Efficiently Training Vision Transformers on Structural MRI Scans for Alzheimer's Disease Detection
2023 45TH ANNUAL INTERNATIONAL CONFERENCE OF THE IEEE ENGINEERING IN MEDICINE & BIOLOGY SOCIETY, EMBC(2023)
摘要
Neuroimaging of large populations is valuable to identify factors that promote or resist brain disease, and to assist diagnosis, subtyping, and prognosis. Data-driven models such as convolutional neural networks (CNNs) have increasingly been applied to brain images to perform diagnostic and prognostic tasks by learning robust features. Vision transformers (ViT) - a new class of deep learning architectures - have emerged in recent years as an alternative to CNNs for several computer vision applications. Here we tested variants of the ViT architecture for a range of desired neuroimaging downstream tasks based on difficulty, in this case for sex and Alzheimer's disease (AD) classification based on 3D brain MRI. In our experiments, two vision transformer architecture variants achieved an AUC of 0.987 for sex and 0.892 for AD classification, respectively. We independently evaluated our models on data from two benchmark AD datasets. We achieved a performance boost of 5% and 9-10% upon fine-tuning vision transformer models pre-trained on synthetic (generated by a latent diffusion model) and real MRI scans, respectively. Our main contributions include testing the effects of different ViT training strategies including pre-training, data augmentation and learning rate warm-ups followed by annealing, as pertaining to the neuroimaging domain. These techniques are essential for training ViT-like models for neuroimaging applications where training data is usually limited. We also analyzed the effect of the amount of training data utilized on the test-time performance of the ViT via data-model scaling curves.
更多查看译文
关键词
structural mri scans,alzheimer,vision transformers,disease detection
AI 理解论文
溯源树
样例
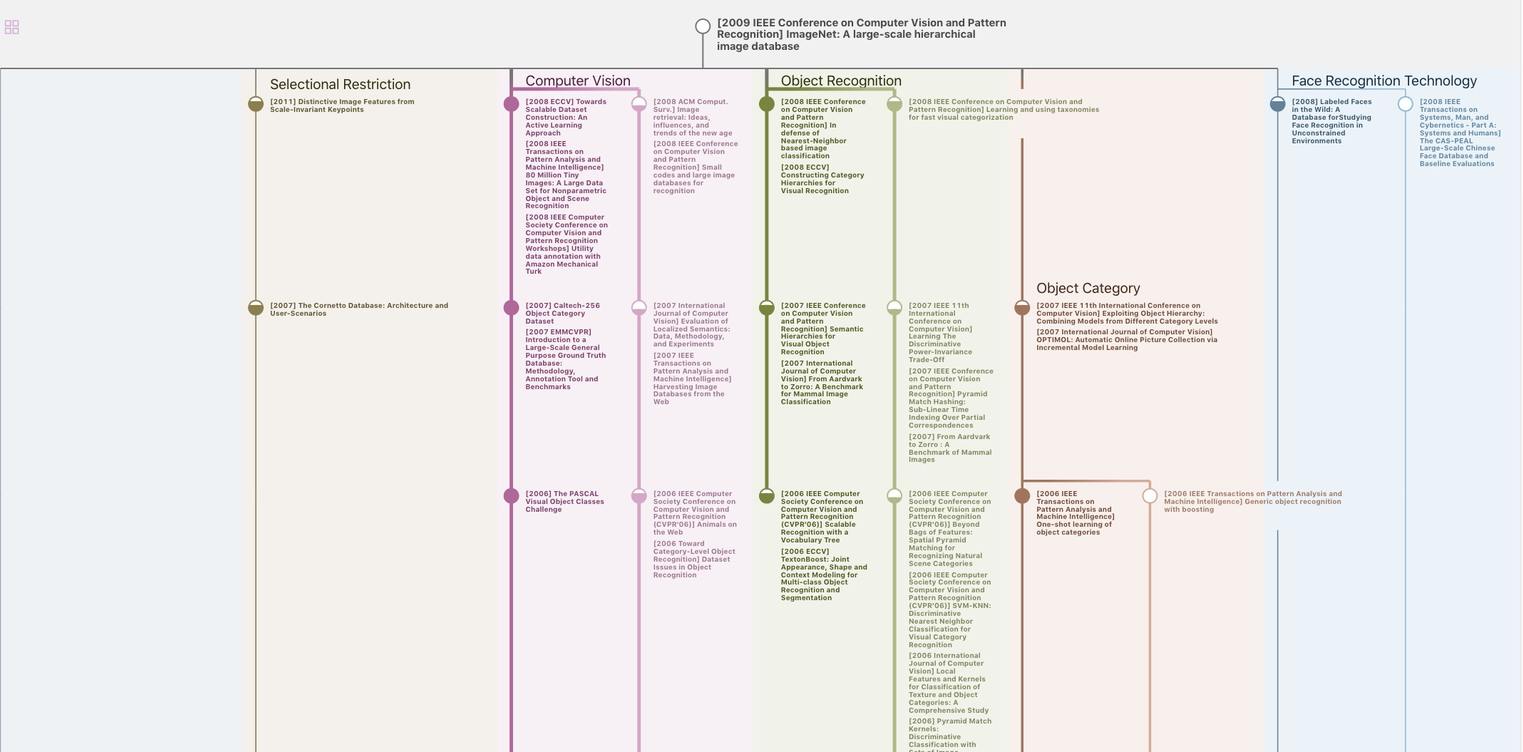
生成溯源树,研究论文发展脉络
Chat Paper
正在生成论文摘要