Do More with What You Have: Transferring Depth-Scale from Labeled to Unlabeled Domains
arxiv
Abstract
Transferring the absolute depth prediction capabilities of an estimator to anew domain is a task with significant real-world applications. This task isspecifically challenging when images from the new domain are collected withoutground-truth depth measurements, and possibly with sensors of differentintrinsics. To overcome such limitations, a recent zero-shot solution wastrained on an extensive training dataset and encoded the various cameraintrinsics. Other solutions generated synthetic data with depth labels thatmatched the intrinsics of the new target data to enable depth-scale transferbetween the domains. In this work we present an alternative solution that can utilize any existingsynthetic or real dataset, that has a small number of images annotated withground truth depth labels. Specifically, we show that self-supervised depthestimators result in up-to-scale predictions that are linearly correlated totheir absolute depth values across the domain, a property that we model in thiswork using a single scalar. In addition, aligning the field-of-view of twodatasets prior to training, results in a common linear relationship for bothdomains. We use this observed property to transfer the depth-scale from sourcedatasets that have absolute depth labels to new target datasets that lack thesemeasurements, enabling absolute depth predictions in the target domain. The suggested method was successfully demonstrated on the KITTI, DDAD andnuScenes datasets, while using other existing real or synthetic sourcedatasets, that have a different field-of-view, other image style or structuralcontent, achieving comparable or better accuracy than other existing methodsthat do not use target ground-truth depths.
MoreTranslated text
AI Read Science
Must-Reading Tree
Example
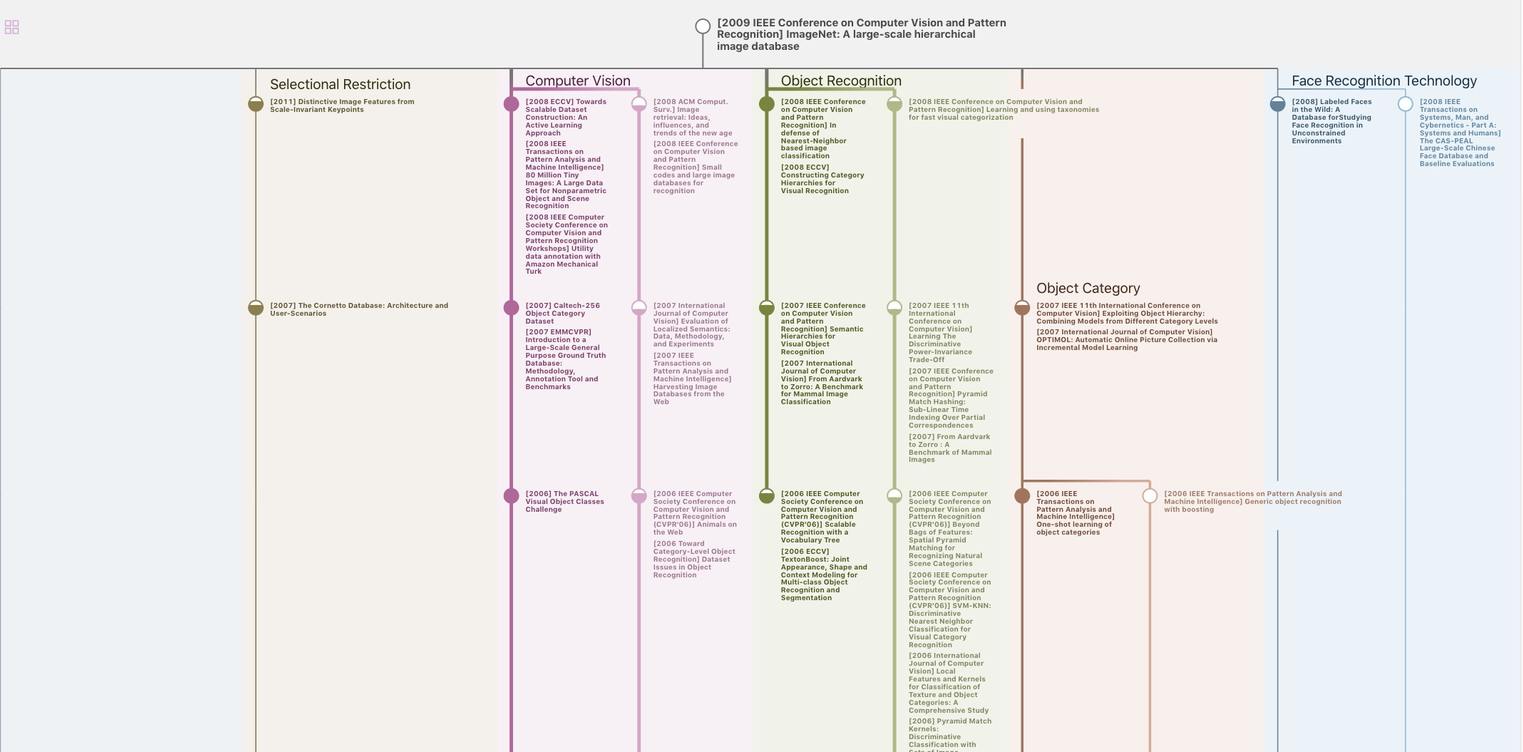
Generate MRT to find the research sequence of this paper
Chat Paper
Summary is being generated by the instructions you defined