Reliability and Validity of Transcranial Magnetic Stimulation-Electroencephalography Biomarkers.
Biological psychiatry. Cognitive neuroscience and neuroimaging(2022)
摘要
Noninvasive brain stimulation and neuroimaging have revolutionized human neuroscience with a multitude of applications, including diagnostic subtyping, treatment optimization, and relapse prediction. It is therefore particularly relevant to identify robust and clinically valuable brain biomarkers linking symptoms to their underlying neural mechanisms. Brain biomarkers must be reproducible (i.e., have internal reliability) across similar experiments within a laboratory and be generalizable (i.e., have external reliability) across experimental setups, laboratories, brain regions, and disease states. However, reliability (internal and external) is not alone sufficient; biomarkers also must have validity. Validity describes closeness to a true measure of the underlying neural signal or disease state. We propose that these metrics, reliability and validity, should be evaluated and optimized before any biomarker is used to inform treatment decisions. Here, we discuss these metrics with respect to causal brain connectivity biomarkers from coupling transcranial magnetic stimulation (TMS) with electroencephalography (EEG). We discuss controversies around TMS-EEG stemming from the multiple large off-target components (noise) and relatively weak genuine brain responses (signal), as is unfortunately often the case in noninvasive human neuroscience. We review the current state of TMS-EEG recordings, which consist of a mix of reliable noise and unreliable signal. We describe methods for evaluating TMS-EEG biomarkers, including how to assess internal and external reliability across facilities, cognitive states, brain networks, and disorders and how to validate these biomarkers using invasive neural recordings or treatment response. We provide recommendations to increase reliability and validity, discuss lessons learned, and suggest future directions for the field.
更多查看译文
AI 理解论文
溯源树
样例
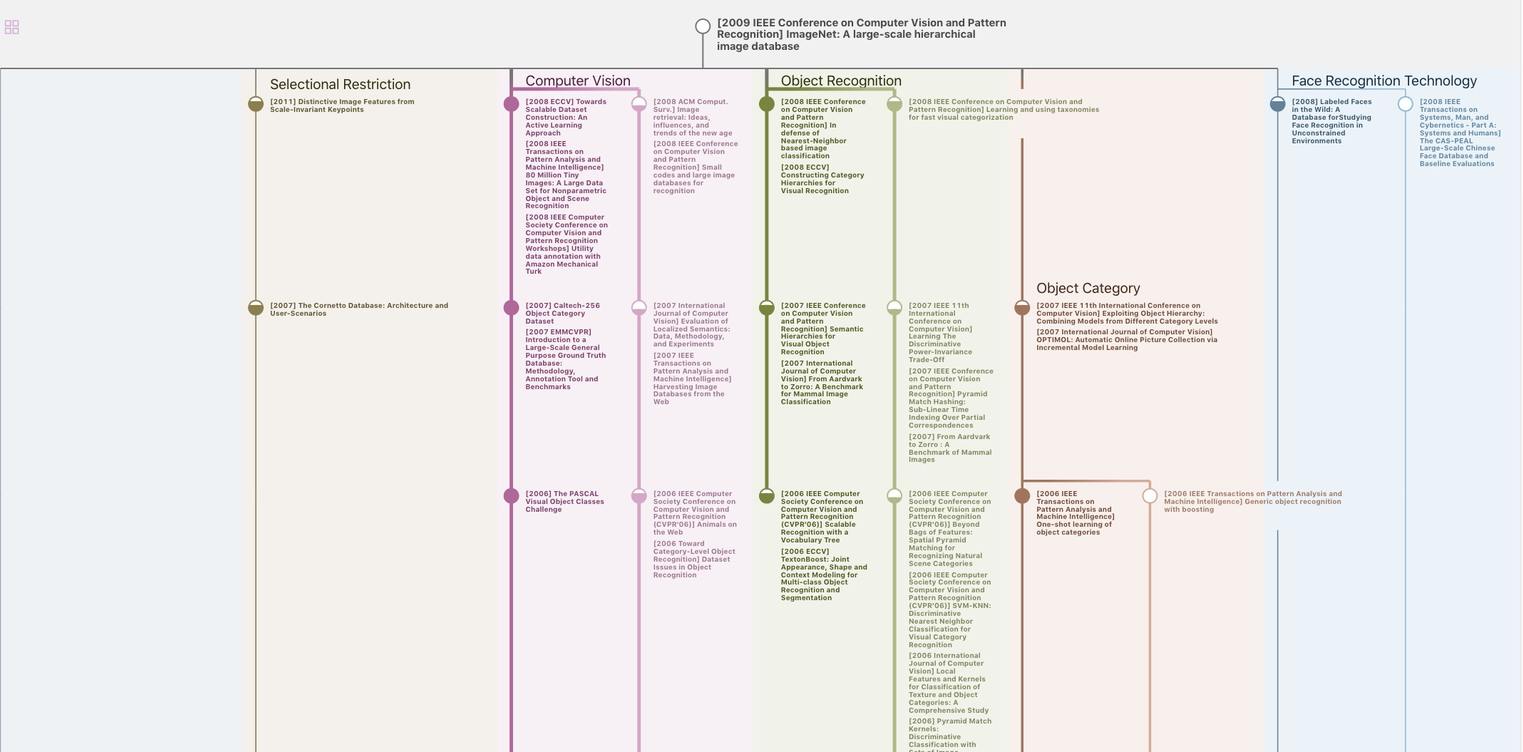
生成溯源树,研究论文发展脉络
Chat Paper
正在生成论文摘要