Towards Data Gravity and Compliance Aware Distributed Deep Learning on Hybrid Clouds
2022 IEEE 29th International Conference on High Performance Computing, Data and Analytics Workshop (HiPCW)(2022)
摘要
To store large volumes of data concurrently from a diverse set of sources, data stores such as data silos, lakes, and warehouses, have been widely embraced by various organizations. Thanks to data fabric architectures, such scattered data (both structurally and geographically), can be accessed transparently at scale while adhering to various administrative regulations (e.g. governance, privacy, compliance, etc.). However, modern workload schedulers and distributed deep learning (DDL) runtimes are oblivious to the uneven data distribution across different storage services and compliance regulations, leading to sub-optimal resource utilization and training completion times. Al-though state-of-art workflow schedulers such as Apache Hadoop Yarn, Horovod, etc. exploit data locality, they require application developers to explicitly map data and resources available across various cloud services during job submission. These approaches are redundant and counterproductive for next-generation data fabric architectures that feature automated transparency and compliance abstractions for accessing disparate data sources with uneven data distribution. To this end, we propose an algorithm based on greedy programming that leverages the meta-data catalog of data fabric to efficiently determine training schedules based on data gravity, compliance, and resource availability. Our simulations based on synthetic data and resource distribution profiles demonstrate significant improvements in execution times and resource utilization compared to traditional DDL scheduling approaches in hybrid multi-cloud environments.
更多查看译文
关键词
Data Fabric,Heterogeneous Data Distribution,Workflow Scheduling,Data-intensive Workloads
AI 理解论文
溯源树
样例
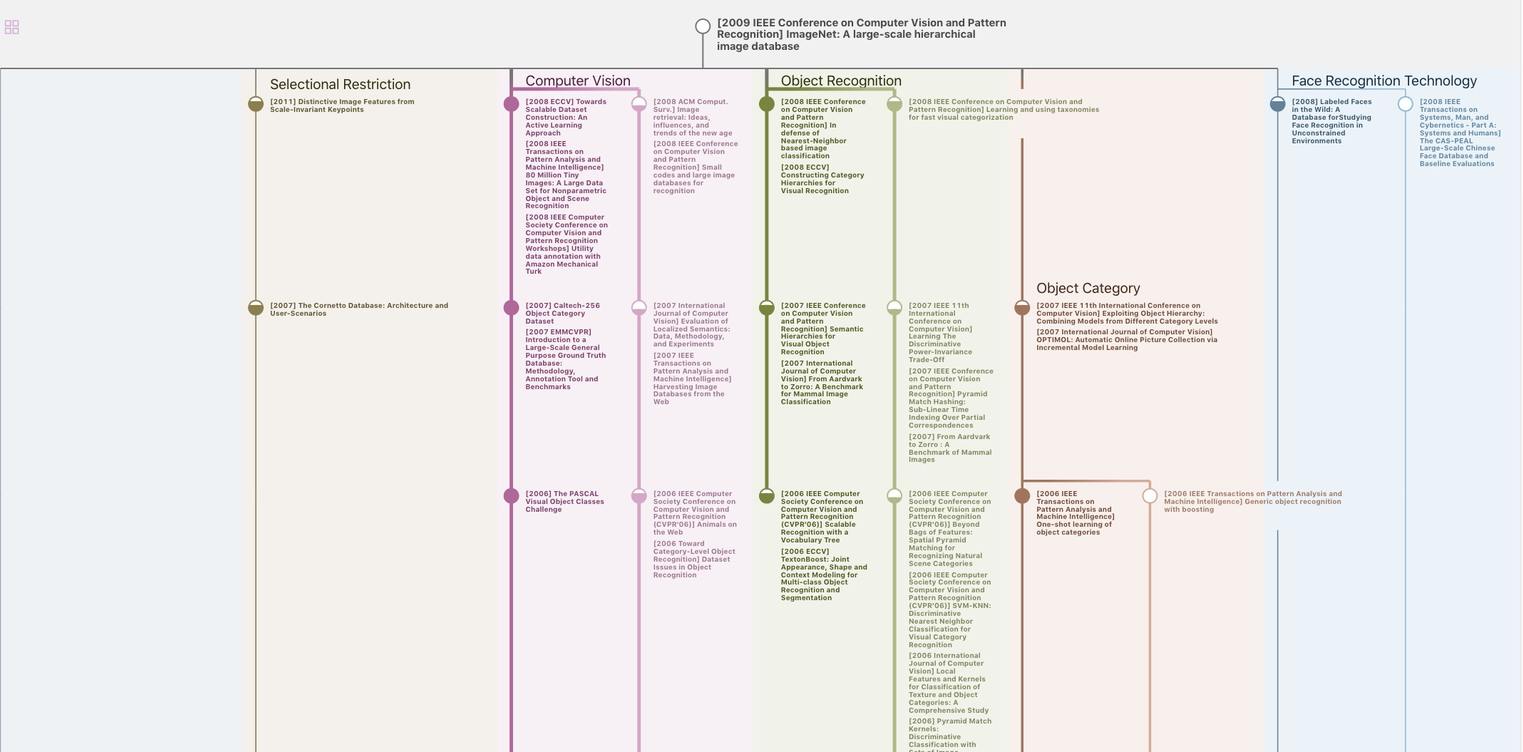
生成溯源树,研究论文发展脉络
Chat Paper
正在生成论文摘要