A General Recipe for the Analysis of Randomized Multi-Armed Bandit Algorithms
arxiv(2023)
摘要
In this paper we propose a general methodology to derive regret bounds for randomized multi-armed bandit algorithms. It consists in checking a set of sufficient conditions on the sampling probability of each arm and on the family of distributions to prove a logarithmic regret. As a direct application we revisit two famous bandit algorithms, Minimum Empirical Divergence (MED) and Thompson Sampling (TS), under various models for the distributions including single parameter exponential families, Gaussian distributions, bounded distributions, or distributions satisfying some conditions on their moments. In particular, we prove that MED is asymptotically optimal for all these models, but also provide a simple regret analysis of some TS algorithms for which the optimality is already known. We then further illustrate the interest of our approach, by analyzing a new Non-Parametric TS algorithm (h-NPTS), adapted to some families of unbounded reward distributions with a bounded h-moment. This model can for instance capture some non-parametric families of distributions whose variance is upper bounded by a known constant.
更多查看译文
关键词
algorithms,multi-armed
AI 理解论文
溯源树
样例
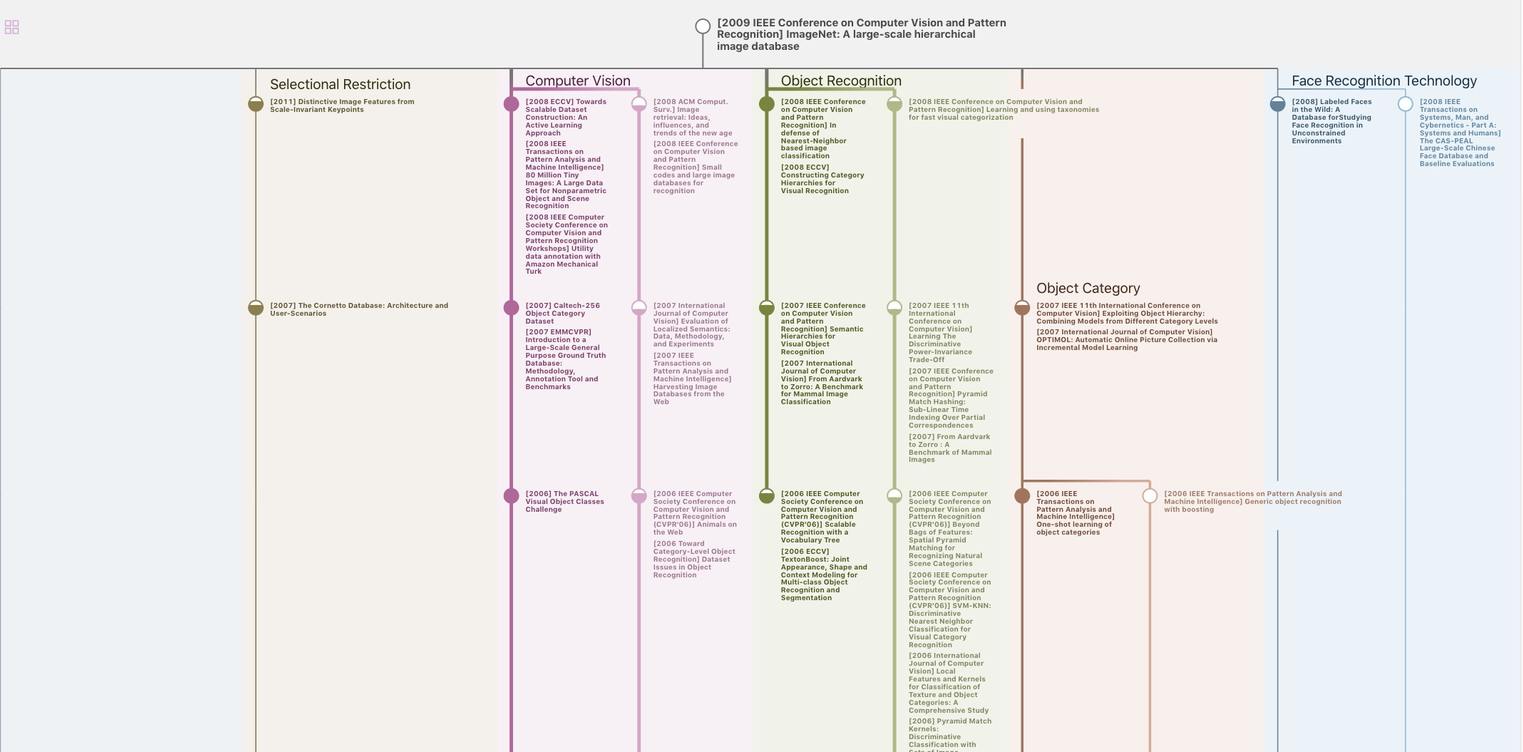
生成溯源树,研究论文发展脉络
Chat Paper
正在生成论文摘要