Quantification of Neoantigen-Mediated Immunoediting in Cancer Evolution-Letter.
Cancer research(2023)
摘要
Wu and colleagues suggested immunoediting signals in primary tumor genomics data from The Cancer Genome Atlas (TCGA; ref. 1). Neoantigens were predicted from the calculated HLA affinities of mutated peptides.The study contradicts our former systematic immunoediting analysis, where we found that neoantigen depletion signals are undetectable and pointed out that HLA affinity–based neoantigen prediction accuracies are likely too low for any signal to be detectable (2). In line with this conclusion, a later consortium effort suggested that on average only 6% of HLA affinity–based neoantigen predictions are correct (3), implying that ± 94% of perceived immune selection signals are random noise. Any analysis indicative of (strong) HLA affinity–based immune selection should thus be critically interpreted, and we believe this is lacking in the Wu study.This is illustrated by the ESRNA analysis, one of the study's two approaches (the other being ESCCF). The authors developed this statistic to quantify the difference between mRNA expression of neoantigenic and nonantigenic mutations. Negative ESRNA values were then demonstrated in most cancer types and in a pan cancer dataset. This implies lower mRNA expression of genes containing neoantigenic mutations and is interpreted by the authors as a signal indicative of the escape phase of immunoediting. However, this interpretation relies on the underlying assumption that gene expression is unrelated to HLA affinity. To demonstrate that this assumption is violated and that the reported negative ESRNA values are not caused by immunoediting, we applied the authors’ published ESRNA code on their TCGA-derived somatic mutation dataset as well as on two independent, in silico-generated somatic mutation datasets (one previously published, trinucleotide substitution type-matched dataset with gene expression data derived from TCGA, the other newly generated with gene expression data derived from GTEx). While no (immune) selection signals are expected in these artificial datasets, the ESRNA results are strikingly similar to the Wu data (pan cancer median ESRNA equals −0.041 in the Wu data vs. −0.046 and −0.043 in both in silico datasets, respectively; Supplementary Fig. S1A–S1C). This analysis provides clear evidence that the reported ESRNA results are not indicative of immune selection. We refer to Supplementary Fig. S2A–S2E and our previous work for more details on the role of hydrophobic amino acids in explaining the immunoediting-independent correlation between gene expression and HLA affinities (2, 4).The authors also report signals of the elimination phase of immunoediting, using another approach (ESCCF), based on different cancer cell fractions (CCF) between neoantigenic and nonantigenic mutations. Remarkably, these ESCCF signals are solely observed in cancer types with low median tumor mutational burdens (TMB; Supplementary Fig. S3A), which are also characterized by higher apparent proportions of neoantigenic mutations (Supplementary Fig. S3B). However, the authors prefiltered their data (only samples with minimally one neoantigenic mutation) and this affected cancer types with low TMBs much stronger than high TMBs (Supplementary Fig. S3C). Given the low average percentage of neoantigenic mutations in the prefiltered data (9.3%), it can be easily demonstrated that this filtering biases the distribution of these mutations in low TMB tumors toward higher numbers of neoantigenic mutations, exactly as we observed (Supplementary Fig. S3D). To provide conclusive evidence that this filtering indeed explains the reported signals, we randomly shuffled the neoantigen labeling before filtering and reanalyzed the data. Strikingly, the ESCCF signal and all observed TMB correlations in this completely random dataset were similar to what was interpreted by the authors as immunoediting (Supplementary Fig. S3E–S3H).Our analyses indicate that both the ESRNA and the ESCCF results are not related to immunoediting but rather caused by a gene expression and a filtering bias, respectively. We do not understand why the authors used different criteria to predict neoantigenic mutations in the ESRNA analysis (15.9% of all mutations) versus the ESCCF analysis (9.3% of all mutations), as the more stringent criteria in the latter analysis made it more prone for the described filtering bias. While, in theory, these metrics can detect immunoediting signals (as the authors correctly simulate), this will only be feasible when more accurate neoantigen prediction methods are available, considering more variables than just a peptide's HLA affinity.In our earlier work, we identified several other confounding factors that should be considered when drawing immunoediting conclusions from HLA affinity–derived neoantigen predictions (2). To demonstrate this, we defined a set of HLA-binding regions in a prototypical MHC class I genotype as part of our study. We regret the misinterpretation in the authors’ unpublished preprint from 2020, which they cite on several occasions. They state that “[our] method for neoantigen depletion signal detection is problematic, as the actual neoantigens with antigenicity are not located in [our] defined HLA-binding regions.” Obviously, our previous study does not provide a new neoantigen prediction method and lacks the same neoantigen prediction accuracy as any other HLA affinity–based approach, including the one in the Wu study. Rather than being the problem itself, our study pinpointed the many problems that occur when naïvely interpreting HLA affinity–based immune selection signals.In conclusion, while we do not doubt the concept of immunoediting as such, we believe that any analysis indicative of strong HLA affinity–based immune selection is questionable given the extremely low neoantigen prediction accuracies (Fig. 1). In our opinion, the Wu study is another illustration of the misinterpretation of HLA affinity signals in cancer genomics data and we strongly argue for more critical evaluations when interpreting these signals. The true extent of immunoediting signals in human cancer genomics data will only become apparent when more accurate and experimentally validated neoantigen prediction methods are available.See the Response, p. 973A. Claeys reports grants from Kom op tegen Kanker (Stand Up To Cancer) during the conduct of the study. No disclosures were reported by the other author.
