Distributed Non-Bayesian Learning for Games with Incomplete Information
arxiv(2023)
摘要
We consider distributed learning problem in games with an unknown cost-relevant parameter, and aim to find the Nash equilibrium while learning the true parameter. Inspired by the social learning literature, we propose a distributed non-Bayesian rule to learn the parameter (each agent maintains a belief on the parameter and updates the belief according to the received noisy cost), combined with best response dynamics for strategy update. The difficulty of the analysis lies mainly in the fact that the parameter learning process and strategy update process are coupled. We first prove that agents' beliefs converge to a common belief and the strategy profiles converge to a Nash equilibrium under this common belief. On this basis, we further show that the beliefs eventually concentrate on the true parameter.
更多查看译文
AI 理解论文
溯源树
样例
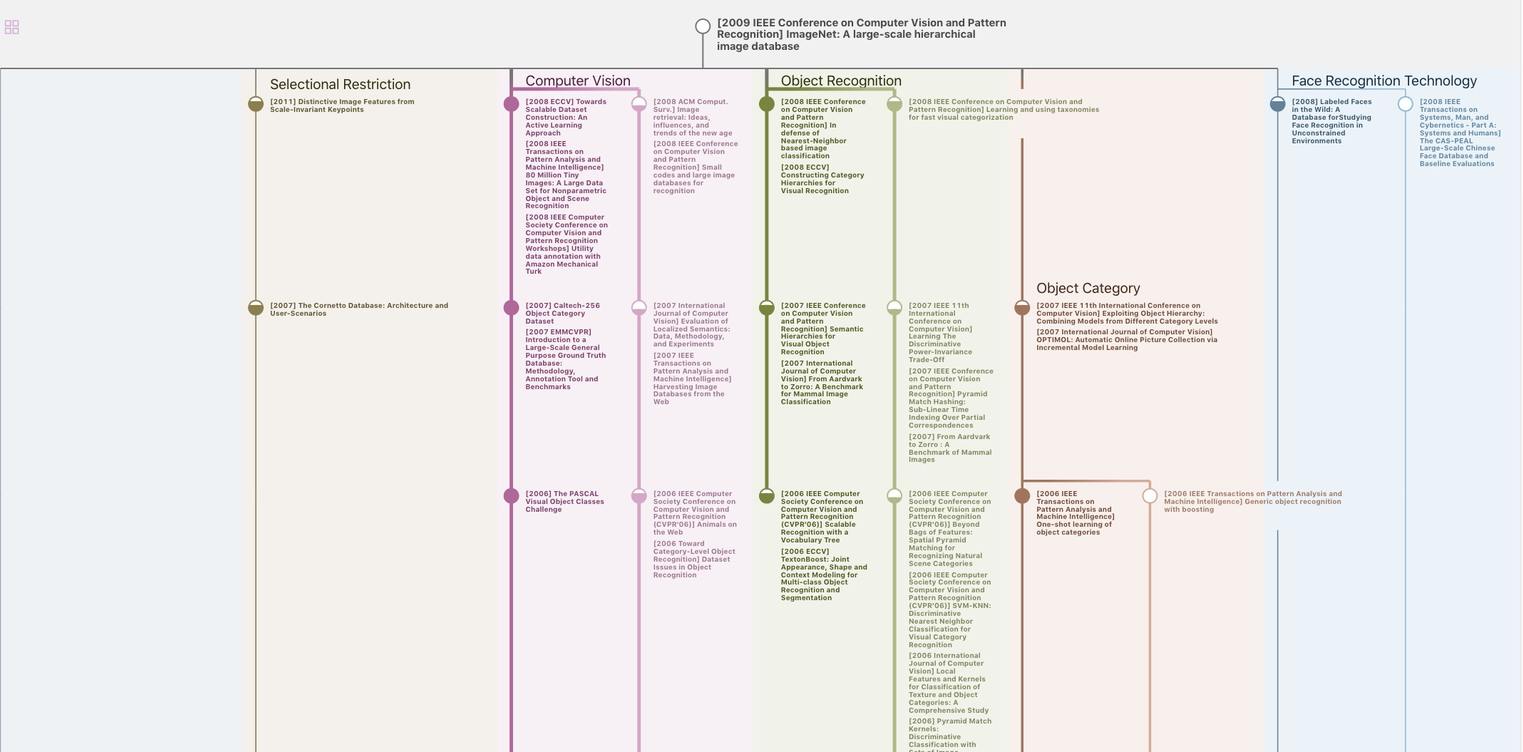
生成溯源树,研究论文发展脉络
Chat Paper
正在生成论文摘要