Class-imbalanced Unsupervised and Semi-Supervised Domain Adaptation for Histopathology Images
2023 45TH ANNUAL INTERNATIONAL CONFERENCE OF THE IEEE ENGINEERING IN MEDICINE & BIOLOGY SOCIETY, EMBC(2023)
摘要
In dealing with the lack of sufficient annotated data and in contrast to supervised learning, unsupervised, self-supervised, and semi-supervised domain adaptation methods are promising approaches, enabling us to transfer knowledge from rich labeled source domains to different (but related) unlabeled target domains, reducing distribution discrepancy between the source and target domains. However, most existing domain adaptation methods do not consider the imbalanced nature of the real-world data, affecting their performance in practice. We propose to overcome this limitation by proposing a novel domain adaptation approach that includes two modifications to the existing models. Firstly, we leverage the focal loss function in response to class-imbalanced labeled data in the source domain. Secondly, we introduce a novel co-training approach to involve pseudo-labeled target data points in the training process. Experiments show that the proposed model can be effective in transferring knowledge from source to target domain. As an example, we use the classification of prostate cancer images into low-cancerous and high-cancerous regions.
### Competing Interest Statement
The authors have declared no competing interest.
更多查看译文
关键词
Deep Learning,Histopathology,Whole Slide Images,Unsupervised Domain Adaptation,Semi-supervised Domain Adaptation,Class imbalance
AI 理解论文
溯源树
样例
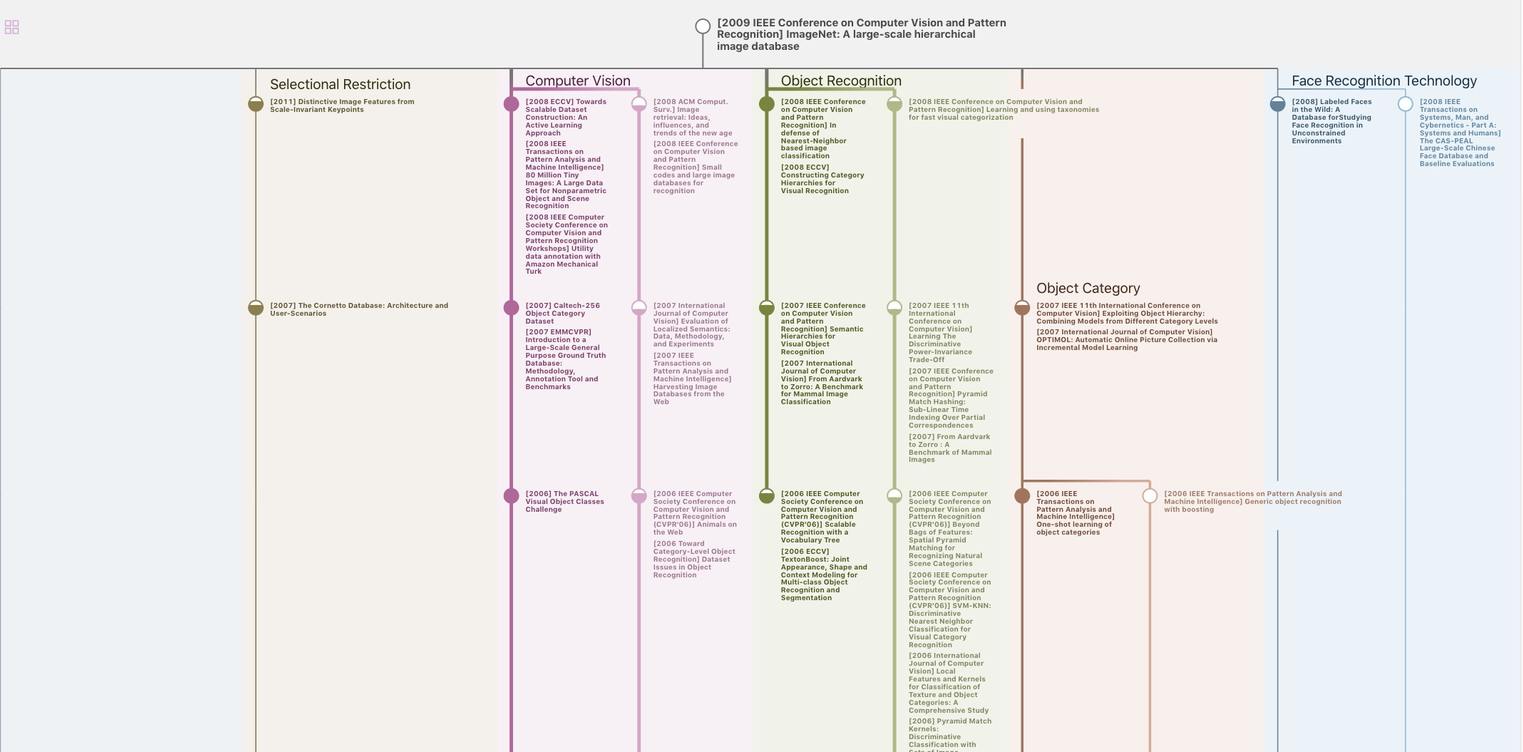
生成溯源树,研究论文发展脉络
Chat Paper
正在生成论文摘要