Prediction of New-Onset Diabetes After Pancreatectomy With Subspace Clustering Based Multi-View Feature Selection
IEEE Journal of Biomedical and Health Informatics(2023)
摘要
The pancreas plays an important role in glucose metabolism, and developing diabetes or long-term glucose metabolism disturbance may be a prevalent sequela after pancreatectomy. Nevertheless, relative factors of new-onset diabetes after pancreatectomy stay unclear. Radiomics analysis is potential to identify image markers for disease prediction or prognosis. Meanwhile, combination of imaging and electronic medical record (EMR) showed superior performance than imaging or EMR alone in previous studies. One critical step is to identity predictors from high-dimensional features, and it is even more challenging to select and fuse imaging and EMR features. In this work, we develop a radiomics pipeline to assess postoperative new-onset diabetes risk of patients undergoing distal pancreatectomy. Specifically, we extract multiscale image features with 3D wavelet transformation, and include patients’ characteristics, body composition and pancreas volume information as clinical features. Then, we propose a multi-view subspace clustering guided feature selection method (MSCUFS) for the selection and fusion of image and clinical features. Finally, a prediction model is constructed with classical machine learning classifier. Experimental results on an established distal pancreatectomy cohort showed that the SVM model with combined imaging and EMR features demonstrated good discrimination, with an AUC value of 0.824, which improved the model with image features alone by 0.037 AUC. Compared with state-of-the-art feature selection methods, the proposed MSCUFS has superior performance in fusing image and clinical features.
更多查看译文
关键词
Diabetes prediction,multi-view feature selection,subspace clustering,distal pancreatectomy
AI 理解论文
溯源树
样例
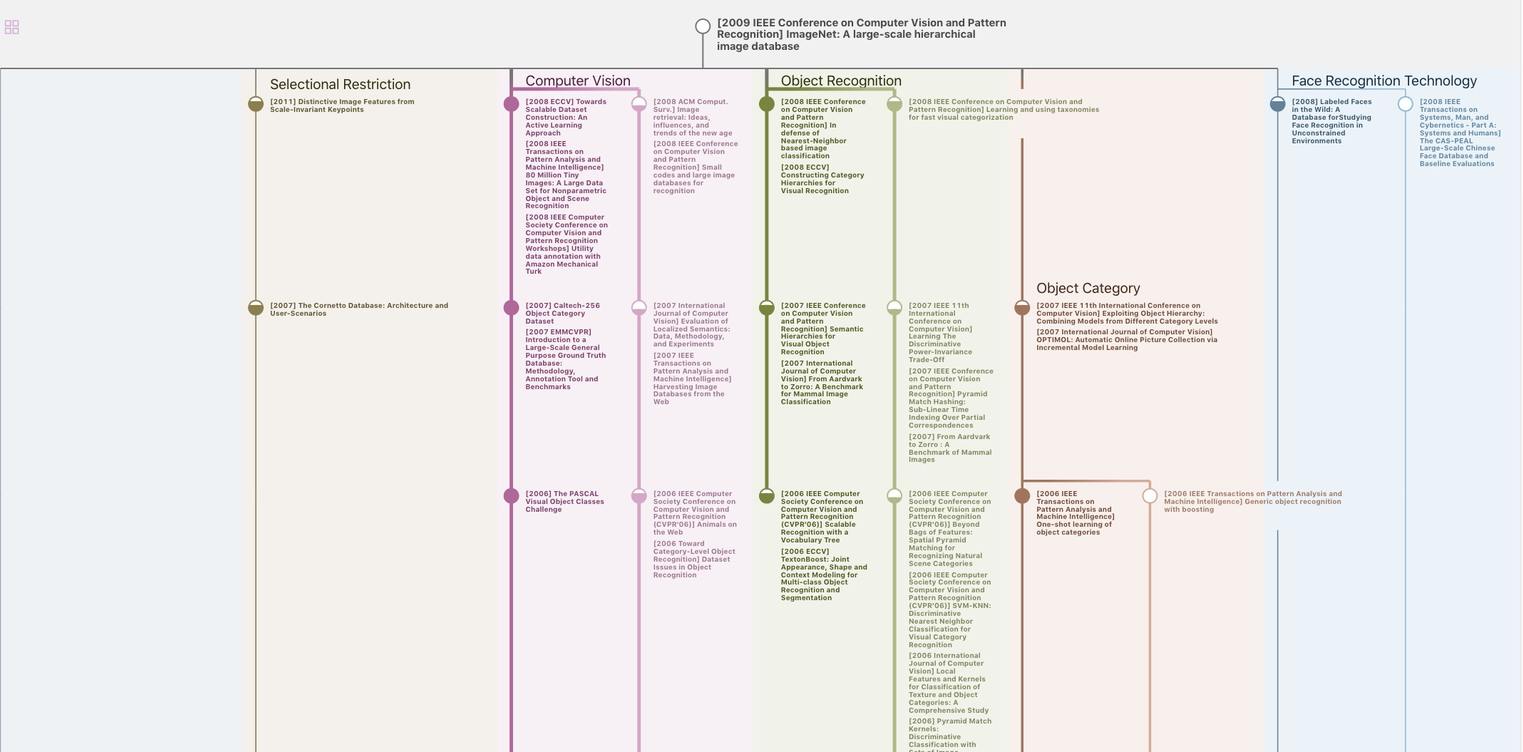
生成溯源树,研究论文发展脉络
Chat Paper
正在生成论文摘要