A Novel Approach for Unsupervised Learning of Highly-Imbalanced Data
2022 IEEE 4th International Conference on Cognitive Machine Intelligence (CogMI)(2022)
摘要
Typical fraud datasets lack consistent and accurate labels and, as such, are typically highly imbalanced with non-fraud examples greatly outnumbering the fraudulent ones. This presents significant challenges to machine learning researchers and practitioners. Due to these challenges, an effective approach in identifying fraudulent data points needs to handle highly-imbalanced datasets and be robust to class labeling. This paper introduces a novel unsupervised procedure for learning from imbalanced datasets without class labels by iteratively cleaning the training dataset. Our methodology uses an autoencoder as an underlying learner. We describe its fraud detection performance and compare it to a baseline unsupervised fraud detection learner. Our results show that our procedure significantly outperforms the baseline, in both AUC and TPR, when testing on a publicly available highly-imbalanced credit card fraud detection dataset.
更多查看译文
关键词
Unsupervised Learning,Anomaly Detection,Autoencoder,high class imbalance
AI 理解论文
溯源树
样例
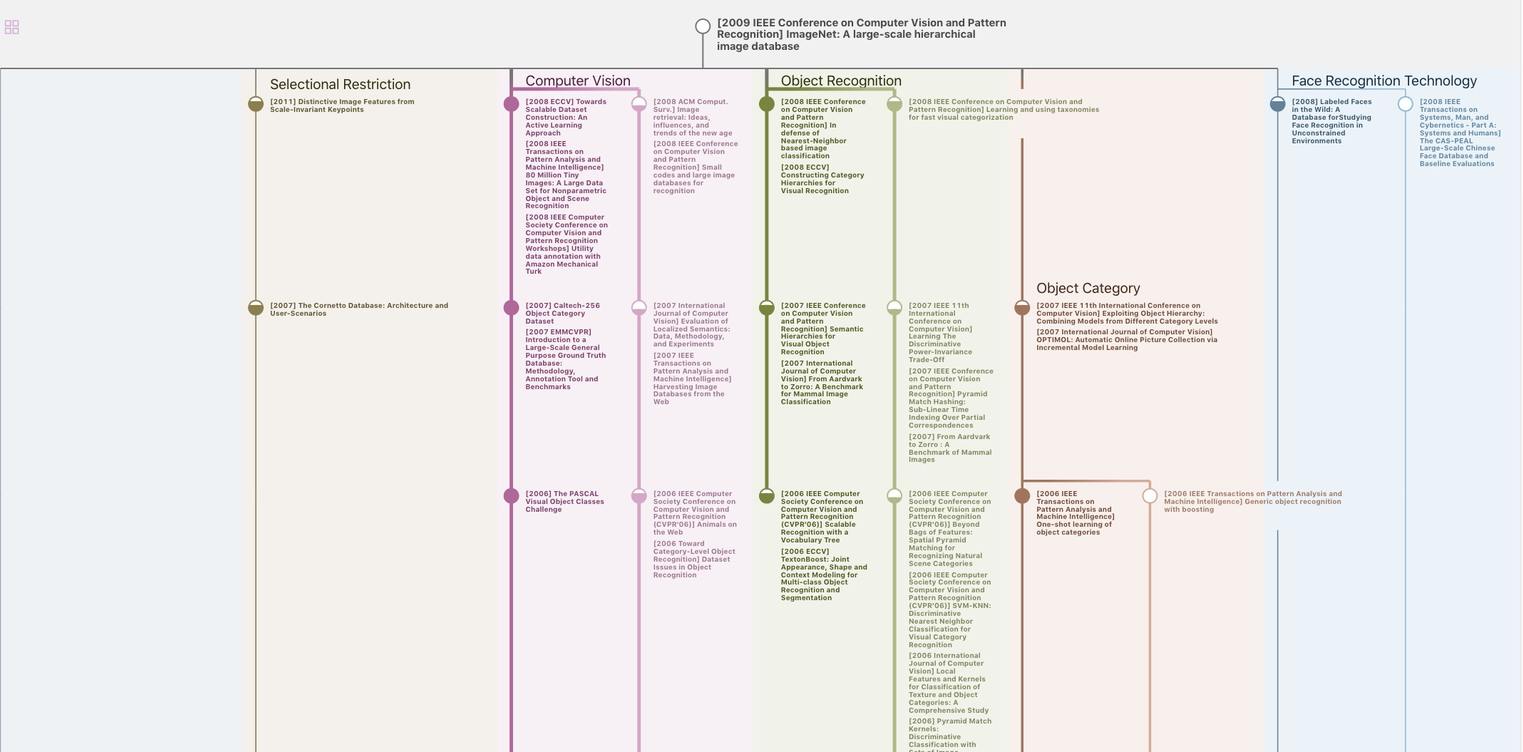
生成溯源树,研究论文发展脉络
Chat Paper
正在生成论文摘要