Interlacing Polynomial Method for the Column Subset Selection Problem
INTERNATIONAL MATHEMATICS RESEARCH NOTICES(2024)
摘要
This paper investigates the spectral norm version of the column subset selection problem. Given a matrix $\textbf{A}\in \mathbb{R}<^>{n\times d}$ and a positive integer $k\leq \textrm{rank}(\textbf{A})$, the objective is to select exactly $k$ columns of $\textbf{A}$ that minimize the spectral norm of the residual matrix after projecting $\textbf{A}$ onto the space spanned by the selected columns. We use the method of interlacing polynomials introduced by Marcus-Spielman-Srivastava to derive a new upper bound on the minimal approximation error. This new bound is asymptotically sharp when the matrix $\textbf{A}\in \mathbb{R}<^>{n\times d}$ obeys a spectral power-law decay. The relevant expected characteristic polynomial is a variation of the expected polynomial for the restricted invertibility problem, incorporating two extra variable substitution operators. Finally, we propose a deterministic polynomial-time algorithm that achieves this error bound up to a computational error.
更多查看译文
AI 理解论文
溯源树
样例
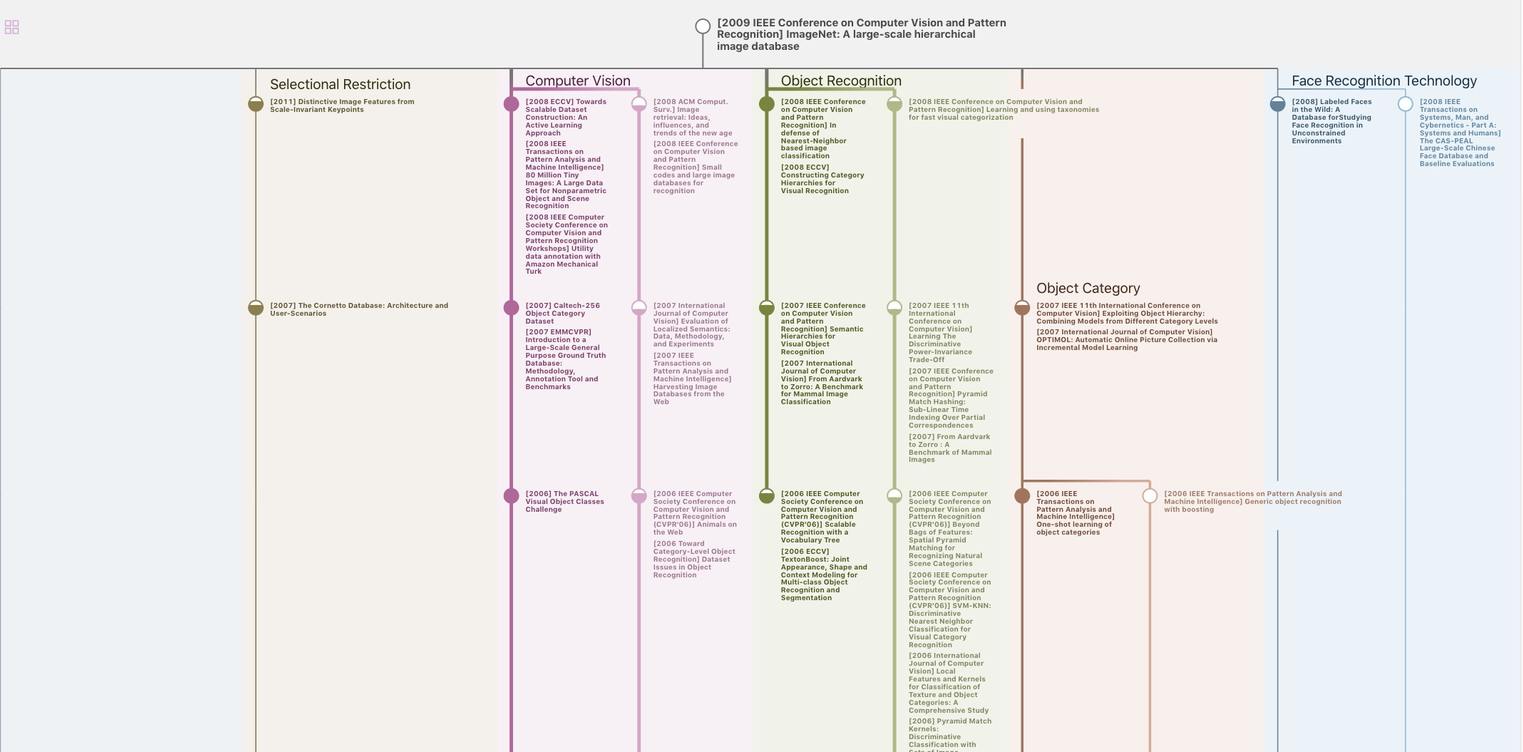
生成溯源树,研究论文发展脉络
Chat Paper
正在生成论文摘要