Real-time detection algorithm of helmet and reflective vest based on improved YOLOv5
Journal of Real-Time Image Processing(2023)
Abstract
To address the current problems of helmet and reflective vest detection algorithms, such as long inference times, high hardware requirements, and difficulty detecting small objects, an improved real-time detection algorithm based on YOLOv5 is proposed. First, the Mosaic-9 data enhancement method is adopted to expand the number of image splicings from four to nine, enriching the small-scale sample dataset. Minimum rectangular area cropping is used instead of the original random cropping to improve the training efficiency. Then, the EIOU (Efficient Intersection Over Union) loss is used as the loss function, and the focal loss is introduced to focus on the high-quality anchor box, achieving the purpose of accelerating the convergence speed and improving regression accuracy. Next, the CBAM-CA (Convolutional Block Attention Module—Coordinate Attention) hybrid attention mechanism is added to the network model. The purpose of the model is to focus on the small object’s spatial and channel feature information to improve the small object’s detection performance. Finally, a new scale object detection layer is added to learn the multilevel features of the dense object. The experimental results show that the improved model’s mAP (mean average precision) is 94.9%, an improvement of 7.0% over the original model. The size of the improved model is 4.49 MB, and the mean FPS (Frames Per Second) is 62. This algorithm guarantees a small model size while significantly increasing mAP, which is suitable for deployment on low computing power platforms.
MoreTranslated text
Key words
Object detection, Helmet, Reflective vest, YOLOv5, Deep learning
AI Read Science
Must-Reading Tree
Example
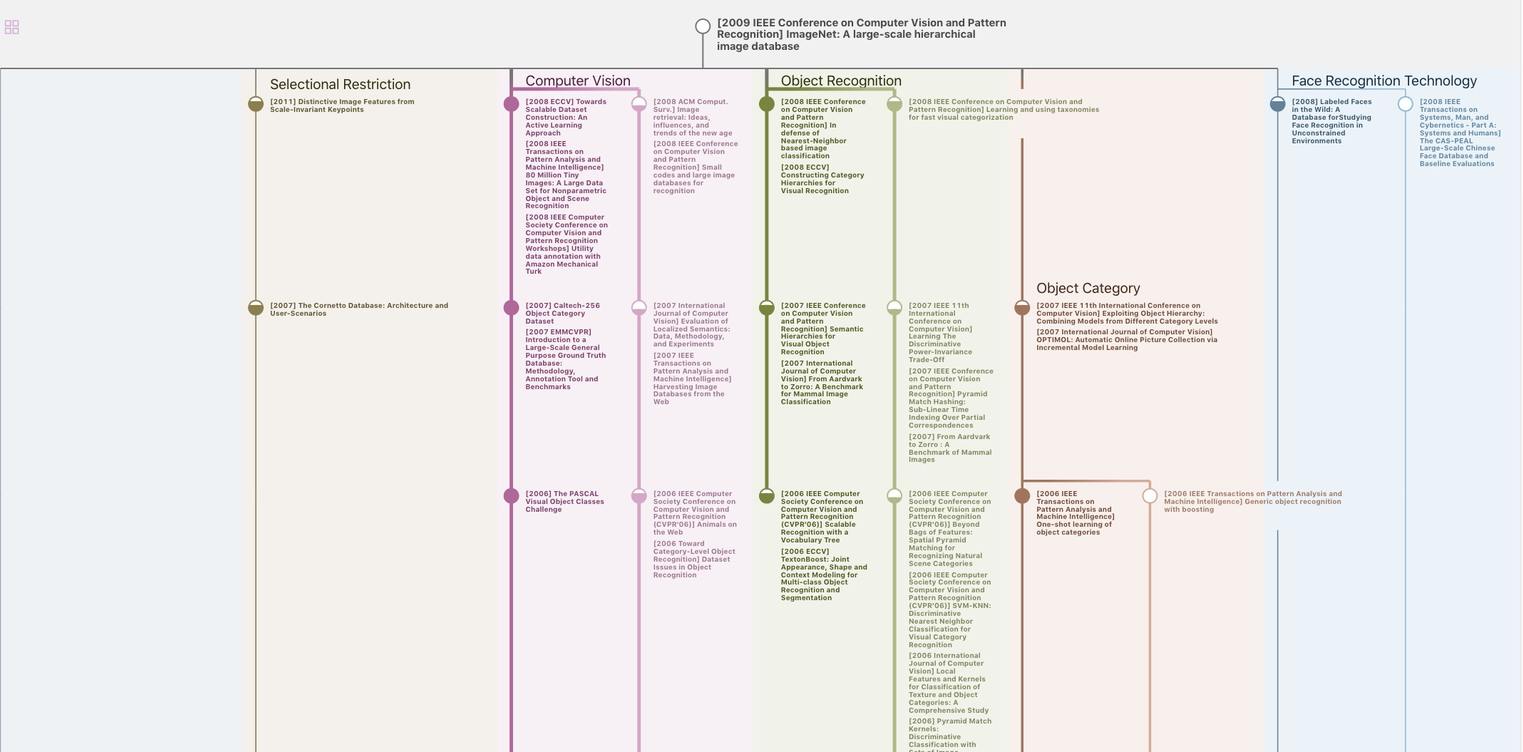
Generate MRT to find the research sequence of this paper
Chat Paper
Summary is being generated by the instructions you defined