RUL prediction based on GAM–CNN for rotating machinery
Journal of the Brazilian Society of Mechanical Sciences and Engineering(2023)
摘要
To solve the problem of inadequate feature extraction and loss of key features in the process of remaining life prediction of bearings by traditional convolutional neural network (CNN), a CNN prediction model based on global attention mechanism (GAM) is proposed to achieve accurate remaining useful life (RUL) prediction of bearings. In this paper, a GAM–CNN prediction model for bearing RUL is proposed. First, the bearing’s one-dimensional (1D) vibration signal is transformed into two-dimensional (2D) image data that CNN is good at processing using continuous wavelet transform (CWT). Secondly, extract the time-domain degradation characteristics of bearings, select and build the health indicator (HI) of bearings using the monotonicity of the degradation characteristics, and introduce GAM into the CNN structure to adjust the contribution of key and unnecessary features to the bearing degradation process. GAM–CNN-based RUL prediction experiments were carried out with high-speed bearings of wind turbines and intelligent maintenance systems (IMS) experimental bearings, and the results show that GAM–CNN can effectively improve the prediction performance of the model. The results show that GAM–CNN has better prediction accuracy and generalization performance than other RUL prediction methods.
更多查看译文
关键词
Remaining useful life, Convolutional neural network, Global attention mechanism, Degenerative features, Continuous wavelet transform
AI 理解论文
溯源树
样例
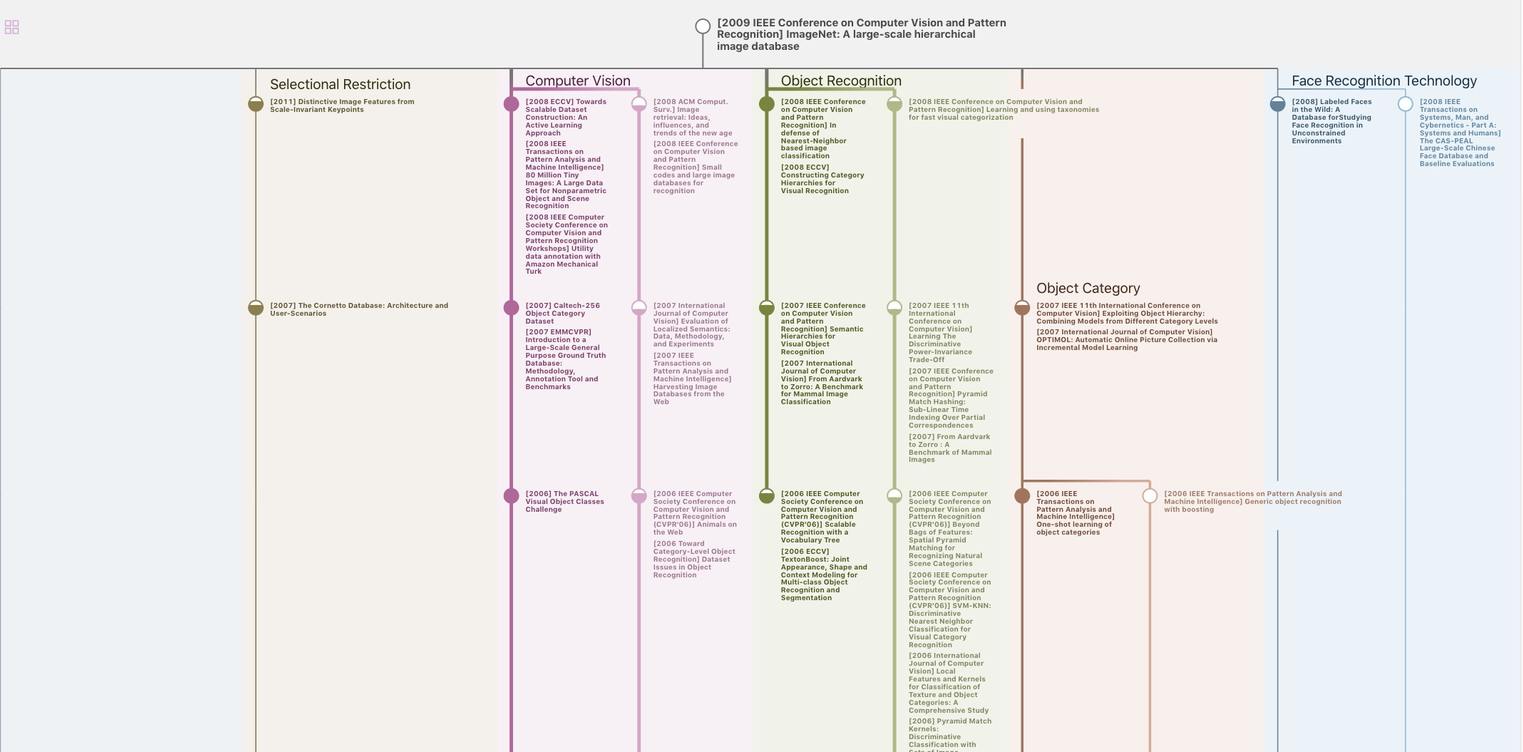
生成溯源树,研究论文发展脉络
Chat Paper
正在生成论文摘要