Estimating Near-Surface Concentrations of Major Air Pollutants From Space: A Universal Estimation Framework LAPSO
IEEE Transactions on Geoscience and Remote Sensing(2023)
摘要
Like many other countries, China is still facing severe air pollution issues after extensive efforts. The difficulties in deriving near-surface concentrations from satellite measurements restrict the application of remote sensing of large-scale surface air quality. Aiming at providing daily accurate near-surface ail pollution estimates (PM2.5, PM10, O3, NO2, SO2, and CO), we propose a robust estimation framework called learning air pollutants from satellite observations (LAPSO). The principle of LAPSO is to derive a nonlinear relationship between surface pollutant concentrations of interest and satellite observations with the aid of meteorological reanalyzes based on deep learning techniques. The LAPSO framework is superior to other algorithms due to its robust retrieval performance, independence from chemical transport models (CTMs), lower hardware requirements, and a user-friendly interface. The retrieval results of LAPSO were in good agreement with ground-level measurements according to extensive cross-validation at 1628 sites (
$\text{R}^{2}>$
0.8 in polluted areas and uncertainty
$\ll 5~\mu \text{g}/\text{m}^{3}$
for most pollutants) in China. The framework also showed a strong capability to capture the temporal variability of different air pollutants. By comparing with the estimation results from different satellite platforms, TROPOspheric monitoring instrument (TROPOMI) onboard the Sentinel-5P demonstrated marginally better performance for estimating PM2.5. Although the selection of satellite observations did not significantly affect the results of O3 estimation, the number and spatial sampling density of in situ sites imposed large impacts on O3 estimation performance. The success of LAPSO for estimating near-surface concentrations from satellite remote sensing at an enhanced spatiotemporal resolution is expected to serve the continuous and dynamical monitoring of regional and global air pollution.
更多查看译文
关键词
Air pollution,deep learning,remote sensing
AI 理解论文
溯源树
样例
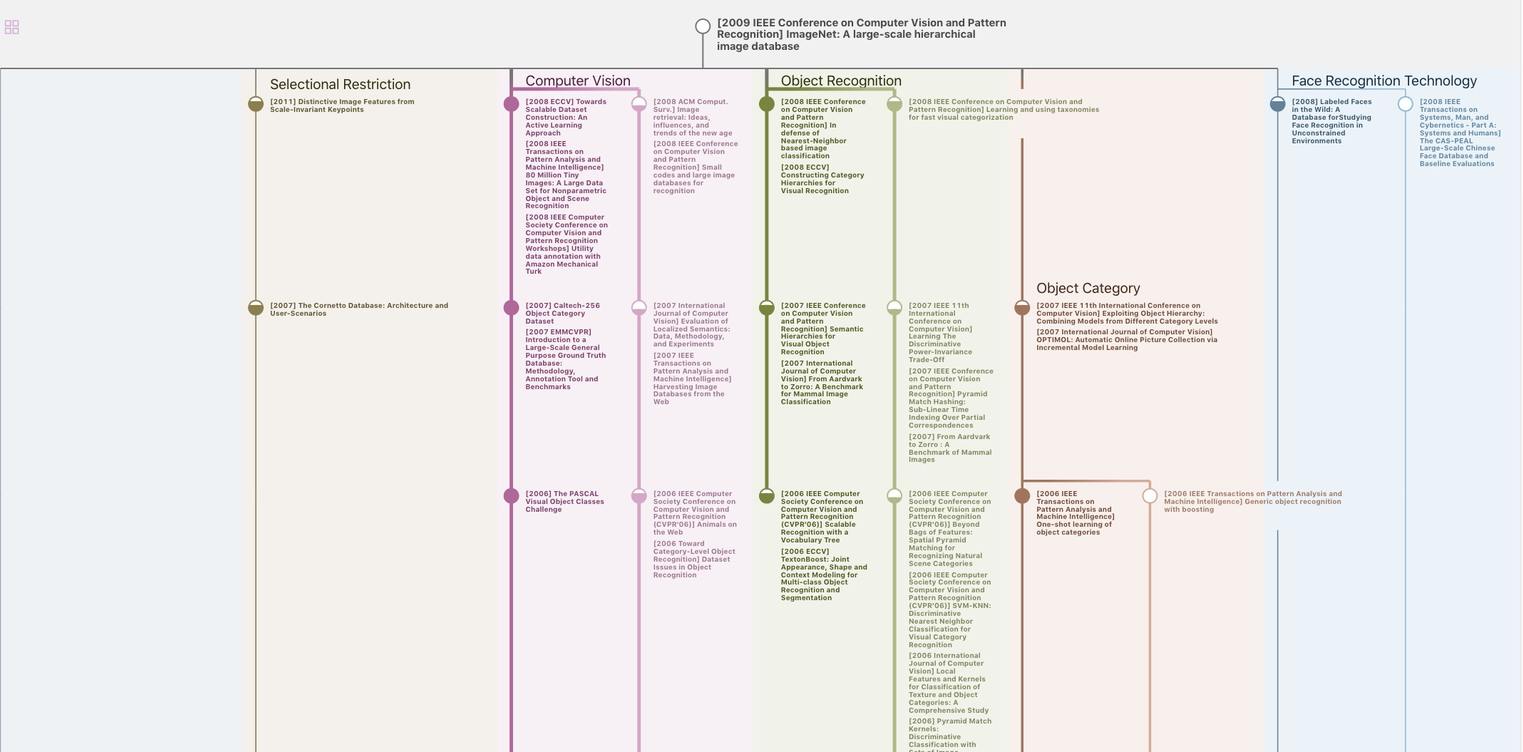
生成溯源树,研究论文发展脉络
Chat Paper
正在生成论文摘要