Machine Learning Prediction Model of Tuberculosis Incidence Based on Meteorological Factors and Air Pollutants.
International journal of environmental research and public health(2023)
Abstract
BACKGROUND:Tuberculosis (TB) is a public health problem worldwide, and the influence of meteorological and air pollutants on the incidence of tuberculosis have been attracting interest from researchers. It is of great importance to use machine learning to build a prediction model of tuberculosis incidence influenced by meteorological and air pollutants for timely and applicable measures of both prevention and control.
METHODS:The data of daily TB notifications, meteorological factors and air pollutants in Changde City, Hunan Province ranging from 2010 to 2021 were collected. Spearman rank correlation analysis was conducted to analyze the correlation between the daily TB notifications and the meteorological factors or air pollutants. Based on the correlation analysis results, machine learning methods, including support vector regression, random forest regression and a BP neural network model, were utilized to construct the incidence prediction model of tuberculosis. RMSE, MAE and MAPE were performed to evaluate the constructed model for selecting the best prediction model.
RESULTS:(1) From the year 2010 to 2021, the overall incidence of tuberculosis in Changde City showed a downward trend. (2) The daily TB notifications was positively correlated with average temperature (r = 0.231), maximum temperature (r = 0.194), minimum temperature (r = 0.165), sunshine duration (r = 0.329), PM2.5 (r = 0.097), PM10 (r = 0.215) and O3 (r = 0.084) (p < 0.05). However, there was a significant negative correlation between the daily TB notifications and mean air pressure (r = -0.119), precipitation (r = -0.063), relative humidity (r = -0.084), CO (r = -0.038) and SO2 (r = -0.034) (p < 0.05). (3) The random forest regression model had the best fitting effect, while the BP neural network model exhibited the best prediction. (4) The validation set of the BP neural network model, including average daily temperature, sunshine hours and PM10, showed the lowest root mean square error, mean absolute error and mean absolute percentage error, followed by support vector regression.
CONCLUSIONS:The prediction trend of the BP neural network model, including average daily temperature, sunshine hours and PM10, successfully mimics the actual incidence, and the peak incidence highly coincides with the actual aggregation time, with a high accuracy and a minimum error. Taken together, these data suggest that the BP neural network model can predict the incidence trend of tuberculosis in Changde City.
MoreTranslated text
Key words
tuberculosis,machine learning,forecasting,neural networks,random forest regression,support vector regression
AI Read Science
Must-Reading Tree
Example
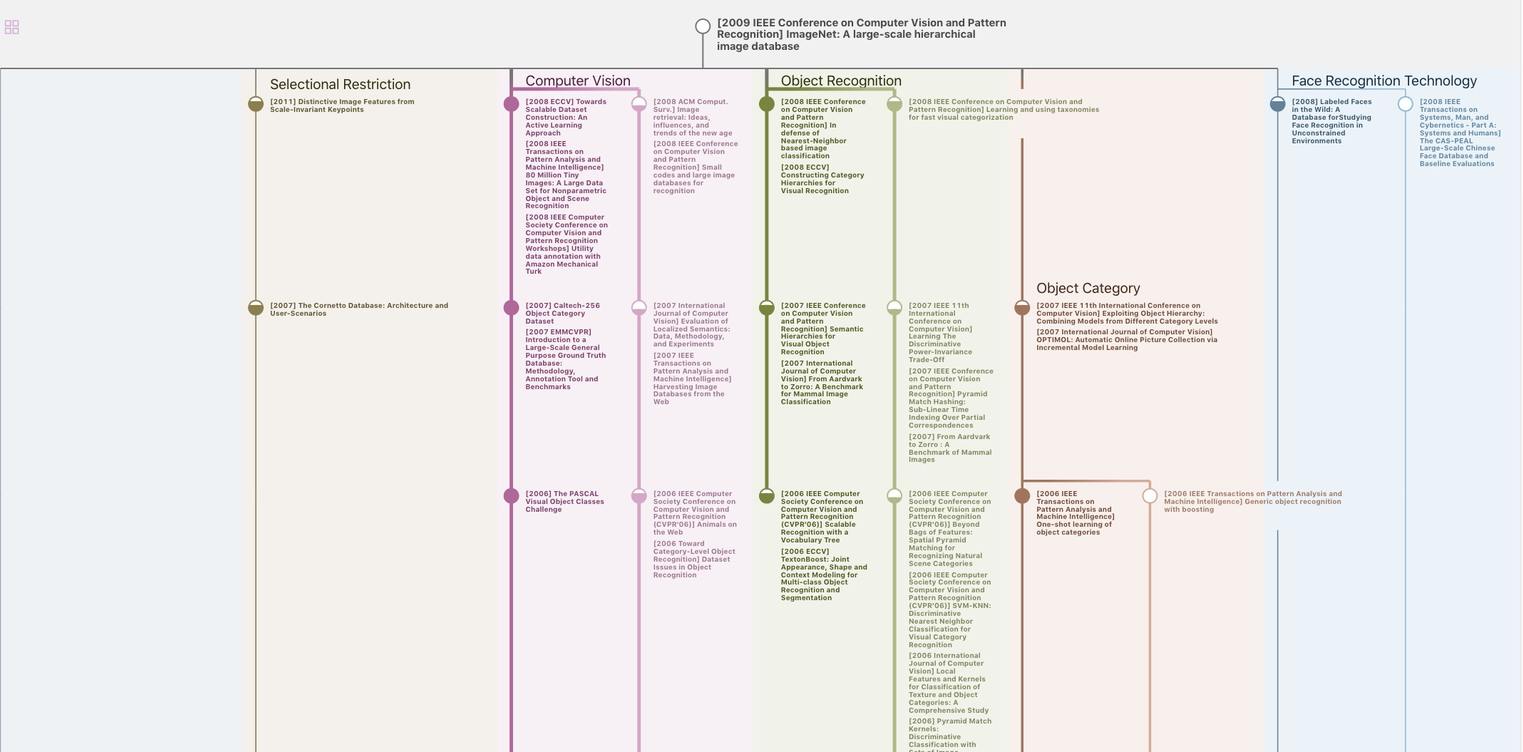
Generate MRT to find the research sequence of this paper
Chat Paper
Summary is being generated by the instructions you defined