FPGA-based DNN Hardware Accelerator for Sensor Network Aggregation Node
2022 56th Asilomar Conference on Signals, Systems, and Computers(2022)
摘要
With the rapid development of various wireless sensors and Internet-of-things devices, a massive amount of time-varying data is generated and continuously streamed to the cloud. Given the transmission latency and cost, real-time or near real-time data analytics using the conventional cloud-centric approach are challenging. Hierarchical computing models have been introduced to reduce the amount of data uploaded to the cloud and enable timely data analysis at different network end-points. Edge computing enables analytics to be physically close to the data source at sensing and aggregation nodes. The limited computational resources on the sensor nodes and aggregation nodes motivate the development of lightweight, energy-efficient algorithms. This work proposes an FPGA-based energy-efficient deep neural network (DNN) architecture for sensor network aggregation nodes. The proposed scheme builds on our previous work, focusing on local outliers detection and data reduction at the sensing nodes. The new system adopts dual prediction (DP), outlier detection, and data compression (DC) techniques to exploit the spatiotemporal characteristic of the monitored area using data from multisite sensing nodes. Through experiments on an FPGA-based testbed, we demonstrate the proposed system's ability to recover the original sensor node observations with minimal accuracy loss using less than 30 % of the actual sensor observations. The
$106\mu s$
average latency and 0.104W power consumption make the proposed architecture suitable for resource-constrained computing platforms.
更多查看译文
关键词
WSNs,FPGA,DNN,Autoencoder,LSTM,Dual prediction,Data Compression,Outliers Detection
AI 理解论文
溯源树
样例
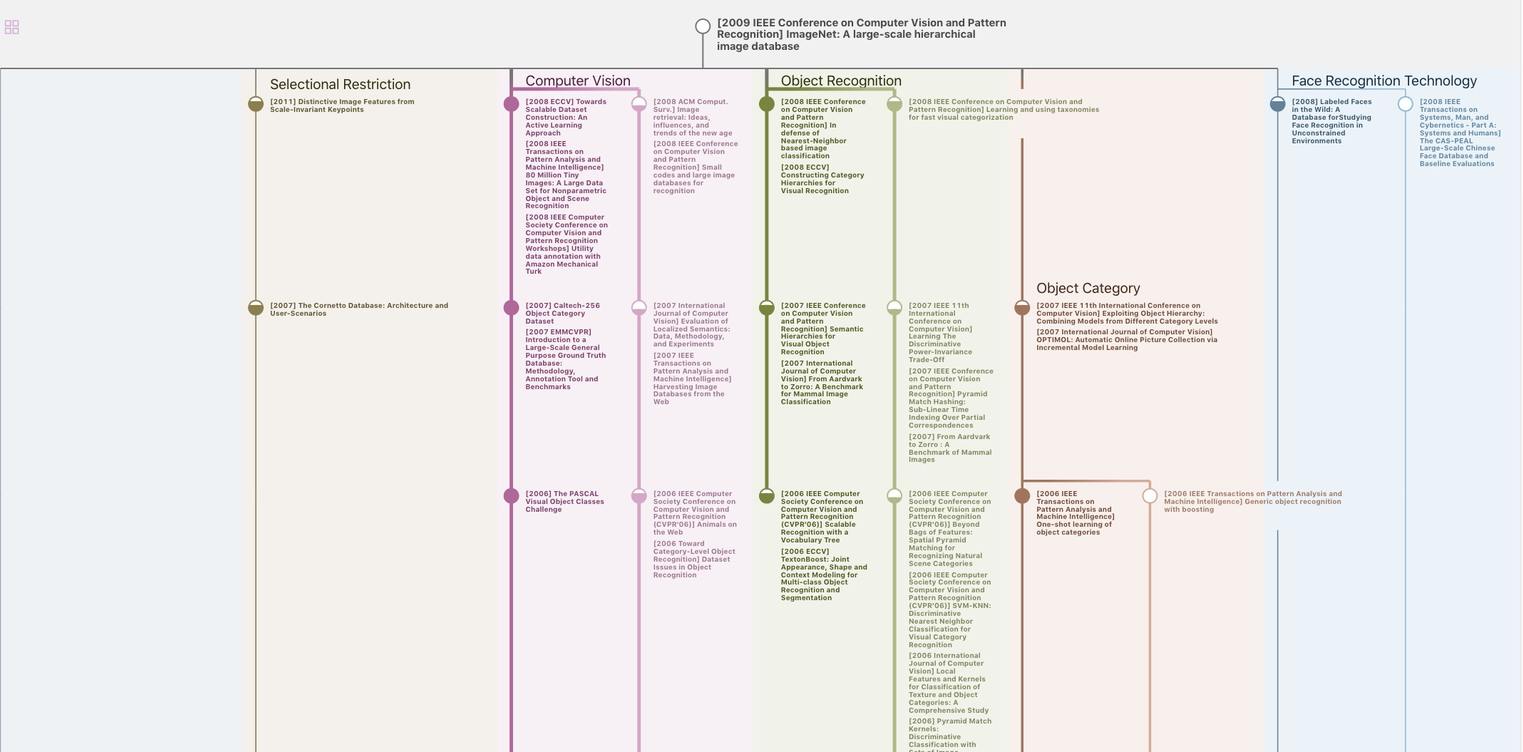
生成溯源树,研究论文发展脉络
Chat Paper
正在生成论文摘要