Semi-supervised Medical Image Segmentation with Low-Confidence Consistency and Class Separation
2023 International Conference on Intelligent Supercomputing and BioPharma (ISBP)(2023)
Abstract
Deep learning has achieved a great success in various fields, such as image classification, semantic segmentation and so on. But its excellent performance tends to rely on a large amount of data annotations that are hard to collect, especially in dense prediction tasks, like medical image segmentation. Semi-supervised learning (SSL), as a popular solution, relieves the burden of labeling. However, most of current semi-supervised medical image segmentation methods treat each pixel equally and underestimate the importance of indistinguishable and low-proportion pixels which are drowned in easily distinguishable but high-proportion pixels. We believe that these regions with less attention tend to contain crucial and indispensable information to obtain better segmentation performance. Therefore, we propose a simple but effective method for semi-supervised medical image segmentation task via enforcing low-confidence consistency and applying low-confidence class separation. Concretely, we separate low- and high-confidence pixels via the maximum probability values of model’s predictions and only low-confidence pixels are kept. For these remaining pixels, in the mean teacher framework, consistency is enforced for invariant predictions between student and teacher in the output level, and class separation is applied for promoting representations close to corresponding class prototypes in the feature level. We evaluated the proposed approach on two public datasets of cardiac, achieving a higher performance than the state-of-the-art semi-supervised methods on both datasets.
MoreTranslated text
Key words
Medical image segmentation,Semi-supervised learning,Low confidence,Consistency,Class separation
AI Read Science
Must-Reading Tree
Example
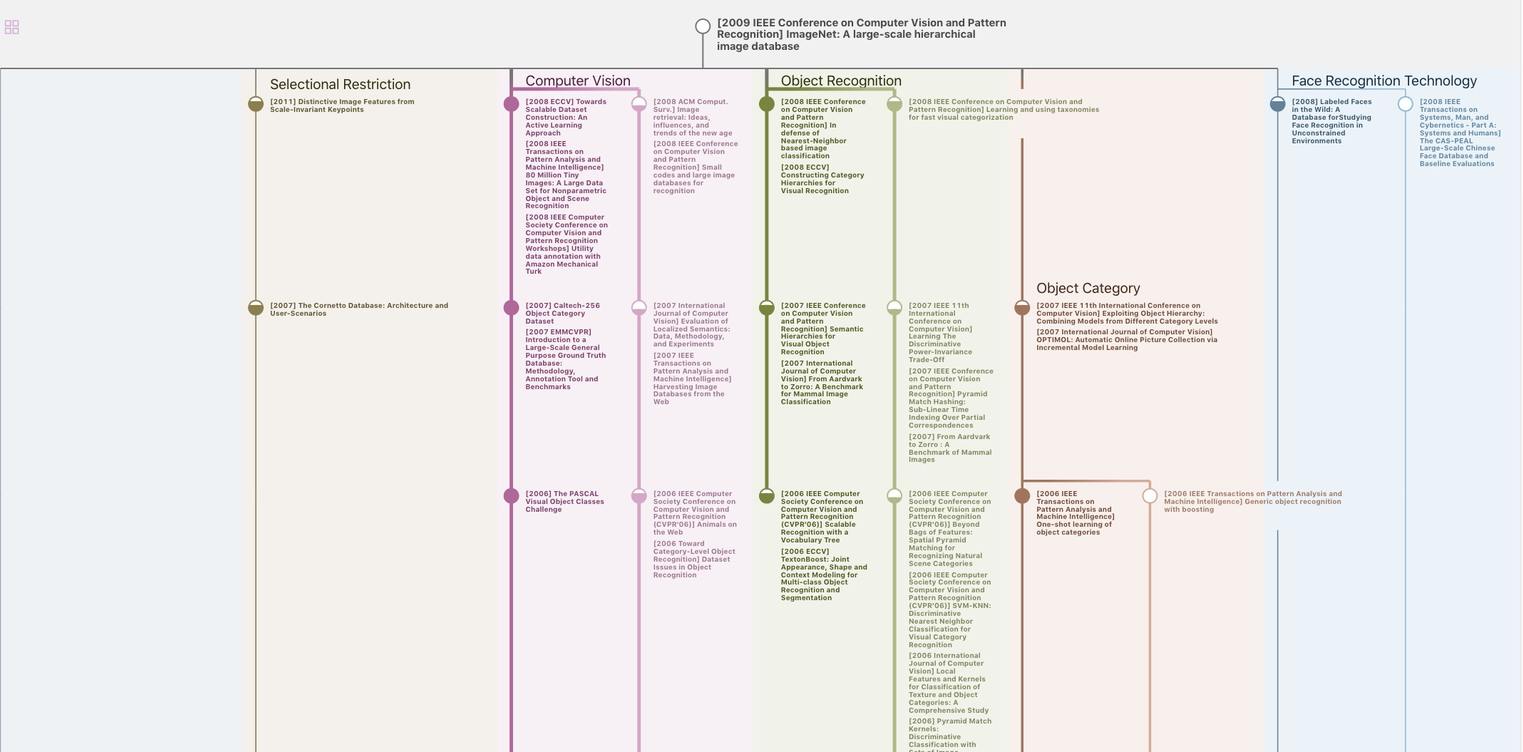
Generate MRT to find the research sequence of this paper
Chat Paper
Summary is being generated by the instructions you defined