Learning Stationary Markov Processes with Contrastive Adjustment
CoRR(2023)
Abstract
We introduce a new optimization algorithm, termed contrastive adjustment, for learning Markov transition kernels whose stationary distribution matches the data distribution. Contrastive adjustment is not restricted to a particular family of transition distributions and can be used to model data in both continuous and discrete state spaces. Inspired by recent work on noise-annealed sampling, we propose a particular transition operator, the noise kernel, that can trade mixing speed for sample fidelity. We show that contrastive adjustment is highly valuable in human-computer design processes, as the stationarity of the learned Markov chain enables local exploration of the data manifold and makes it possible to iteratively refine outputs by human feedback. We compare the performance of noise kernels trained with contrastive adjustment to current state-of-the-art generative models and demonstrate promising results on a variety of image synthesis tasks.
MoreTranslated text
Key words
stationary markov processes,learning,adjustment
AI Read Science
Must-Reading Tree
Example
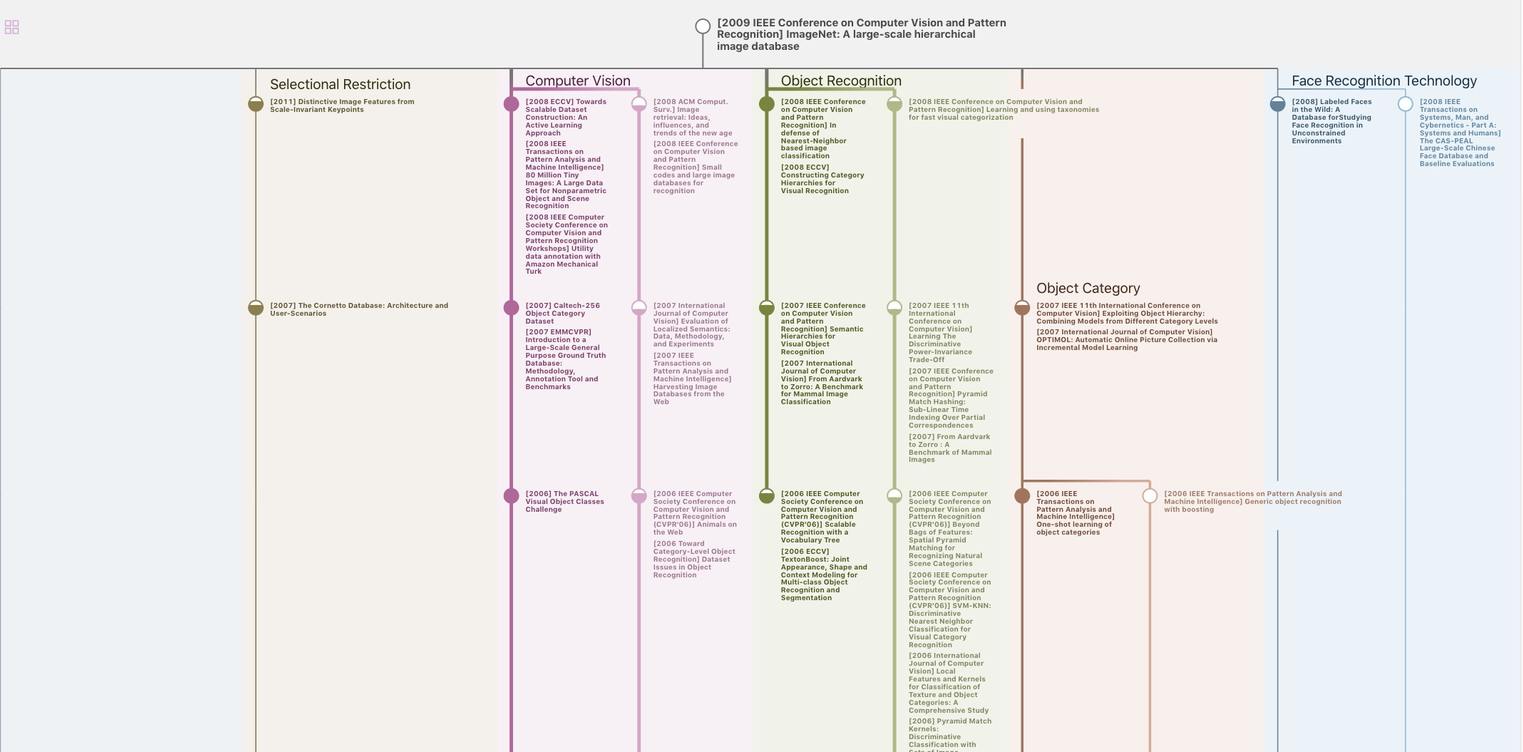
Generate MRT to find the research sequence of this paper
Chat Paper
Summary is being generated by the instructions you defined