Cross-lingual Alzheimer's Disease detection based on paralinguistic and pre-trained features
CoRR(2023)
Abstract
We present our submission to the ICASSP-SPGC-2023 ADReSS-M Challenge Task, which aims to investigate which acoustic features can be generalized and transferred across languages for Alzheimer's Disease (AD) prediction. The challenge consists of two tasks: one is to classify the speech of AD patients and healthy individuals, and the other is to infer Mini Mental State Examination (MMSE) score based on speech only. The difficulty is mainly embodied in the mismatch of the dataset, in which the training set is in English while the test set is in Greek. We extract paralinguistic features using openSmile toolkit and acoustic features using XLSR-53. In addition, we extract linguistic features after transcribing the speech into text. These features are used as indicators for AD detection in our method. Our method achieves an accuracy of 69.6% on the classification task and a root mean squared error (RMSE) of 4.788 on the regression task. The results show that our proposed method is expected to achieve automatic multilingual Alzheimer's Disease detection through spontaneous speech.
MoreTranslated text
Key words
Alzheimer’s dementia detection,paralinguistic features,pre-trained features
AI Read Science
Must-Reading Tree
Example
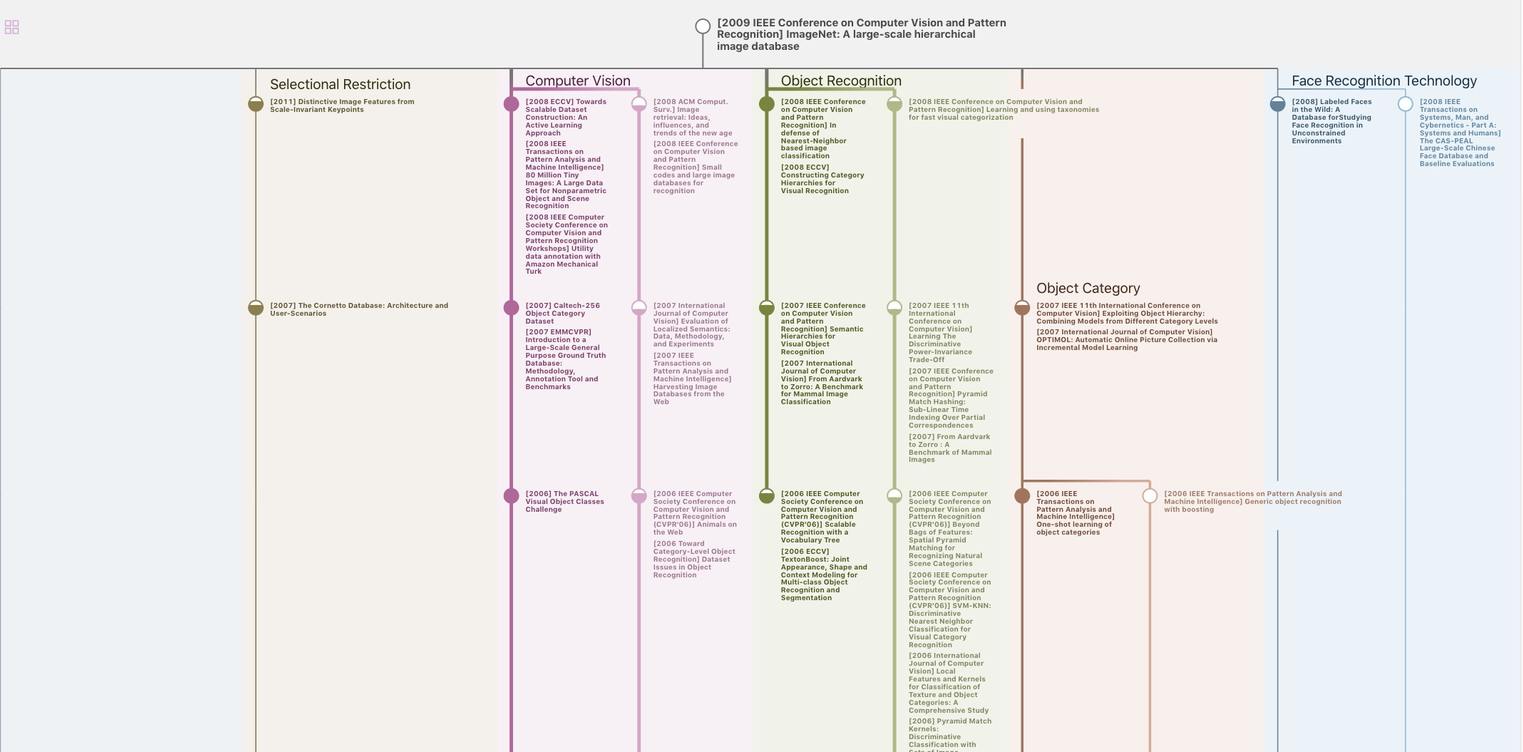
Generate MRT to find the research sequence of this paper
Chat Paper
Summary is being generated by the instructions you defined