Everywhere: A Framework for Ubiquitous Indoor Localization
IEEE Internet of Things Journal(2023)
摘要
Smartphones have become an integral part of daily human life and enable almost unlimited coverage of human mobility. Thus, collecting pervasive crowdsourced signatures is feasible. Autonomous localization of such signatures promotes the development of a self-deployable and ubiquitous indoor positioning system (IPS). However, previous crowdsourcing-based IPSs have not considered leveraging such data for developing ubiquitous IPSs. They have relied on methods for data selection and sources for localization adjustment that could work against realizing a ubiquitous system. In contrast, this study introduces a framework Everywhere that leverages crowdsourced data to develop a ubiquitous IPS and addresses existing challenges while developing such systems. Particularly, inertial data selection criteria are proposed to autonomously generate traces with better localization. Moreover, pervasive global navigation satellite system (GNSS) data are leveraged to adjust trace localization, while simultaneously introducing a deploying location (inside elevators) of one anchor node. The node surveys all the floors while reducing the localization error, especially for the buildings surrounded by GNSS-denied areas. Additionally, cumulative data densification is leveraged to realize pervasive resources within the building, thereby boosting trace adjustment and extending database spatial coverage. Furthermore, a better selection of neighboring fingerprints is proposed to enhance online fingerprinting. Such a framework can promote a ubiquitous IPS development for buildings regardless of whether they are surrounded by open sky or GNSS-denied areas.
更多查看译文
关键词
Crowdsourcing,fingerprinting,indoor localization,Internet of Things (IoT),location-based service (LBS),ubiquitous localization
AI 理解论文
溯源树
样例
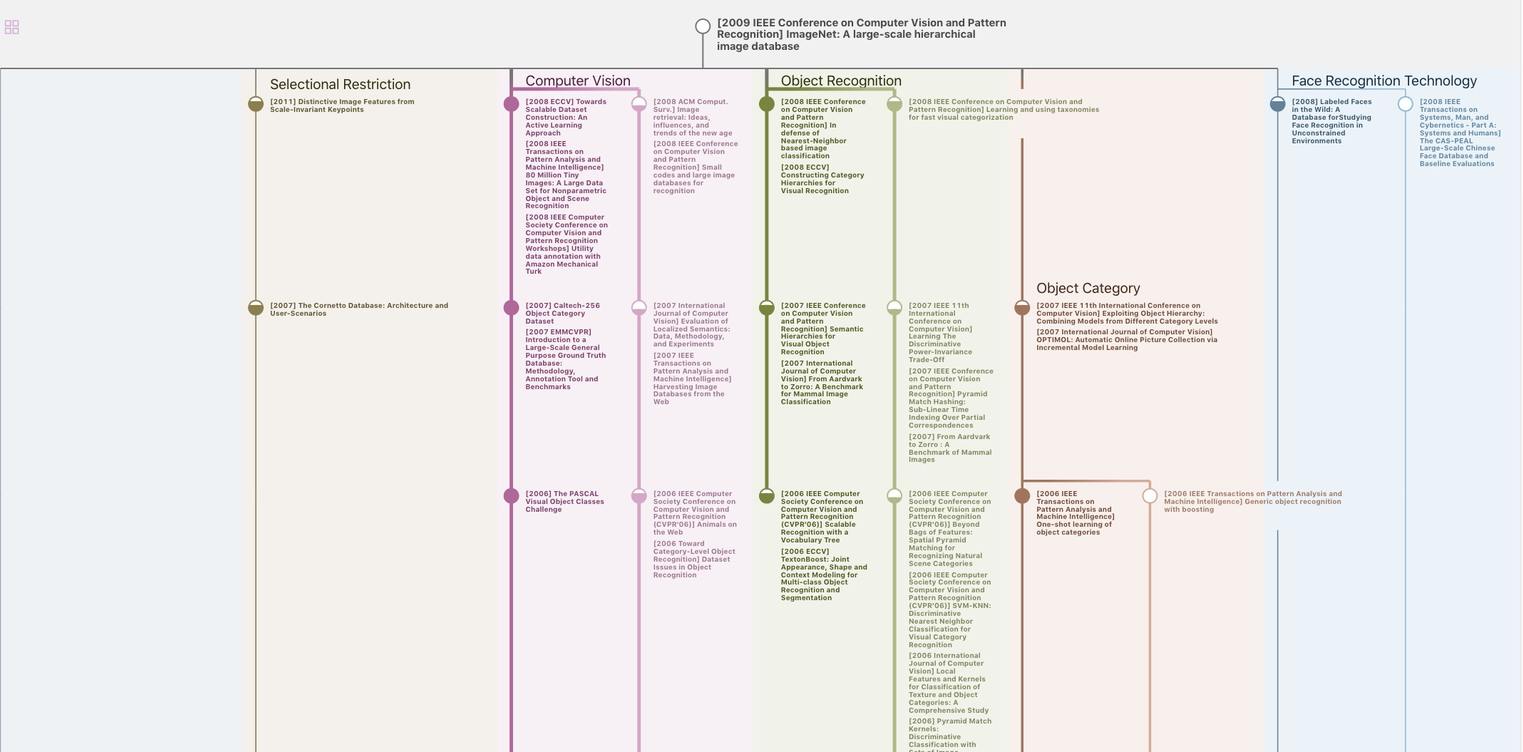
生成溯源树,研究论文发展脉络
Chat Paper
正在生成论文摘要