Remaining Useful Life Prediction of Aero-Engine Based on Transformer with Tendency Retainment
2022 International Conference on Sensing, Measurement & Data Analytics in the era of Artificial Intelligence (ICSMD)(2022)
摘要
One of the essential technologies for prognostics and health management of aero-engines is remaining useful life (RUL) prediction. Many deep learning models have recently been presented to extract features adaptively and forecast RUL end-to-end. However, it is still a challenging task to model data of long-life cycles and retain the degradation information when extracting features. To overcome the problem, we present a Transformer-based method with tendency retainment to predict RUL. Convolutional neural network is first used to fuse data from different sensors. Then, the long-life cycle data is encoded by Transformer encoder followed by long short-term memory neural network to extract features and finally RUL is predicted. Moreover, a tendency retainment module is designed based on contrastive learning to maintain the degradation information. The proposed method's performance is validated using NASA's C-MAPSS aero-engine dataset. The experimental results reveal that the proposed method outperforms other state-of-the-art methods in terms of prediction accuracy.
更多查看译文
关键词
Remaining useful life,Aero-engine,Transformer,Tendency retainment,Contrastive learning
AI 理解论文
溯源树
样例
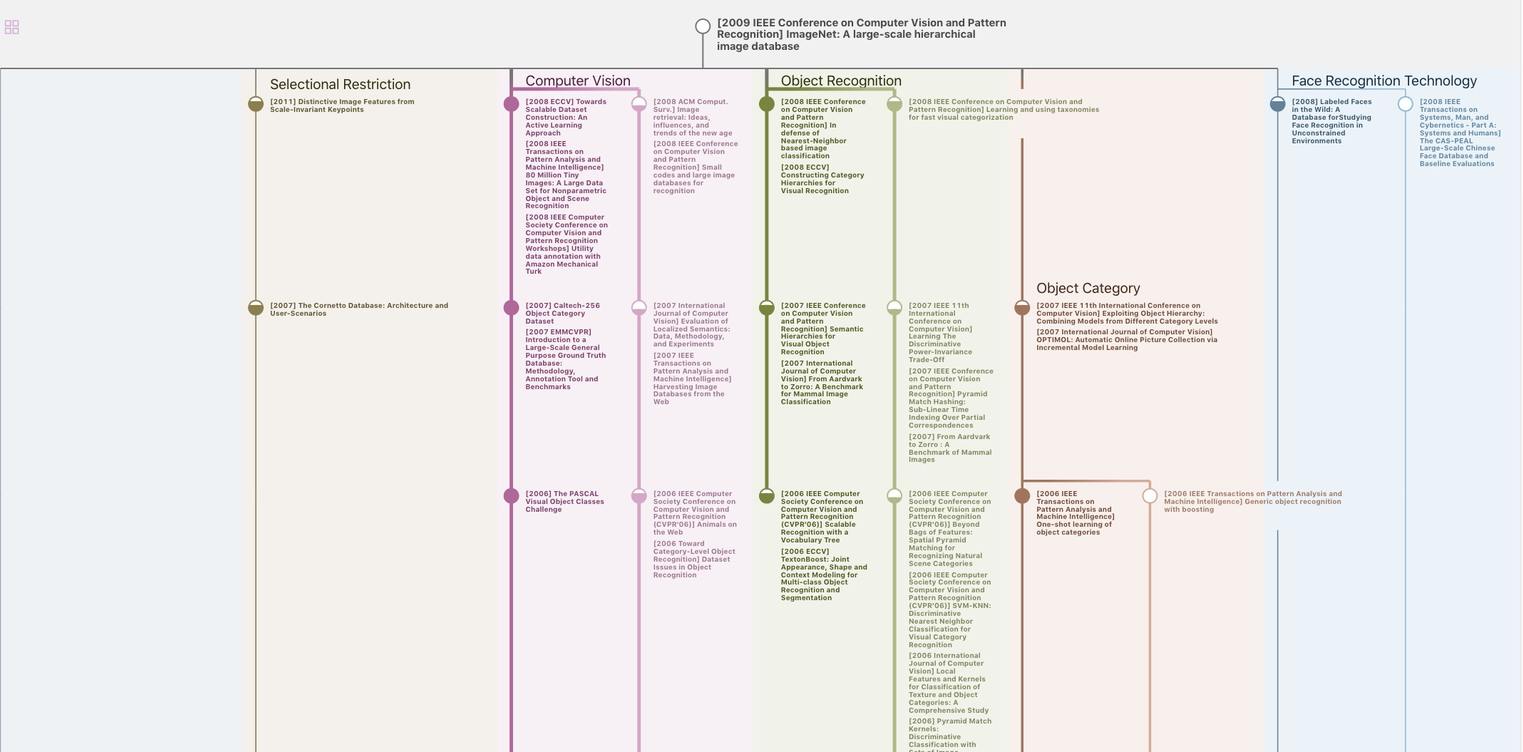
生成溯源树,研究论文发展脉络
Chat Paper
正在生成论文摘要