Recent Advances and Applications of Machine Learning in Experimental Solid Mechanics: A Review
APPLIED MECHANICS REVIEWS(2023)
摘要
For many decades, experimental solid mechanics has played a crucial role in characterizing and understanding the mechanical properties of natural and novel artificial materials. Recent advances in machine learning (ML) provide new opportunities for the field, including experimental design, data analysis, uncertainty quantification, and inverse problems. As the number of papers published in recent years in this emerging field is growing exponentially, it is timely to conduct a comprehensive and up-to-date review of recent ML applications in experimental solid mechanics. Here, we first provide an overview of common ML algorithms and terminologies that are pertinent to this review, with emphasis placed on physics-informed and physics-based ML methods. Then, we provide thorough coverage of recent ML applications in traditional and emerging areas of experimental mechanics, including fracture mechanics, biomechanics, nano- and micromechanics, architected materials, and two-dimensional materials. Finally, we highlight some current challenges of applying ML to multimodality and multifidelity experimental datasets, quantifying the uncertainty of ML predictions, and proposing several future research directions. This review aims to provide valuable insights into the use of ML methods and a variety of examples for researchers in solid mechanics to integrate into their experiments.
更多查看译文
关键词
experimental solid mechanics,machine learning,fracture mechanics,biomechanics,architected materials,nanoindentation,2D materials,physics-informed neural networks (PINNs),neural operator
AI 理解论文
溯源树
样例
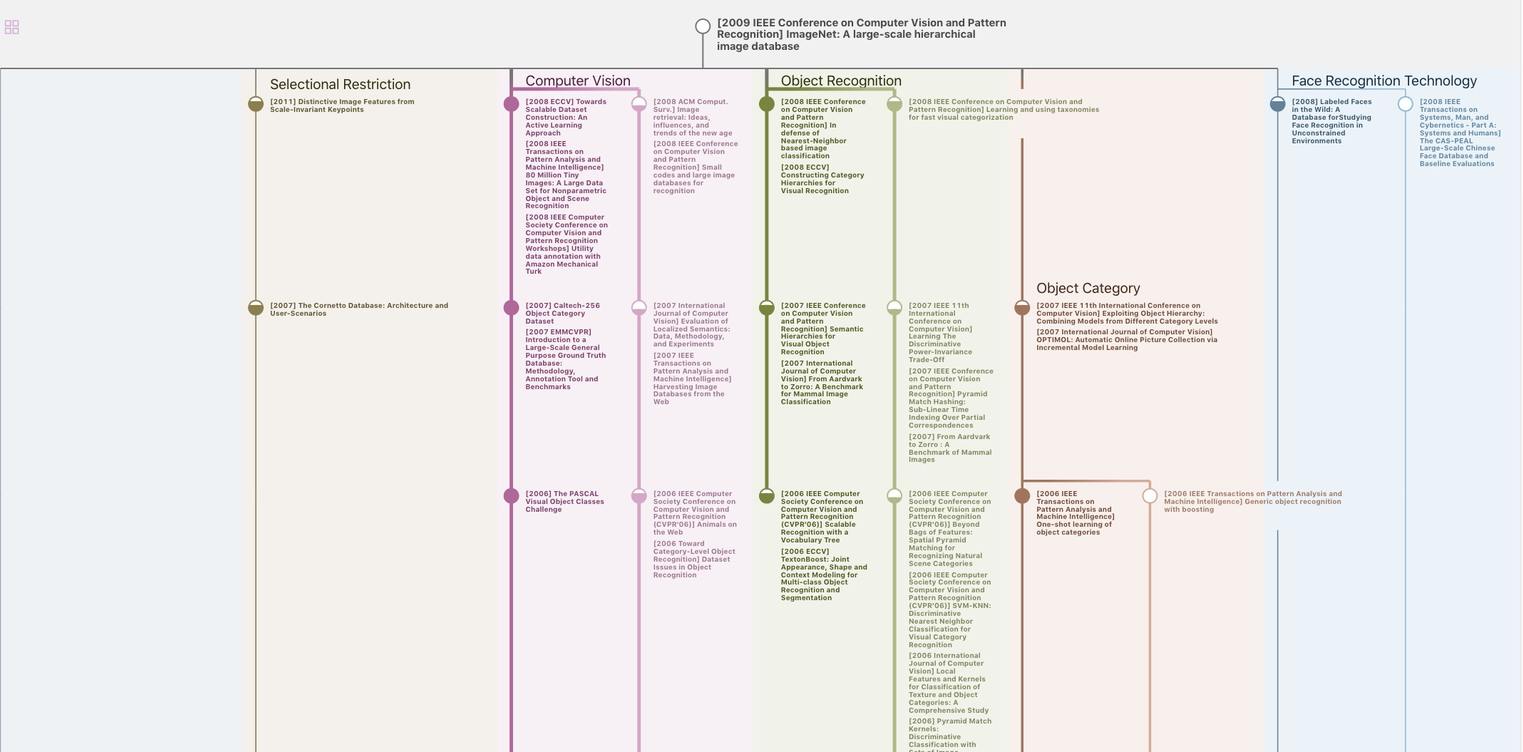
生成溯源树,研究论文发展脉络
Chat Paper
正在生成论文摘要