Radial Basis Neural Networks for Class Discovery
2022 OITS International Conference on Information Technology (OCIT)(2022)
摘要
The non-iterative structure of radial basis function neural networks makes them more appealing for classification tasks decade after decade. In line with the growth of the training data set, the pattern layer also grows. The unprecedented growth of data with no class labels is becoming a challenge for researchers who are working in data science, big data analysis, etc. Although there is literature witnessed for many algorithms to handle data with no class label. However, neural network-based algorithms with a special characteristic of radial basis neural networks for uncovering class labels are very rare. In this paper, we propose a novel class discovery algorithm that combines the best features of radial basis function neural networks (RBFN) and self-organizing feature map (SOFM). We have taken a few datasets with class label for our experimental work. In the training phase of the network, the training instances are used without class labels. In the test phase, they are validated by combining the predicted class labels with their actual class label. The result shows that the proposed algorithm can be treated as an alternative method for class discovery.
更多查看译文
关键词
Radial basis function neural networks,Class discovery,Self-organizing feature map,Unsupervised learning
AI 理解论文
溯源树
样例
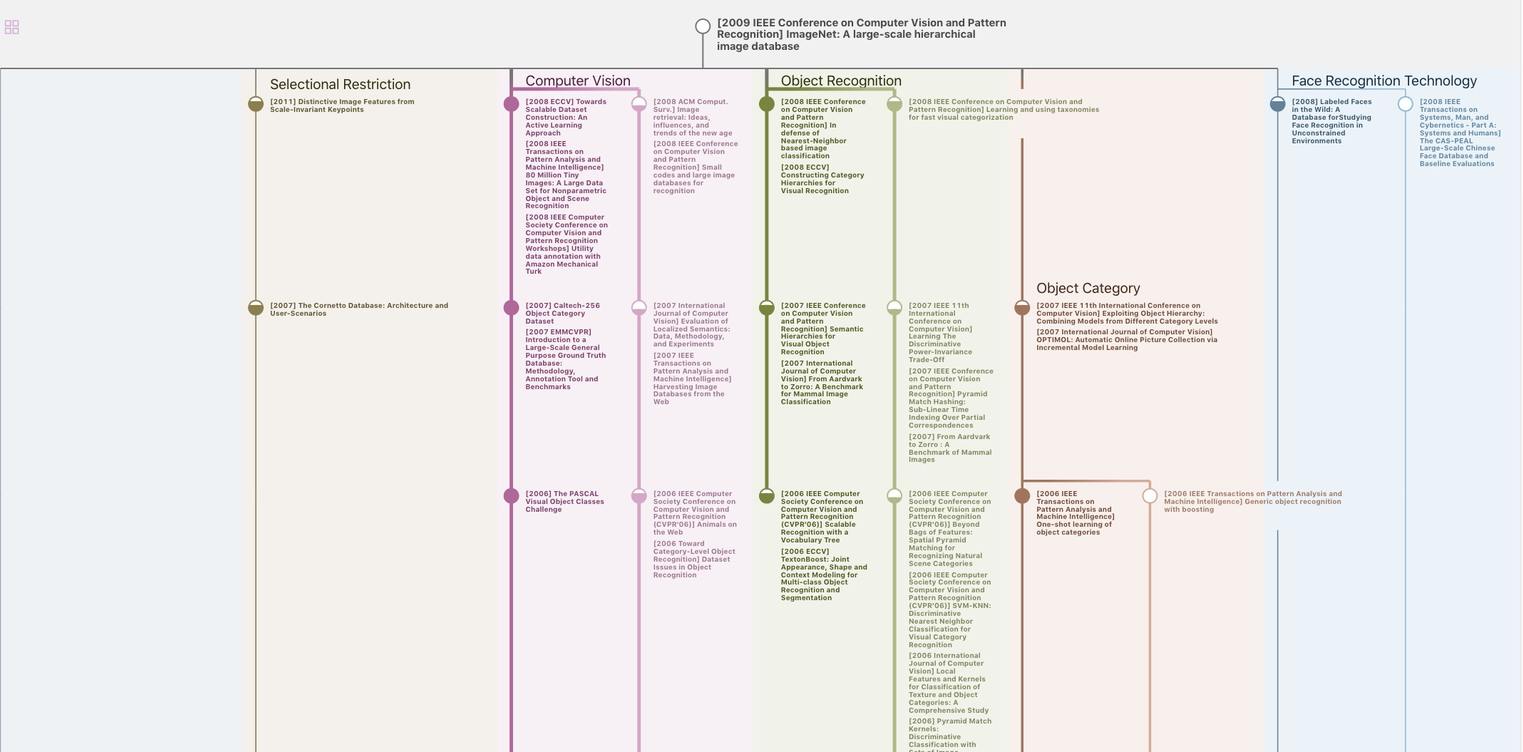
生成溯源树,研究论文发展脉络
Chat Paper
正在生成论文摘要