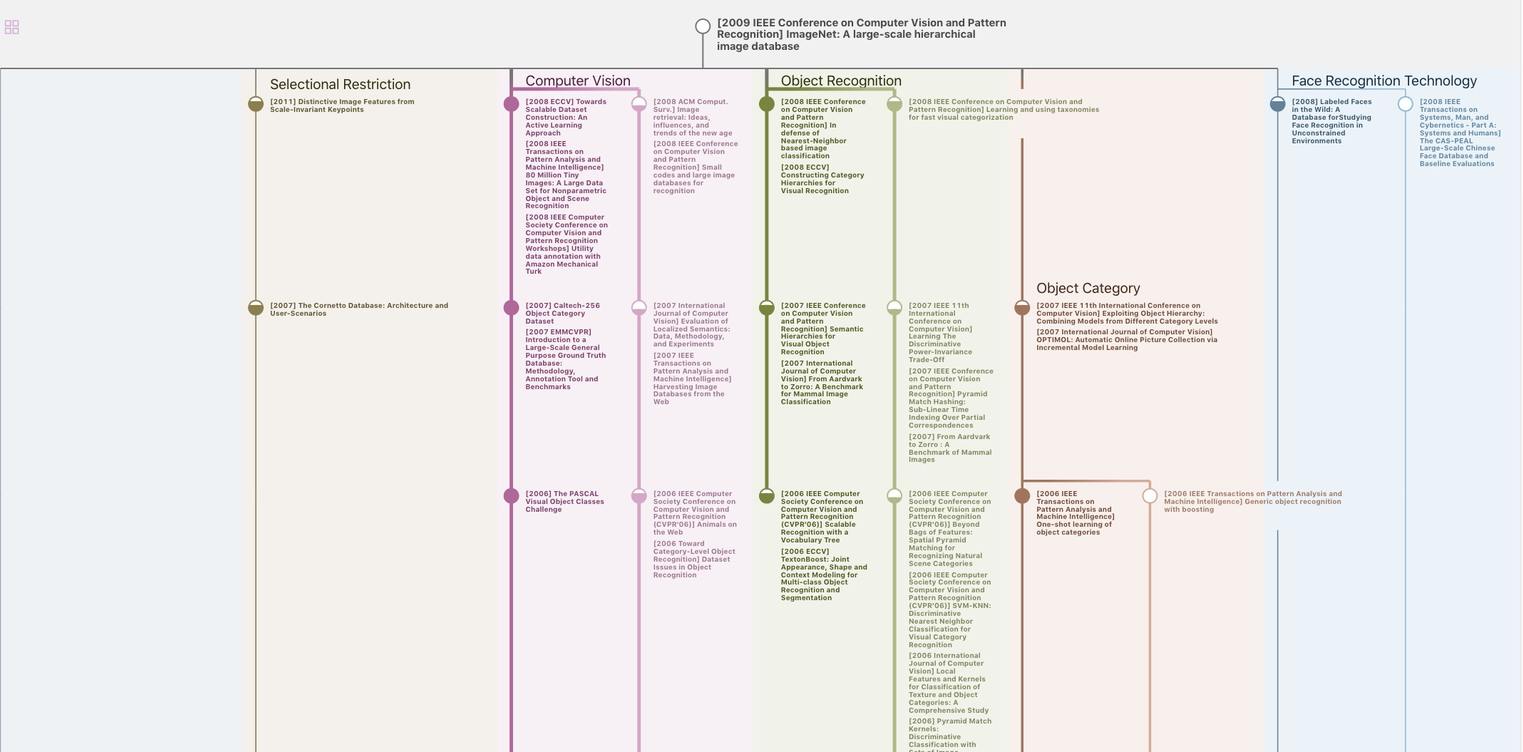
生成溯源树,研究论文发展脉络
Chat Paper
正在生成论文摘要