Pure graph-guided multi-view subspace clustering
Pattern Recognition(2023)
摘要
Multi-view subspace clustering approaches have shown outstanding performance in revealing similarity relationships and complex structures hidden in data. Despite the progress, previous multi-view clustering methods still face two challenges: (1) it is difficult to simultaneously achieve sparsity and connectivity of the affinity graph; (2) existing methods usually separate the graph learning step from the clustering process, which leads to unsatisfactory clustering performance as the final results critically rely on the learned graph. In this paper, we propose to achieve a structured consensus graph for multi-view subspace clustering by leveraging the sparsity and connectivity of each affinity graph. In the proposed method, the pure graph for each view is searched by finding the good neighbors. The multiple pure graphs are further fused into a consensus graph with a block-diagonal structure. That is, the consensus graph is enforced to contain exactly c connected components where c is the number of the clusters. Hence the label to each sample can be directly assigned since each connected component precisely corresponds to an individual cluster. As a result, the proposed model seamlessly accomplishes the subtasks including graph construction, pure graph learning (i.e., good neighbors searching), and cluster label allocation in a mutual reinforcement manner. Extensive experimental results demonstrate the superiority and reliability of our proposed method. (c) 2022 Elsevier Ltd. All rights reserved.
更多查看译文
关键词
Multi -view learning,Subspace clustering,Graph learning,Pure graph
AI 理解论文
溯源树
样例
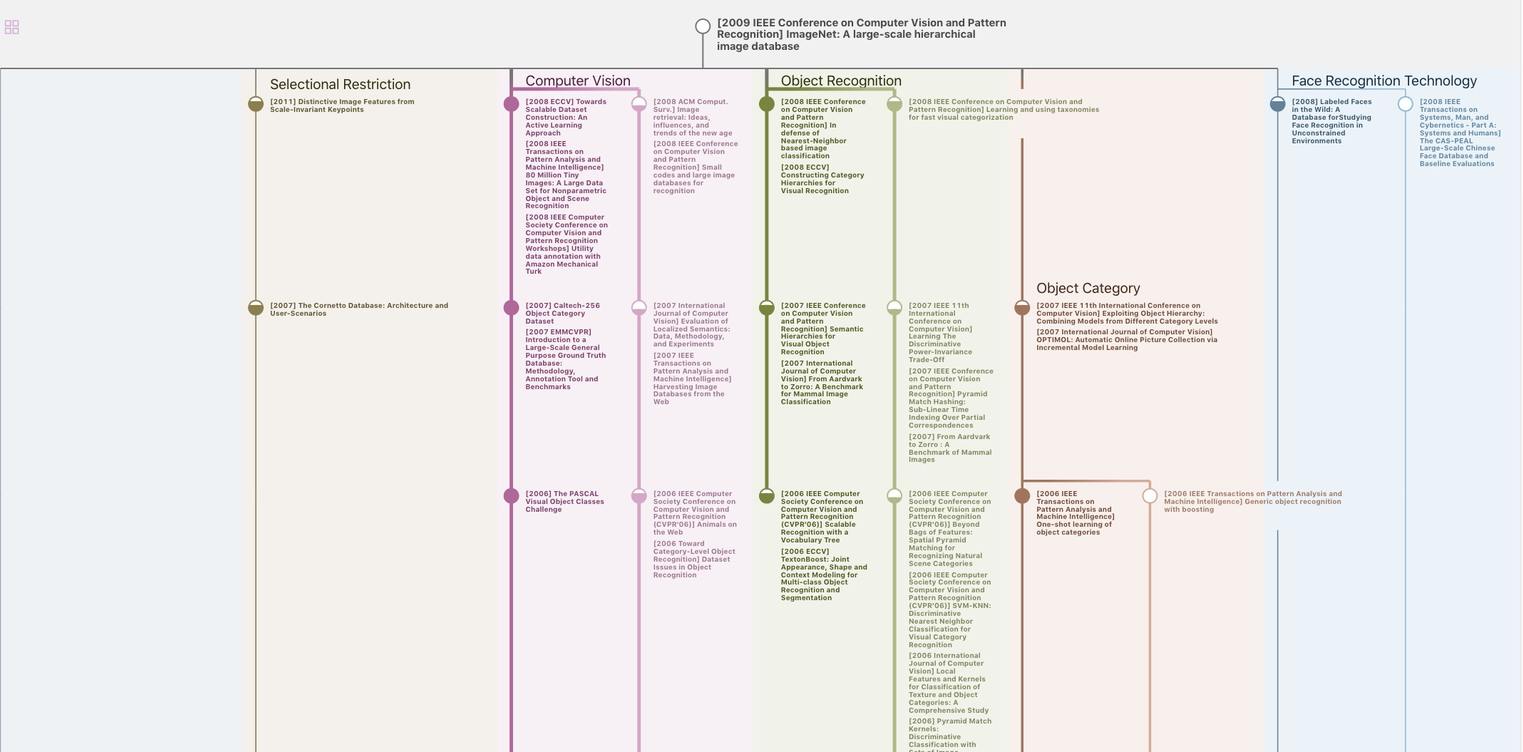
生成溯源树,研究论文发展脉络
Chat Paper
正在生成论文摘要