The challenge of representation learning: Improved accuracy in deep vision models does not come with better predictions of perceptual similarity
arxiv(2023)
摘要
Over the last years, advancements in deep learning models for computer vision have led to a dramatic improvement in their image classification accuracy. However, models with a higher accuracy in the task they were trained on do not necessarily develop better image representations that allow them to also perform better in other tasks they were not trained on. In order to investigate the representation learning capabilities of prominent high-performing computer vision models, we investigated how well they capture various indices of perceptual similarity from large-scale behavioral datasets. We find that higher image classification accuracy rates are not associated with a better performance on these datasets, and in fact we observe no improvement in performance since GoogLeNet (released 2015) and VGG-M (released 2014). We speculate that more accurate classification may result from hyper-engineering towards very fine-grained distinctions between highly similar classes, which does not incentivize the models to capture overall perceptual similarities.
更多查看译文
关键词
representation learning,vision models,improved accuracy
AI 理解论文
溯源树
样例
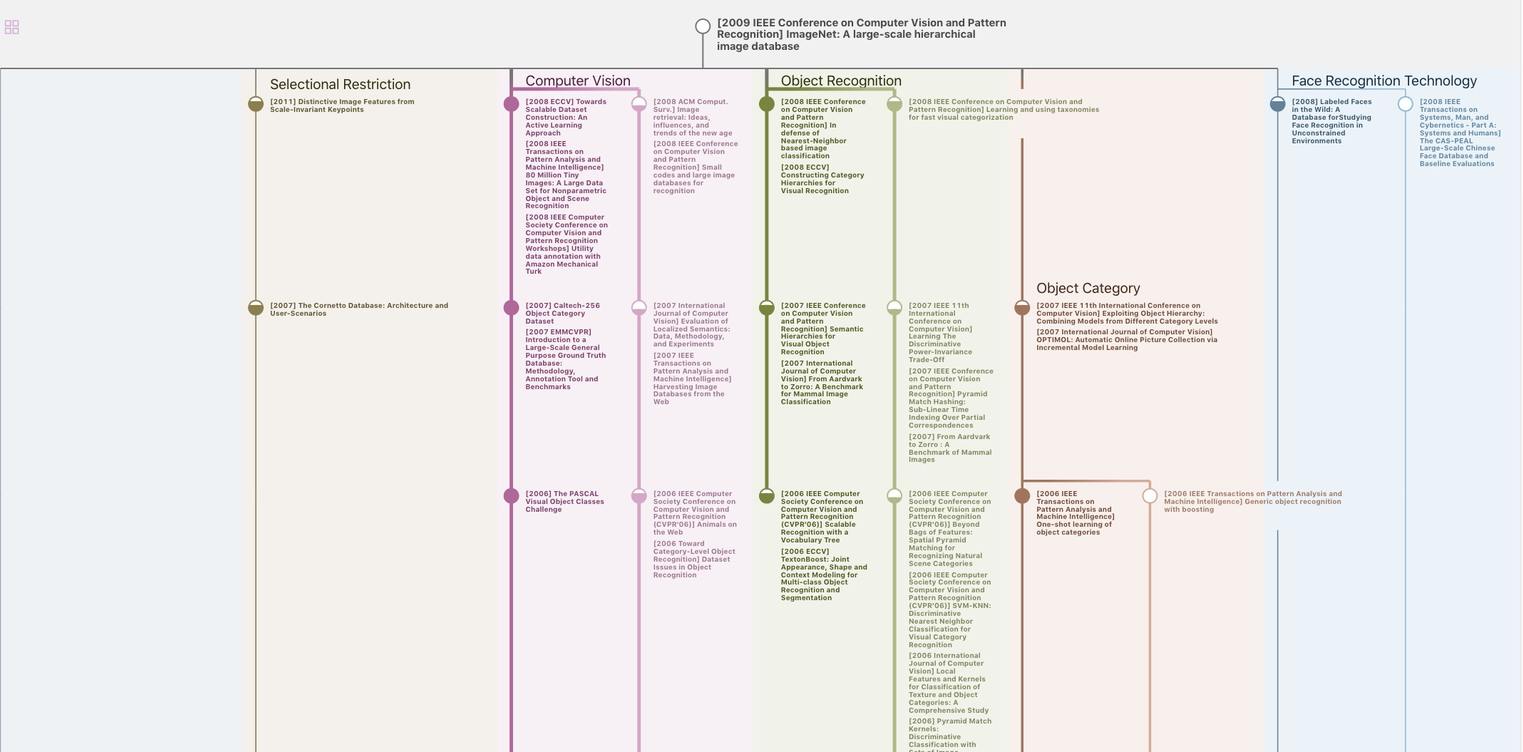
生成溯源树,研究论文发展脉络
Chat Paper
正在生成论文摘要