Fast exploration and learning of latent graphs with aliased observations
arxiv(2023)
摘要
Consider this scenario: an agent navigates a latent graph by performing actions that take it from one node to another. The chosen action determines the probability distribution over the next visited node. At each node, the agent receives an observation, but this observation is not unique, so it does not identify the node, making the problem aliased. The purpose of this work is to provide a policy that approximately maximizes exploration efficiency (i.e., how well the graph is recovered for a given exploration budget). In the unaliased case, we show improved performance w.r.t. state-of-the-art reinforcement learning baselines. For the aliased case we are not aware of suitable baselines and instead show faster recovery w.r.t. a random policy for a wide variety of topologies, and exponentially faster recovery than a random policy for challenging topologies. We dub the algorithm eFeX (from eFficient eXploration).
更多查看译文
AI 理解论文
溯源树
样例
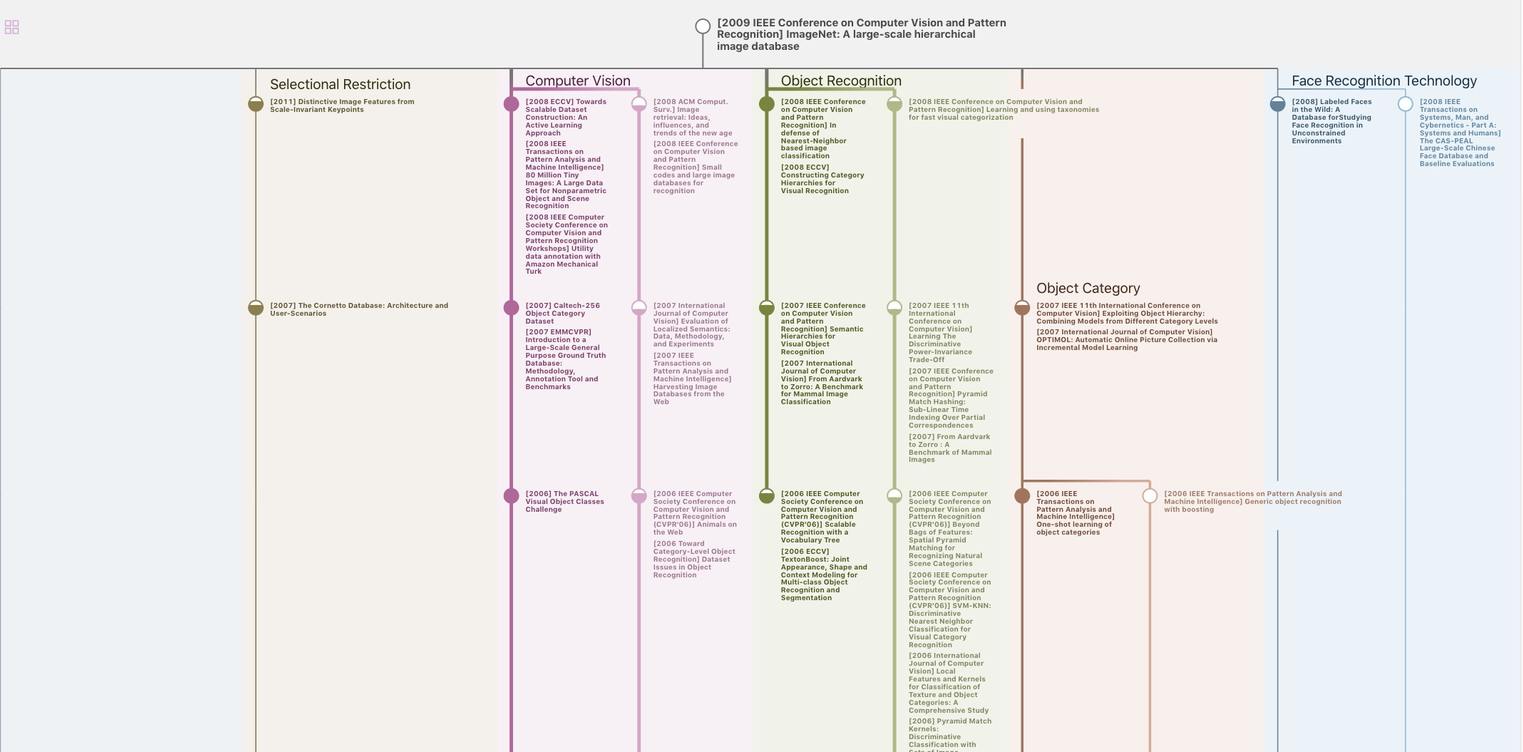
生成溯源树,研究论文发展脉络
Chat Paper
正在生成论文摘要