Fed-IoUT: Opportunities and Challenges of Federated Learning in the Internet of Underwater Things
IEEE Internet of Things Magazine(2023)
摘要
The Internet of Underwater Things (IoUT), as an emerging technology, plays an important role in the development of marine resources. With the growth of IoUT equipment, data from various sources has increased dramatically. Multiple challenges, such as data privacy, communication overhead, and data heterogeneity, arise with the application of new underwater Internet of Things (IoT) scenarios. To solve these challenges, we propose an IoUT framework called Fed-IoUT, which utilizes Federated Learning (FL) paradigm. FL, with its advantages of data privacy protection, low communication overhead, and balanced computing resources, is perfectly suited to our scenario. Fed-IoUT can make full use of the large amount of data collected by IoUT equipment, reduce data communication overhead, and protect the privacy of observing data. In this article, we also introduced the application of Fed-IoUT in relevant fields from five aspects: water quality monitoring, underwater life detection, flood forecasting, underwater detection, and marine energy transfer. And then, we put forward the limitations of development under the current conditions and made a comprehensive outlook for the future.
更多查看译文
关键词
federated learning,underwater things,internet,fed-iout
AI 理解论文
溯源树
样例
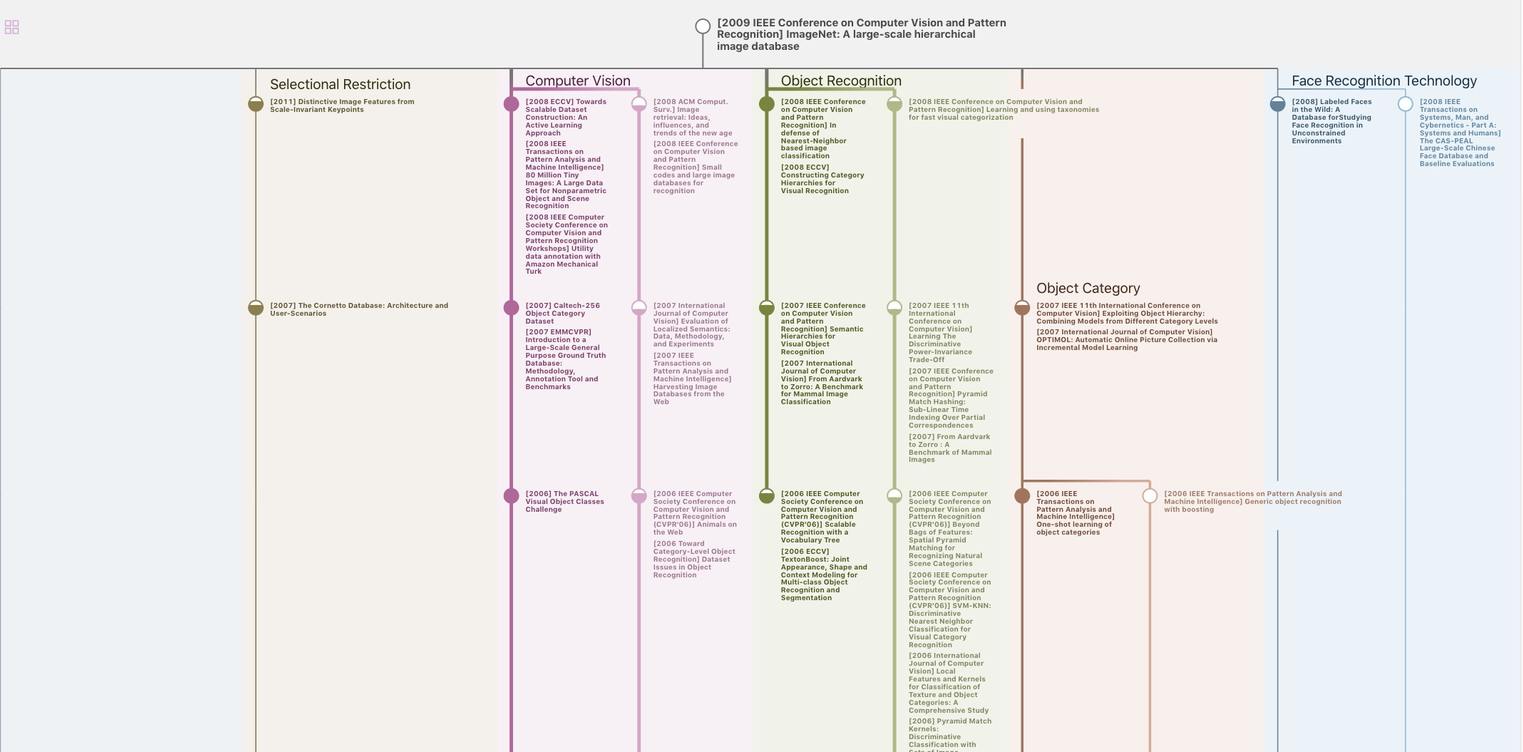
生成溯源树,研究论文发展脉络
Chat Paper
正在生成论文摘要