A Tensor Solution for Health Indicator Construction of Metro Wheelset Degradation with Irregular Noise
2022 International Conference on Sensing, Measurement & Data Analytics in the era of Artificial Intelligence (ICSMD)(2022)
摘要
During the operation of metro vehicles, wheel flange and wheel diameter continue to be abrased, so wheel degradation is inevitable. Influenced by the states of welded rail, subgrade and turnout, applied load, speed of running and other components, the degradation process of wheelset is vulnerable to irregular noise interference, which leads to abnormal fluctuation of vibration signal. As a result, the degradation process is hard to be accurately described. To address this problem, this paper proposes a new health indicator construction method for metro wheelset based on tensor reconstruction. First, tensor Tucker decomposition is utilized to obtain the core tensor of original signal, and then tensor reconstruction is applied to transform the signal into a new degradation sequence with noise reduction. Second, the Savitzky-Golay filter is employed to remove the irregular trend from the obtained degradation sequence. Finally, deep autoencoder network is used to extract deep degradation features, and after dimension reduction, a health indicator of wheelset degradation process can be obtained. The effectiveness of the proposed method is verified with Beijing subway wheelset degradation dataset in 2020. The results show that the constructed health indicator can accurately describe the whole degradation process with good trendability and monotonicity. More importantly, the proposed method possess good practicability since the key changing parts in the health indicator can correspond well to the actual maintenance records.
更多查看译文
关键词
Health indicator,Tucker decomposition,Deep autoencoder network,Irregular noise
AI 理解论文
溯源树
样例
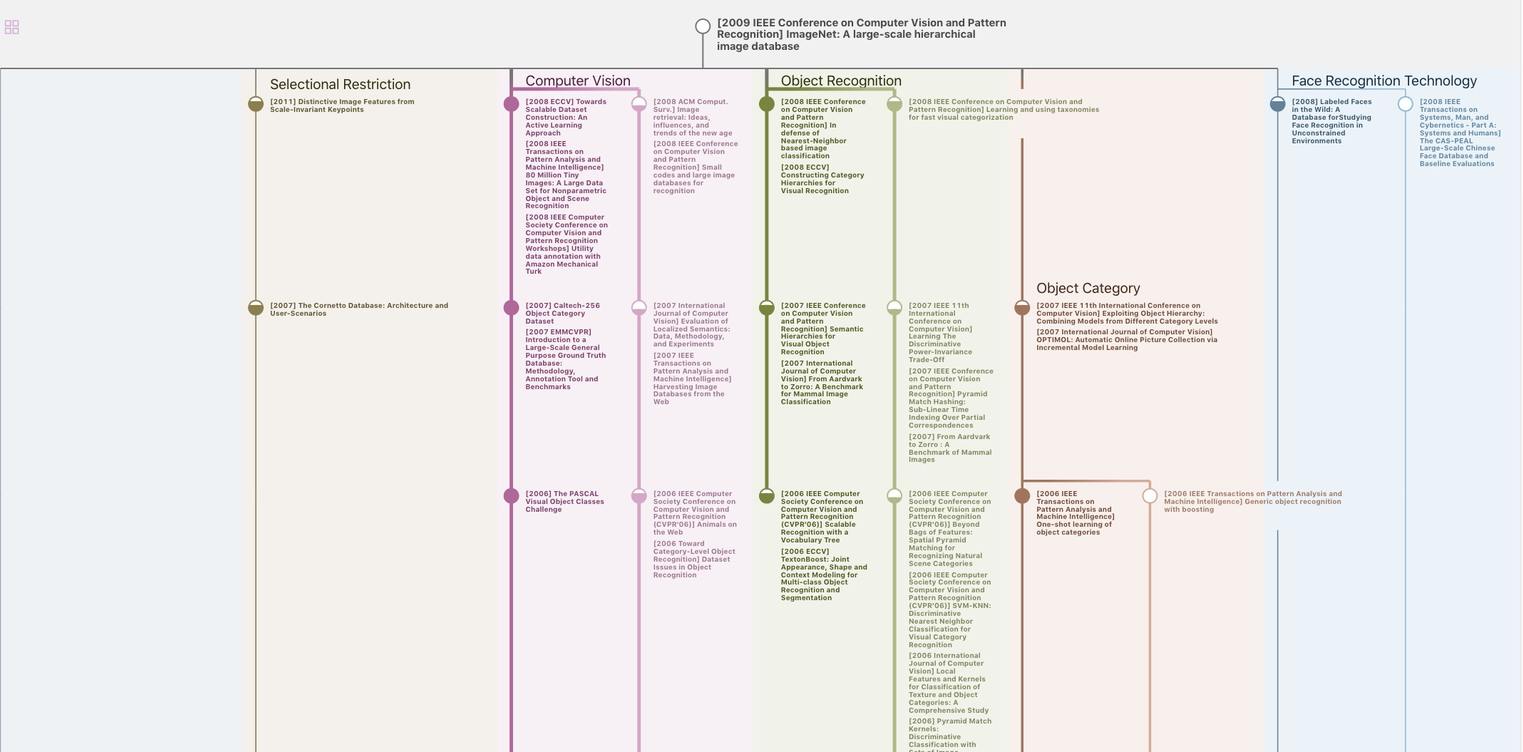
生成溯源树,研究论文发展脉络
Chat Paper
正在生成论文摘要