Metric-Free Exploration for Topological Mapping by Task and Motion Imitation in Feature Space
RSS 2023(2023)
Abstract
We propose DeepExplorer, a simple and lightweight metric-free exploration method for topological mapping of unknown environments. It performs task and motion planning (TAMP) entirely in image feature space. The task planner is a recurrent network using the latest image observation sequence to hallucinate a feature as the next-best exploration goal. The motion planner then utilizes the current and the hallucinated features to generate an action taking the agent towards that goal. Our novel feature hallucination enables imitation learning with deep supervision to jointly train the two planners more efficiently than baseline methods. During exploration, we iteratively call the two planners to predict the next action, and the topological map is built by constantly appending the latest image observation and action to the map and using visual place recognition (VPR) for loop closing.
The resulting topological map efficiently represents an environment’s connectivity and traversability, so it can be used for tasks such as visual navigation. We show DeepExplorer’s exploration efficiency and strong sim2sim generalization capability on large-scale simulation datasets like Gibson and MP3D. Its effectiveness is further validated via the image-goal navigation performance on the resulting topological map. We further show its strong zero-shot sim2real generalization capability in real-world experiments. The source code is available at https://ai4ce.github.io/DeepExplorer/.
MoreTranslated text
Key words
robotics,academic conference
AI Read Science
Must-Reading Tree
Example
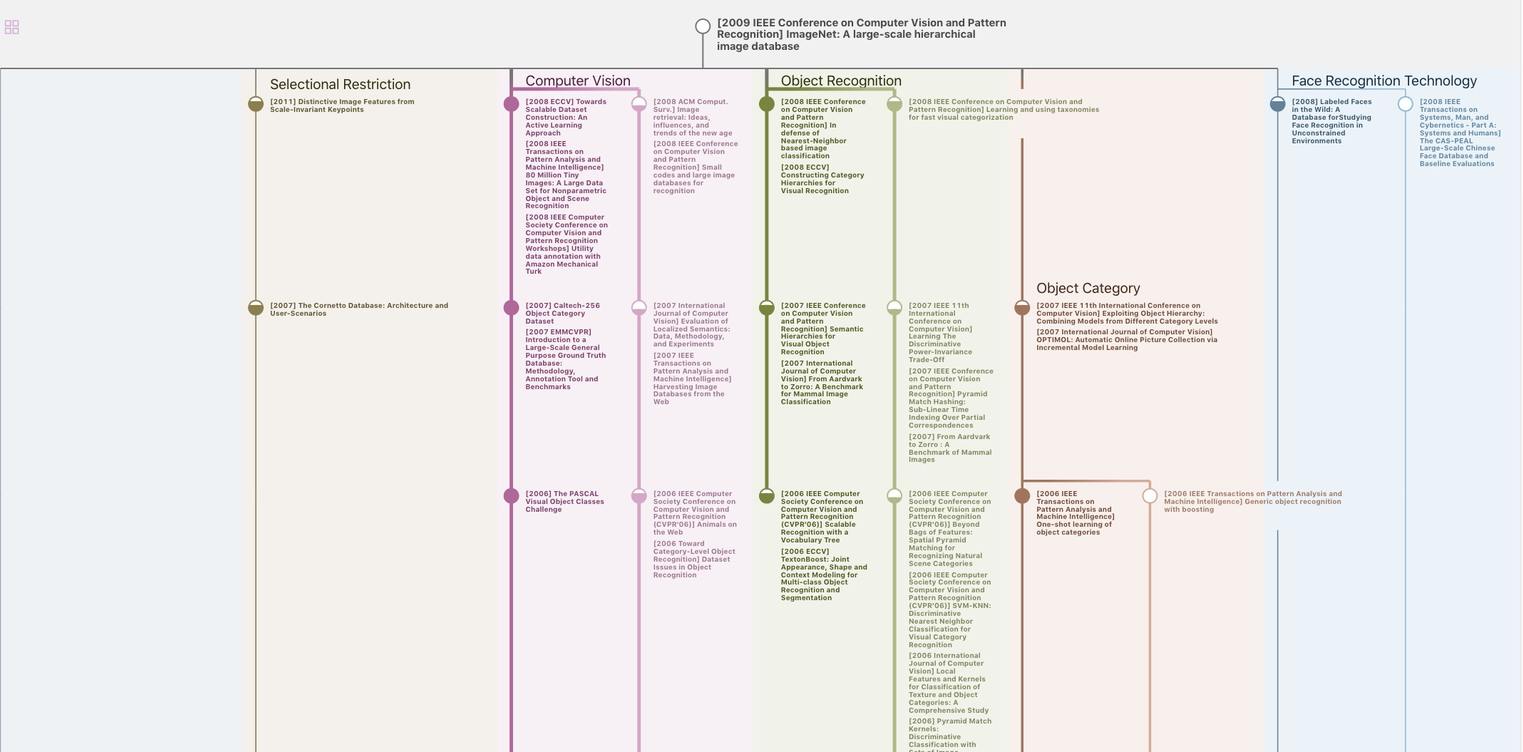
Generate MRT to find the research sequence of this paper
Chat Paper
Summary is being generated by the instructions you defined