更多查看译文
关键词
cancer evolution—letter,immunoediting,neoantigen-mediated
AI 理解论文
溯源树
样例
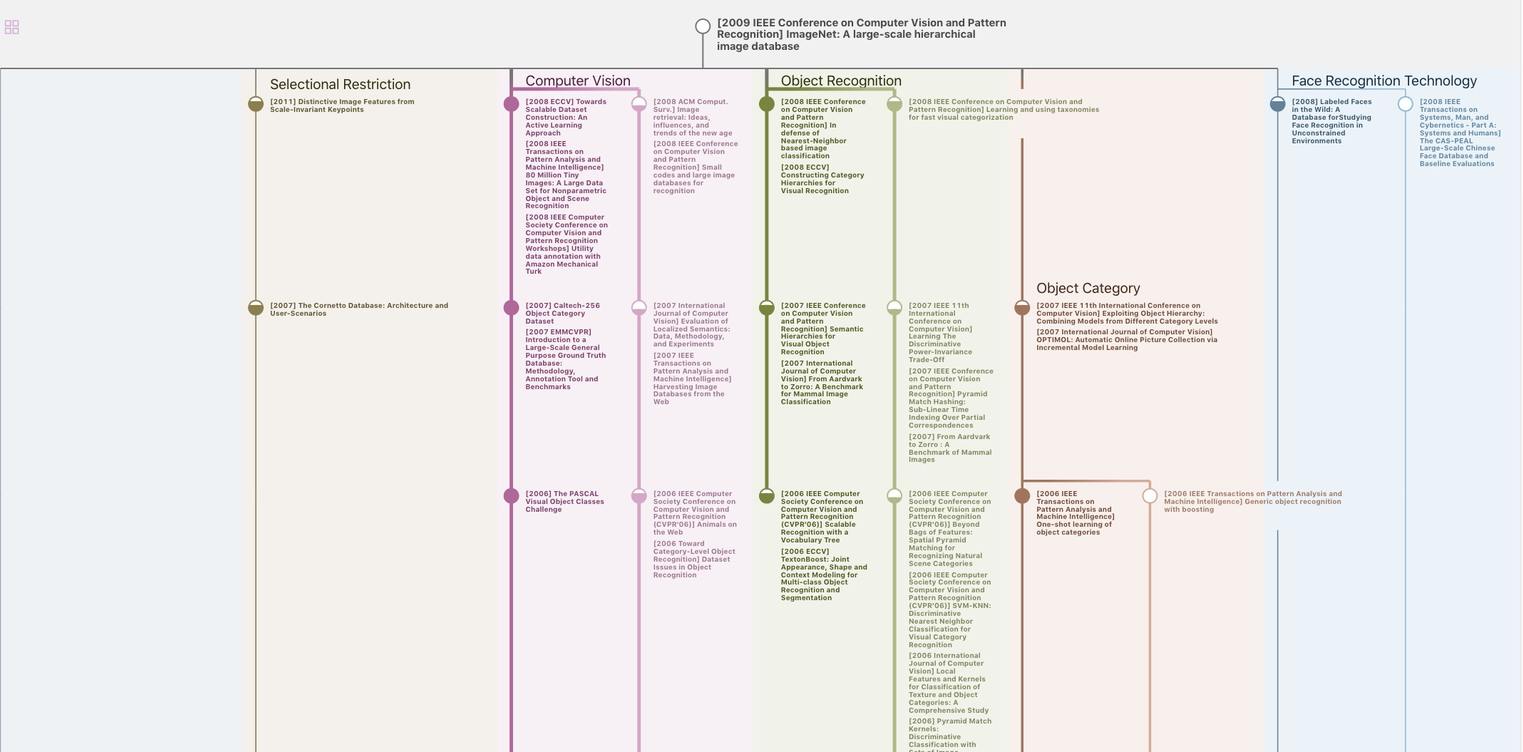
生成溯源树,研究论文发展脉络
Chat Paper
正在生成论文摘要