An Adaptive Real-Time Malicious Node Detection Framework Using Machine Learning in Vehicular Ad-Hoc Networks (VANETs).
Sensors (Basel, Switzerland)(2023)
摘要
Modern vehicle communication development is a continuous process in which cutting-edge security systems are required. Security is a main problem in the Vehicular Ad Hoc Network (VANET). Malicious node detection is one of the critical issues found in the VANET environment, with the ability to communicate and enhance the mechanism to enlarge the field. The vehicles are attacked by malicious nodes, especially DDoS attack detection. Several solutions are presented to overcome the issue, but none are solved in a real-time scenario using machine learning. During DDoS attacks, multiple vehicles are used in the attack as a flood on the targeted vehicle, so communication packets are not received, and replies to requests do not correspond in this regard. In this research, we selected the problem of malicious node detection and proposed a real-time malicious node detection system using machine learning. We proposed a distributed multi-layer classifier and evaluated the results using OMNET++ and SUMO with machine learning classification using GBT, LR, MLPC, RF, and SVM models. The group of normal vehicles and attacking vehicles dataset is considered to apply the proposed model. The simulation results effectively enhance the attack classification with an accuracy of 99%. Under LR and SVM, the system achieved 94 and 97%, respectively. The RF and GBT achieved better performance with 98% and 97% accuracy values, respectively. Since we have adopted Amazon Web Services, the network's performance has improved because training and testing time do not increase when we include more nodes in the network.
更多查看译文
关键词
DDoS,OMNET++,VANET,machine learning,real-time malicious nodes
AI 理解论文
溯源树
样例
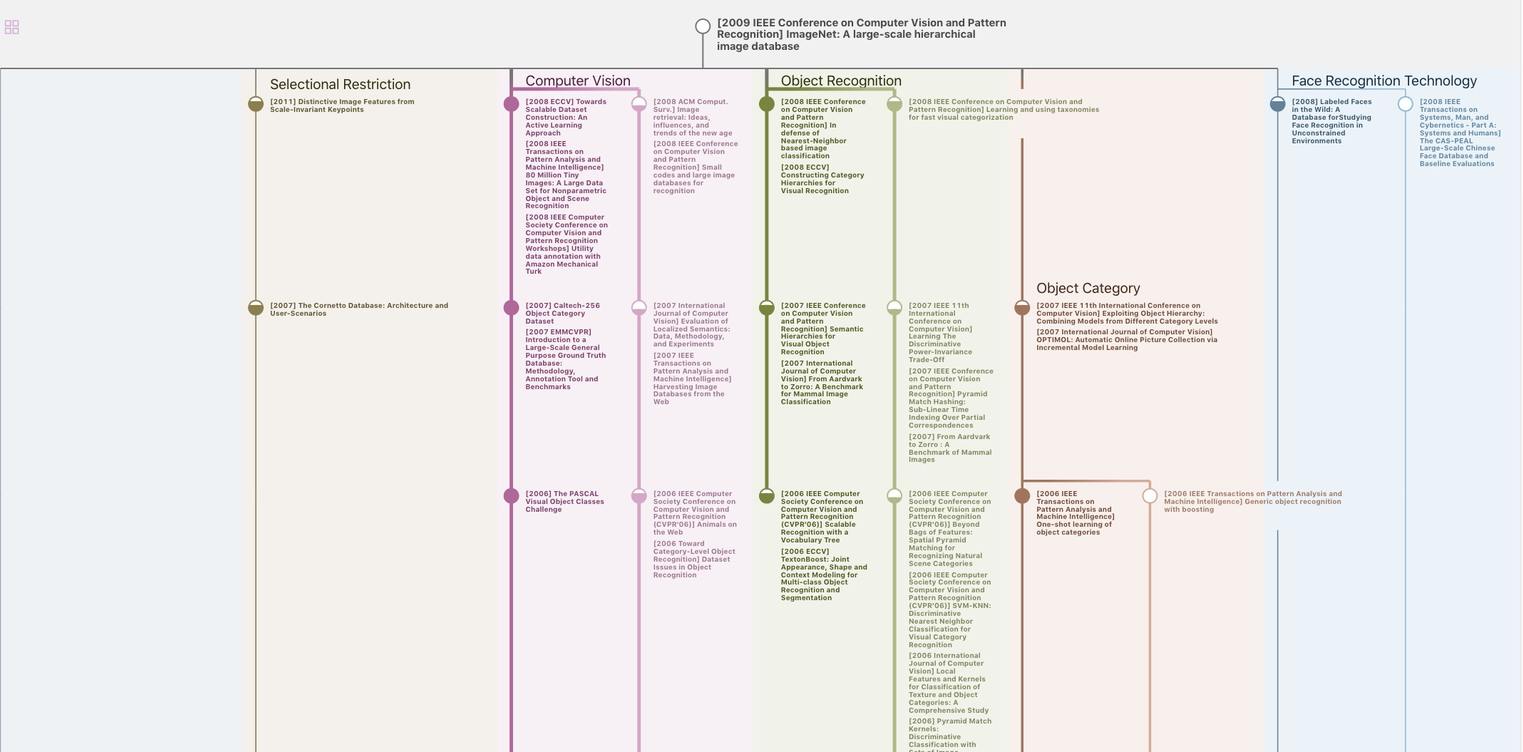
生成溯源树,研究论文发展脉络
Chat Paper
正在生成论文摘要