Learning Adaptable Risk-Sensitive Policies to Coordinate in Multi-agent General-Sum Games
NEURAL INFORMATION PROCESSING, ICONIP 2023, PT I(2024)
Abstract
In general-sum games, the interaction of self-interested learning agents commonly leads to socially worse outcomes, such as defect-defect in the iterated stag hunt (ISH). Previous works address this challenge by sharing rewards or shaping their opponents' learning process, which require too strong assumptions. In this paper, we observe that agents trained to optimize expected returns are more likely to choose a safe action that leads to guaranteed but lower rewards. To overcome this, we present Adaptable Risk-Sensitive Policy (ARSP). ARSP learns the distributions over agent's return and estimates a dynamic risk-seeking bonus to discover risky coordination strategies. Furthermore, to avoid overfitting training opponents, ARSP learns an auxiliary opponent modeling task to infer opponents' types and dynamically alter corresponding strategies during execution. Extensive experiments show that ARSP agents can achieve stable coordination during training and adapt to non-cooperative opponents during execution, outperforming a set of baselines by a large margin.
MoreTranslated text
Key words
Distributional Reinforcement Learning,Policy Adaptation,General-Sum Games,Multi-Agent Systems
AI Read Science
Must-Reading Tree
Example
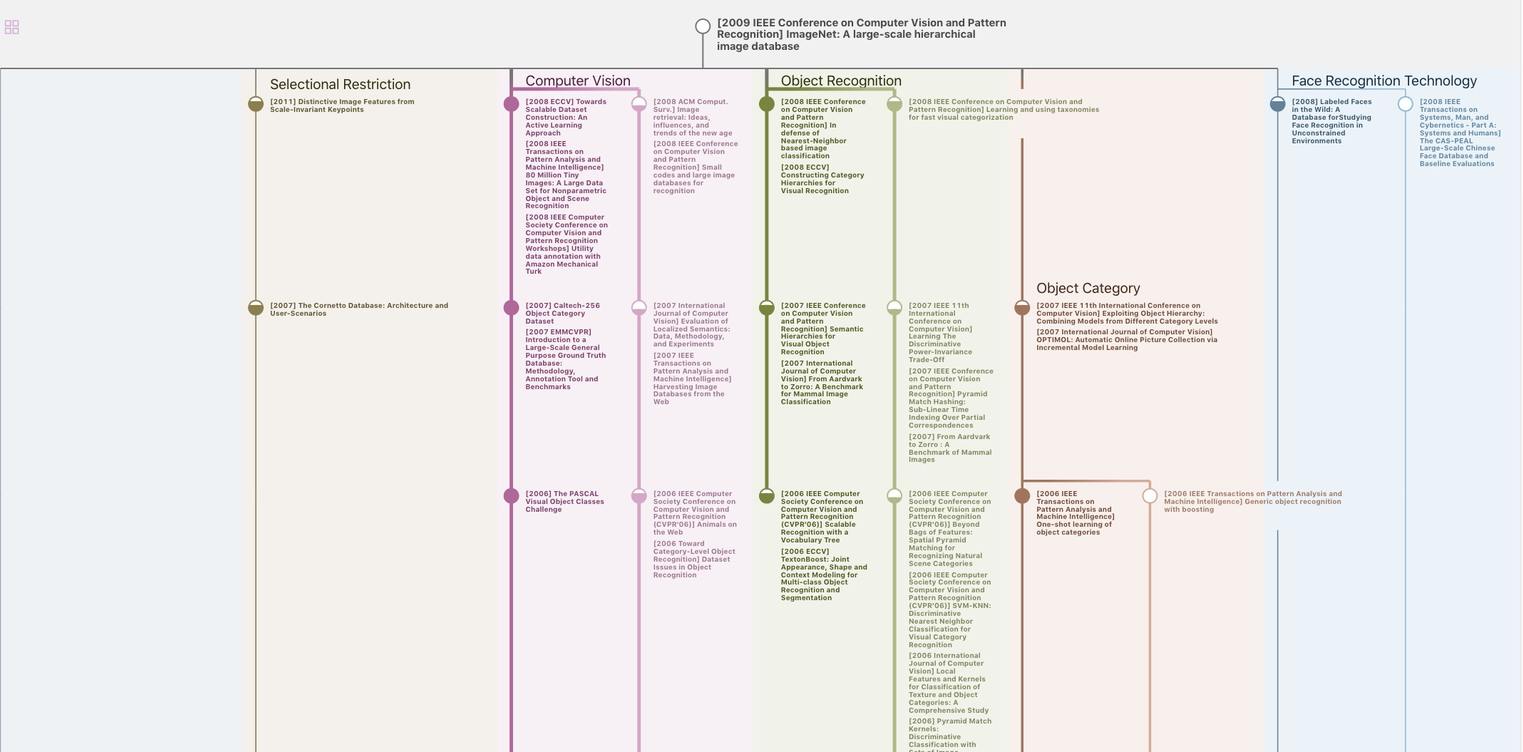
Generate MRT to find the research sequence of this paper
Chat Paper
Summary is being generated by the instructions you defined