Deep Reinforcement Learning for Reactive Content Caching With Predicted Content Popularity in Three-Tier Wireless Networks
IEEE Transactions on Network and Service Management(2023)
摘要
With the explosive growth of micro-video applications, the mobile traffic generated by retrieving a few user-generated micro-videos has brought a massive burden to backhaul links and backbone networks. Unlike other types of videos, user-generated micro-videos are typically requested by a large number of users within an extremely short period after their release. Therefore, it is crucial to predict the content popularity and make caching decision for the newly requested content timely. To predict content popularity in different locations, the request probabilities of the content in different locations are translated into rating scores. Then, a recommendation system-based prediction model is designed to predict rating scores of the newly requested content in different locations. To increase caching diversity and realize vertically collaboration in a three-tier wireless network, a deep reinforcement learning-based reactive content caching strategy is proposed to make caching decision for the newly requested content. The main goal is to obtain a higher caching gain at a lower caching space cost. To evaluate the caching performance, a new metric, cache benefit rate, is defined as the download latency reduction brought by each bit of cache. Compared with matrix factorization, the performance of prediction model can be increased by 23.98% and 12.44% in terms of mean absolute error and root mean square error, respectively. Extensive simulations demonstrate that the proposed reactive caching strategy outperforms other caching strategies under different system parameters in terms of the cache hit rate, average download time, and cache benefit rate.
更多查看译文
关键词
Content popularity prediction,convolutional neural network,deep reinforcement learning,reactive caching
AI 理解论文
溯源树
样例
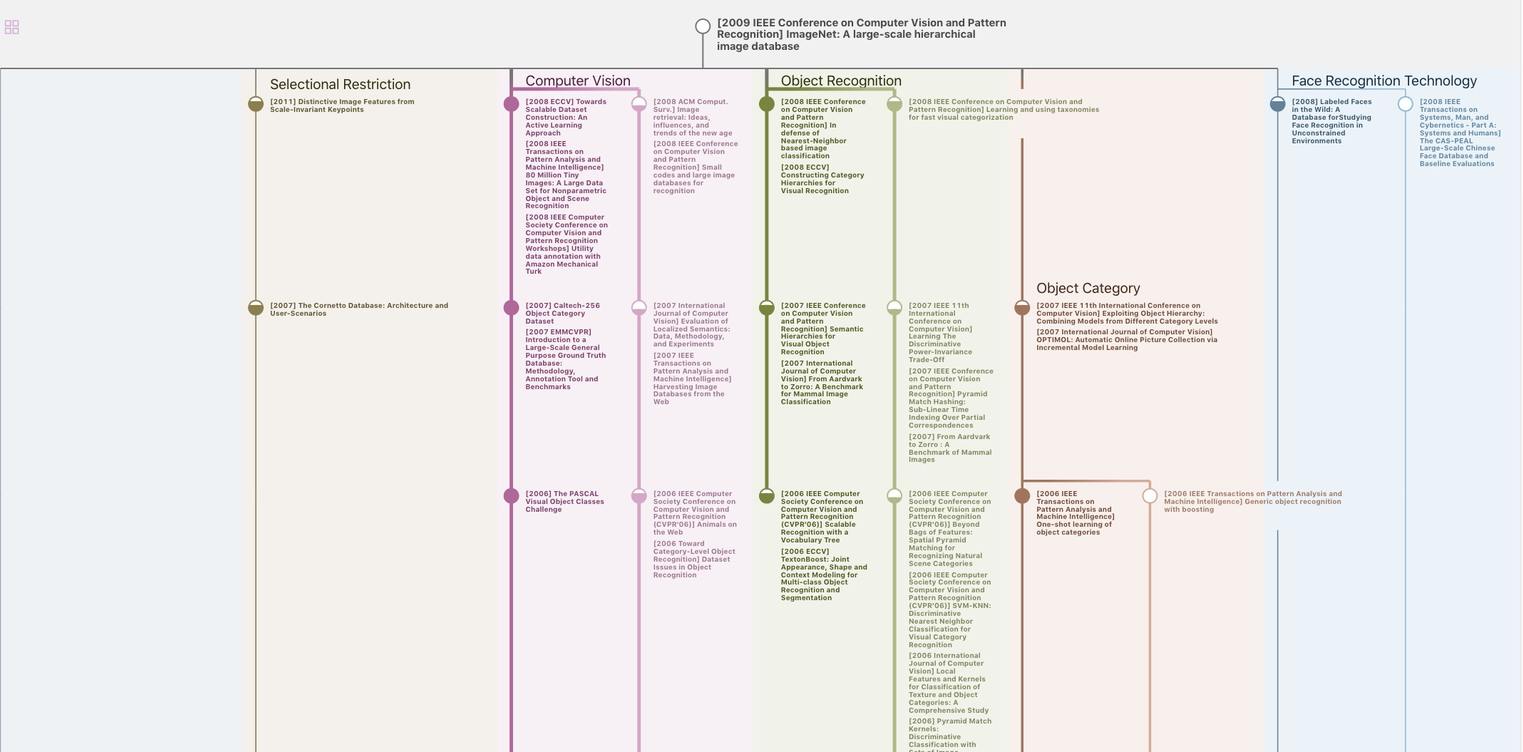
生成溯源树,研究论文发展脉络
Chat Paper
正在生成论文摘要