Deep Anomaly Detection on Tennessee Eastman Process Data
CHEMIE INGENIEUR TECHNIK(2023)
摘要
This paper provides the first comprehensive evaluation and analysis of modern (deep-learning) unsupervised anomaly detection methods for chemical process data. We focus on the Tennessee Eastman process dataset, which has been a standard litmus test to benchmark anomaly detection methods for nearly three decades. Our extensive study will facilitate choosing appropriate anomaly detection methods in industrial applications.
更多查看译文
关键词
anomaly detection
AI 理解论文
溯源树
样例
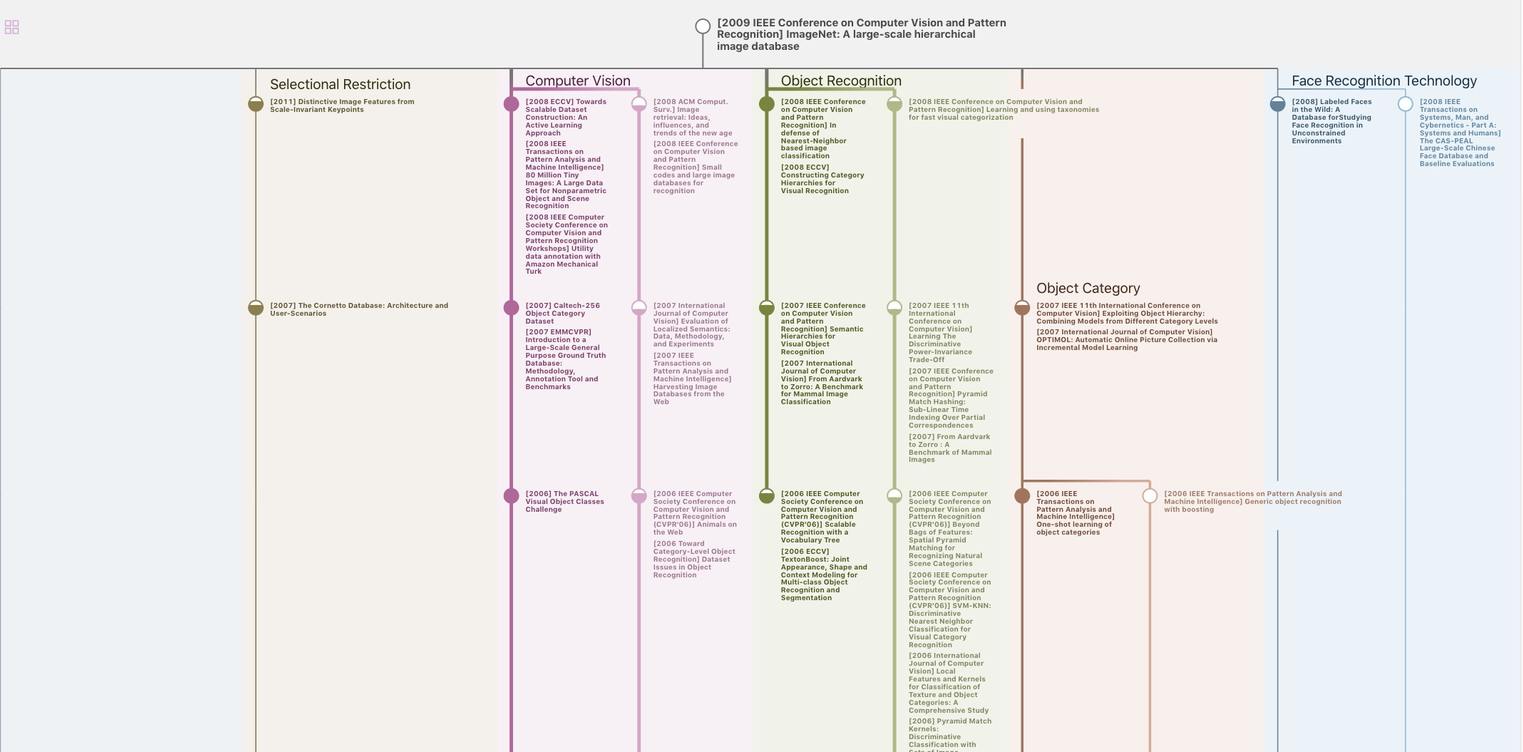
生成溯源树,研究论文发展脉络
Chat Paper
正在生成论文摘要