Unifying Grokking and Double Descent
arxiv(2023)
摘要
A principled understanding of generalization in deep learning may require unifying disparate observations under a single conceptual framework. Previous work has studied \emph{grokking}, a training dynamic in which a sustained period of near-perfect training performance and near-chance test performance is eventually followed by generalization, as well as the superficially similar \emph{double descent}. These topics have so far been studied in isolation. We hypothesize that grokking and double descent can be understood as instances of the same learning dynamics within a framework of pattern learning speeds. We propose that this framework also applies when varying model capacity instead of optimization steps, and provide the first demonstration of model-wise grokking.
更多查看译文
关键词
double descent
AI 理解论文
溯源树
样例
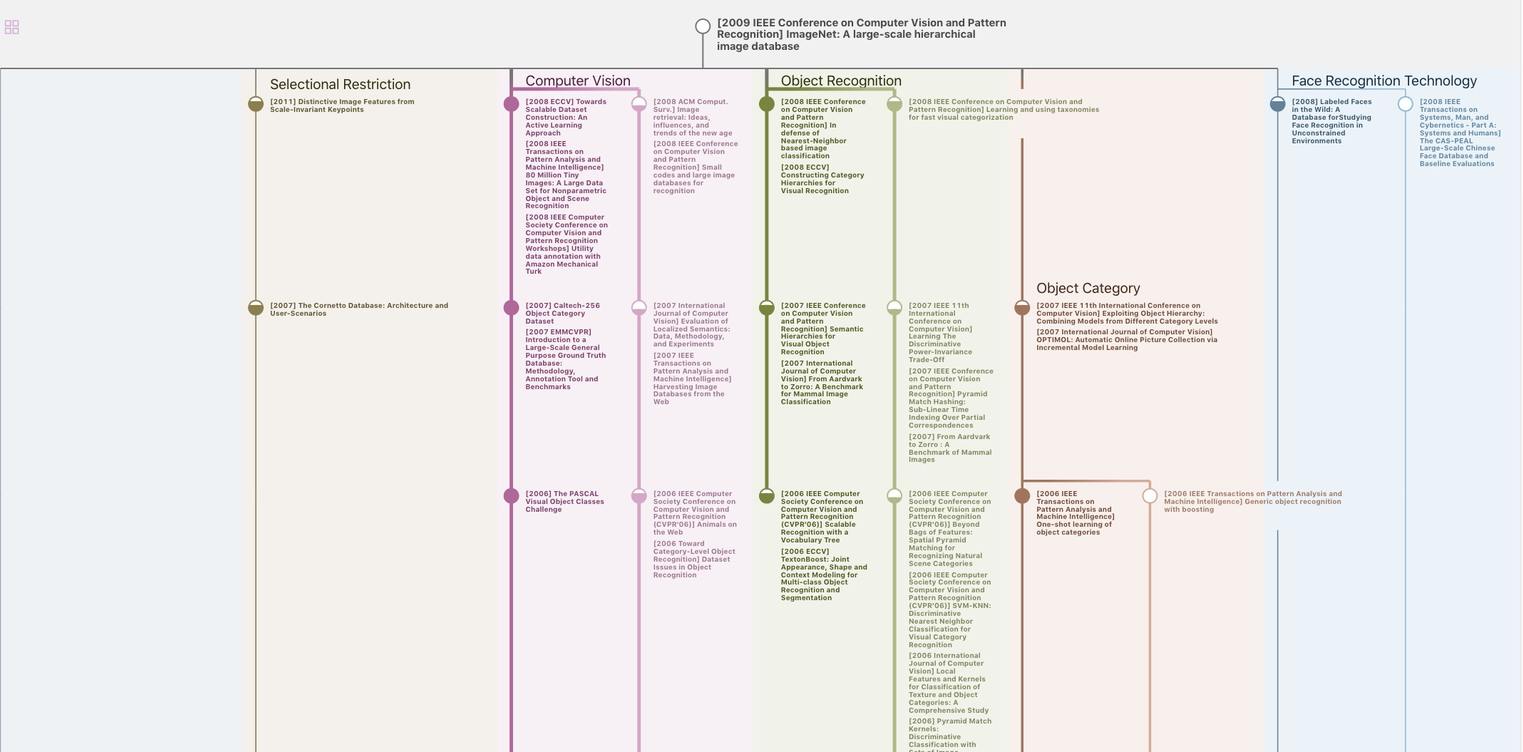
生成溯源树,研究论文发展脉络
Chat Paper
正在生成论文摘要