A nonconvex $$\hbox{TV}_q-l_1$$ TV q - l 1 regularization model and the ADMM based algorithm
Scientific Reports(2022)
摘要
The total variation (TV) regularization with $$l_1$$ fidelity is a popular method to restore the image contaminated by salt and pepper noise, but it often suffers from limited performance in edge-preserving. To solve this problem, we propose a nonconvex $$\hbox{TV}_q-l_1$$ regularization model in this paper, which utilizes a nonconvex $$l_q$$ -norm $$(0<1)$$ defined in total variation (TV) domain (called $$\hbox{TV}_q$$ regularizer) to regularize the restoration, and uses $$l_1$$ fidelity to measure the noise. Compared to the traditional TV model, the proposed model can more effectively preserve edges and contours since it provides a more sparse representation of the restoration in TV domain. An alternating direction method of multipliers (ADMM) combining with majorization-minimization (MM) scheme and proximity operator is introduced to numerically solve the proposed model. In particular, a sufficient condition for the convergence of the proposed algorithm is provided. Numerical results validate the proposed model and algorithm, which can effectively remove salt and pepper noise while preserving image edges and contours. In addition, compared with several state-of-the-art variational regularization models, the proposed model shows the best performance in terms of peak signal to noise ratio (PSNR) and mean structural similarity index (MSSIM). We can obtain about 0.5 dB PSNR and 0.06 MSSIM improvements against all compared models.
更多查看译文
关键词
Applied mathematics,Computational science,Computer science,Information technology,Software,Science,Humanities and Social Sciences,multidisciplinary
AI 理解论文
溯源树
样例
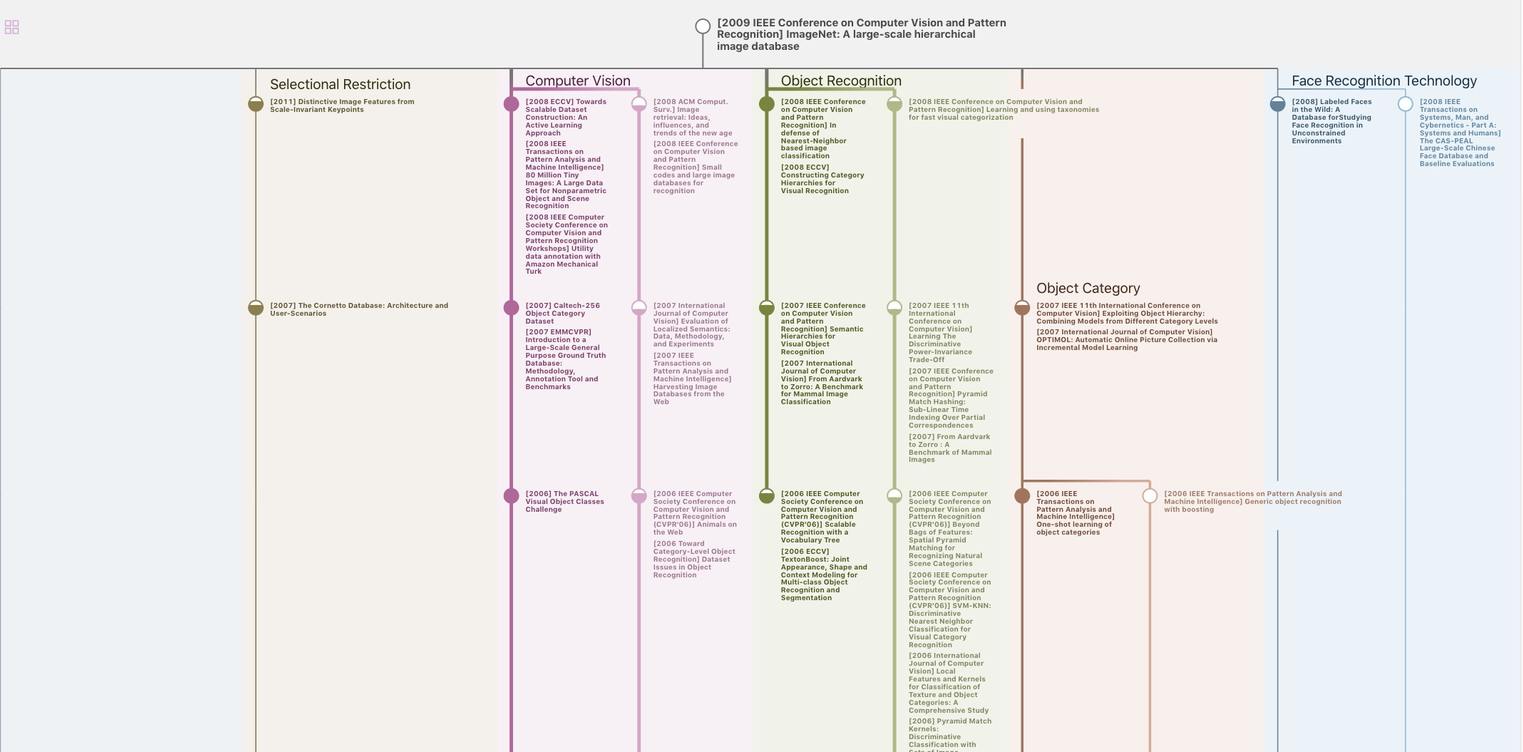
生成溯源树,研究论文发展脉络
Chat Paper
正在生成论文摘要